RDP 9307: Explaining Forward Discount Bias: Is it Anchoring? 5. Empirical Results
June 1993
- Download the Paper 119KB
5.1 Calibrating the Model
We derive empirical results for the special case of the model and hence the exchange rate evolves according to equation (11).[20] We require values for five parameters: θ, λ, σm, σe and β. Estimates for the first four come from real-world data. 1/θ is the rate at which goods-prices and the interest differential adjust to money supply shocks and is estimated from the time-series properties of 3-month interest differentials between pairs of the countries US, UK, (West) Germany and Japan. Details of the interest rates used are in the Data Appendix. The AR(1) equation describing the evolution of (i – i*)t (equation (6)) is estimated by OLS leading to the estimates for θ shown in Table 1 (p 36).[21] To cover the range of results in Table 1, we generate numerical results with θ = 6 periods and θ = 50 periods.[22]
Estimates of the semi-elasticity of money demand with respect to the interest rate, λ, are derived from money demand functions estimated for the G-7 by Fair (1987). Details are presented in Appendix D and the resulting parameter values are shown in Table 2. When θ = 6 periods (50 periods), the derived value of λ implies that a 1% increase in the nominal money supply leads to contemporaneous fall in the domestic interest rate of 2.0% p.a. (0.7% p.a.).
The standard deviation of nominal money shocks, σm, is set so that the standard deviation of the interest differential,
σ(i–i*), equals 1.9% p.a. (the standard deviation of
the Japan – Germany interest differential from Table 1, chosen because
these two countries had comparable inflation rates over the 1980s). The AR(1)
specification for (i–i*)t
implies that
, which allows σm to
be determined for given θ and λ. Again, the results are shown
in Table 2.
The standard deviation of long-run real exchange rate shocks, σe, is set so that the model generates an appropriate amount of exchange rate volatility. Baille and Bollerslev (1989) examine the stochastic properties of the nominal exchange rates of France, Italy, Japan, Switzerland, UK and (West) Germany against the US dollar, 1980–85. For 4-week periods, these exchange rates are well modelled by the process
where bj and σΔsj are country-specific constants and σΔsj ranges from 0.029 to 0.037 with a mean over the six exchange rates of 0.034. Choosing σe = 0.031 for the model ensures that model values of σΔs always fall within the range found by Baille and Bollerslev.[23]
We present results for two values of β. With the goods market in long-run equilibrium
(pt =
t), the impact response
to a money shock νt+1 is Δst+1
= 0 with only anchored traders in the market and Δst+1
= νt+1(1+θ/λ)
with only rational traders. We use values for β derived from the market's
impact response to this shock when managed wealth is divided equally between
the traders. When α = 1/2, if the spot exchange rate immediately jumps
halfway to the value it would take were the market fully rational (Δst+1
= νt+1(1+θ/λ)/2),
then from equation (11),
and we describe the anchored traders as ‘weakly anchored’. Alternatively, when α = 1/2, if the spot exchange rate immediately jumps only one-tenth of the way to the value it would take were the market fully rational, Δst+1 = νt+1(1 + θ/λ)/10, then
and we describe the anchored traders as ‘strongly anchored’.
5.2 Endogenous Determination of the Proportion of Anchored Traders
With a proportion α of market wealth managed by anchored traders, define Rk (α,n) as the gross real return trader k earns over n periods on the portfolio she manages. Further, define Z(α,n) ≡ Rα(α,n) – Rr (α,n) as the excess real return earned by anchored traders over n periods. Then, as demonstrated in Appendix E,
where .
For given α, we use (15) to derive estimates of p(α,n) ≡ Pr[Z(α,n) > 0], the probability that the anchored traders' chosen portfolio outperforms the rational traders' portfolio over the assumed horizon of investors, n. From these estimates, we also derive estimates of the proportion of anchored traders in the market in the long-run. We assume that investors follow a simple decision rule. Every n periods, they compare the relative performance of the two types of traders over the past n periods. When type-k traders (k = a or r) had the better performance, investors increase the proportion of wealth entrusted to them by a constant q, subject to the constraint that the share of market wealth entrusted to either type of trader never falls below zero.[24] Then the evolution of α follows a Markov chain
where the subscript T refers to successive n-period time-intervals.[25]
We can now derive the long-run stationary probability distribution of α. We assume α0 = 0 and q ∈ {0.1,0.3,0.5,1.0}. Then there are at most eleven states for the Markov chain, αi = i/10, i = 0,..., 10, and at all times, αT must take one of the values αi. The stationary probability distribution for α is defined by the probabilities pi
From this probability distribution, we derive the long-run average proportion of
anchored traders in the foreign exchange market, , given by
and the long-run average coefficient on the forward discount in equation (11),
,
5.3 Results and Discussion
Figure 3 shows empirical estimates of p(α,n) for one set of parameter values
(θ = 6 periods; strongly anchored traders) and for three assumed horizons
for investors (one, three and ten
years).[26]
Table 3 reports long-run averages of the proportion of anchored traders in the market,
, and the coefficient
on the forward discount in the standard test of forward discount bias,
, derived for different assumed values
of the Markov chain parameter, q, in equation (16).
