RDP 2024-04: Nowcasting Quarterly GDP Growth during the COVID-19 Crisis Using a Monthly Activity Indicator Non-technical summary for ‘Nowcasting Quarterly GDP Growth during the COVID-19 Crisis Using a Monthly Activity Indicator’
July 2024
What did we set out to do?
It is said that the future is uncertain – but so is the present. Policymakers need to know what's happening in the economy right now but often the information they have is outdated or provided with a lag. This need for timely information was most acute during the COVID-19 crisis when conditions were rapidly evolving, forcing policymakers to make decisions under significant uncertainty. Policymakers are further hamstrung because the most detailed measure of economy activity – gross domestic product (GDP) – is published with a long lag, limiting its value as a measure of the current state of the economy.
We develop a monthly activity indicator (MAI) for Australia to help close this information gap. We did this by identifying the common signal from 30 timely indicators of economic activity. We then tested the MAI to determine how accurate it is.
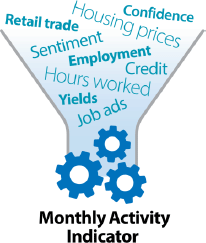
What did we learn?
Our results show that:
- The MAI can predict quarterly GDP growth with reasonable accuracy months before the official release.
- The MAI ‘nowcasts’ – that is, its predictions of the present – significantly outperform standard benchmarks that rely only on quarterly data.
- This outperformance was largest during the COVID-19 crisis, emphasising the benefit of exploiting more timely monthly data at times of high uncertainty.
What was our key takeaway?
The MAI is an accurate and useful tool for monitoring economic activity and can provide policymakers in Australia with a more immediate snapshot of current economic conditions than existing models.