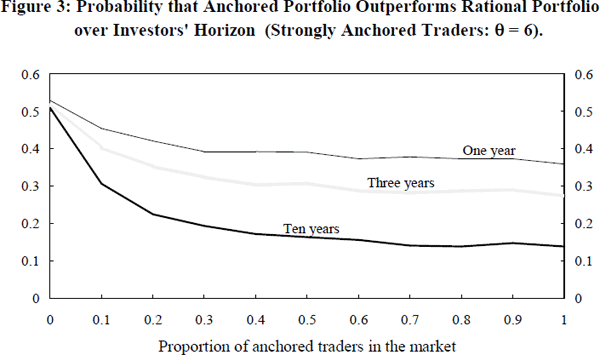
For all sets of parameter values, p(0,n) ≈ 0.5 and p(α,n) falls monotonically as α increases.[27] But it is noteworthy that even over long horizons, the chance that the anchored traders' portfolio outperforms the rational traders portfolio is not so small. An example makes the point. With θ = 6 and market wealth divided equally between rational and strongly anchored traders, η takes the value η ≈ −0.9. Thus, the forward discount is a very significantly biased estimate of the future exchange rate change. Despite this, over a three year (ten year) horizon, the portfolio chosen by the anchored traders (who use the forward discount as the anchor for their expectations of the exchange rate change) gives a higher return than the rational portfolio 31% (16%) of the time. This example highlights the general point that unpredictable exchange rate movements are so big that only a small advantage is imparted to those (rational) traders who understand the true relationship between interest rates and the exchange rate.
The results in Table 3 imply, over a wide range of parameter values, that a significant
proportion of anchored traders survive in the long-run and that they induce
substantial bias in the forward discount. The largest value of
in the Table is
= 0.63 while for strongly anchored
traders and shorter investor horizons, the value of
is close to zero or negative. As reported
in Section 2,
the empirically estimated coefficient on the forward discount in the corresponding
regression (equation (1)) is often negative. For the range of parameter values
investigated, while the model does not often generate negative values of
, it always generates strong forward
discount bias in the direction consistently observed empirically.
While one might quibble with the specification chosen for the anchored traders' expectations, a strong message emerges from these empirical results. If rational agents in the foreign exchange market are risk-averse or liquidity-constrained, the rational expectations solution for the exchange rate (allowing for risk-aversion) is a fragile one. Unpredictable exchange rate movements are so big that there are no strong grounds for presuming that agents with quite different expectations will be driven from the market. This may help explain why economists have had such difficulty modelling the short-run behaviour of exchange rates.
5.4 Comparison with Other Work
We begin this sub-section by showing that the model generates several of the empirical
regularities described in the literature. McCallum (1992) presents a useful
summary of these regularities with eight OLS regressions using over twelve
years of monthly data for $/DM, $/£ and $/¥ exchange rates. For the first seven regressions, Table 4 compares
his $/DM results (which are representative) with results derived from a simulation
of the model. The first regression in the table is the standard test for forward
discount bias and, while the model produces a negative coefficient on the forward
discount, it is significantly less negative than McCallum's coefficient.
As discussed earlier, for the range of parameter values investigated, the model
does not generate large negative values of this coefficient ().
We now explain the model results for the remaining regressions in the table. The model results for regressions (2) and (3) are a consequence of the fact that s and f are co-integrated I(1) variables with co-integrating vector, st = ft. This explains both the estimated slope coefficient and the very high regression R2. Further, in the model,
Since et is much larger than all the other terms on the right-hand-side of either of these equations, a regression of Δst on Δft is rather like regressing et on itself, which explains the model results for regression (4). Lagging the right-hand-side variable in regression (4) generates regression (5), and the relationship disappears because, for the model, (5) is rather like regressing et on et–1. Similar logic explains the model results for regressions (6) and (7). Regression (6) is like regressing et + et–1 on et–1; which explains both the slope coefficient and the fact that R2 ≈ 1/2. Regression (7) is like regressing et + et–1 + et–2 on et–1 + et–2; which again explains the slope coefficient and the fact that R2 ≈ 2/3. As is clear from the table, there is a very close fit between the model results and all the empirical results reported by McCallum.
The last OLS regression reported by McCallum is
For each of his three exchange rate datasets, he shows that a1 ≈ 1 – a2 ≈ b, where b is the coefficient on the forward discount in the standard test for forward discount bias (regression (1) in Table 4). Equations (3) and (13) imply for the model that
where c1 ≡ 1 – c2 ≡ η, and η is the model coefficient on the forward discount in the standard test for forward discount bias (equation (13)). Thus, again, McCallum's empirical results are reproduced by the model.
We conclude this sub-section with a potentially serious shortcoming of our assumed money supply process. We assume the log-level of the money supply follows an ARIMA(0,1,0) process while Lyons (1990) points out that quarterly log-changes in the US money supply are approximately ARIMA(0,1,1). Lyons' observation implies that a current shock to the money stock leads rational agents to adjust their estimates of future money growth. So a money shock has both a direct effect on the exchange rate as well as an indirect growth-rate effect. Our assumed money supply process ignores the indirect growth-rate effect because it assumes that shocks to the money stock are independent of each other.
There are two relevant comments. Firstly, for realistic parameter values, the importance of the indirect growth-rate effect is unclear. If money supply growth rate innovations are truly permanent, the indirect effect is about twice as large as the direct effect (Lyons' Table 5). But if they are long-lived but temporary, as seems more plausible, his Table 3 suggests that the indirect effect is much less important. Secondly, recall our observation that only a small advantage is imparted to those (rational) agents who understand the true relationship between interest rates and the exchange rate. This key observation explains the long-run survival of a significant proportion of anchored traders. While Lyons' evidence represents a potential challenge to this observation, other empirical evidence (footnote 4) is strongly supportive of it. Note in particular the Meese and Rogoff (1983) finding that knowledge of the actual future money supply hardly helps at all to explain the level of the future exchange rate for horizons up to a year. To summarise, Lyons' point is an important conceptual one, but the balance of evidence strongly suggests that it does not overturn the key assumption on which our results depend – that only a small advantage is imparted to those who understand the true relationship between interest rates and the exchange rate.
Footnotes
As will soon be clear, the size of the unpredictable real exchange rate shocks, σe, determines our empirical results. But these shocks are the same in both cases of the model, so results for the general case should be very similar to those reported below. However, deriving results for the general case requires specifying values for two more parameters (γ and κ). [20]
A constant is included in the OLS regression to allow for different inflation rates between countries. Augmented Dickey-Fuller tests (Appendix D) imply, at least for the Japan–Germany and Japan–US interest differentials, that the hypothesis of a unit root can be rejected with some confidence. An alternative method for estimating θ using the autocovariance of the interest differentials gives similar results. [21]
θ = 50 periods is chosen because the OLS estimates of θ are biased downward. θ = 6 (θ = 50) implies that the half-life for goods-price adjustment is 3.5 months (32 months) which probably spans the plausible range for goods-price stickiness. [22]
Exchange rate volatility in the model is dominated by real exchange rate shocks,
but it also depends weakly on nominal shocks. Equations (11) or (13) imply
that:
.
With σe = 0.031 and
θ = 6 (θ = 50), σΔs
ranges from 0.031 (0.031) when α = 1 to 0.033 (0.037) when α = 0.
[23]
We assume that both types of traders always have at least an infinitesimal market presence so that investors can always observe their relative performance. One way to motivate this assumption is to imagine that new anchored and rational traders enter the market every period, but only when they are successful do they attract a non-negligible fraction of market wealth. [24]
Incorporating this Markov chain for αT into the rational traders expectations in Section 4 would add little of substance but greatly complicate the algebra. Our decision rule for investors is similar to the ‘Imitation Based on Realised Returns’ rule of De Long et. al. (1990a). As an alternative, the investors' decision rule could be based on the traders' relative realised utilities rather than their realised returns. This alternative assumption would reduce the proportion of anchored traders in equilibrium but would not change the qualitative nature of our results. De Long et. al. present reasons for preferring a decision rule based on realised returns: ‘we find it plausible that many investors attribute the higher return of an investment strategy to the market timing skills of its practitioners and not to its greater risk. This consideration may be particularly important when we ask whether individuals change their own investment strategies that have just earned them a high return. When people imitate investment strategies, they appear to focus on standard metrics such as returns relative to market averages and do not correct for ex ante risk’ (p. 724). Shiller (1992) presents supporting evidence (p. 70–74). [25]
Each year contains thirteen 4-week periods, and for each (α, n) pair, 10,000 realisations of the random variable Z(α,n) are used to generate results. [26]
Despite the fact that E Z(0,n) = 0, in all cases p(0,n) > 0.5 because the random variable Z(0,n) is slightly skew when n > 1. [27]