RDP 2003-01: Business Surveys and Economic Activity 4. What Information do Business Surveys Convey?
February 2003
- Download the Paper 304KB
This section examines the usefulness of business surveys in assessing the state and the outlook of the economy. A graphical examination is a useful first step in analysing the relationship between the various business survey indicators and other measures of economic activity. Figure 1 plots the main survey measures of sales/output or business conditions (relative to their long-run average) against year-ended growth in GDP. It shows that the survey indicators provide a reasonable picture of the major cyclical movements in output, particularly those surveys encompassing the early 1990s recession. However, the positive relationship between the level of the net balance statistic and year-ended growth in GDP suggests that there may be a strong backward-looking element to the survey responses, which needs to be taken into account in assessing the usefulness of a particular survey.
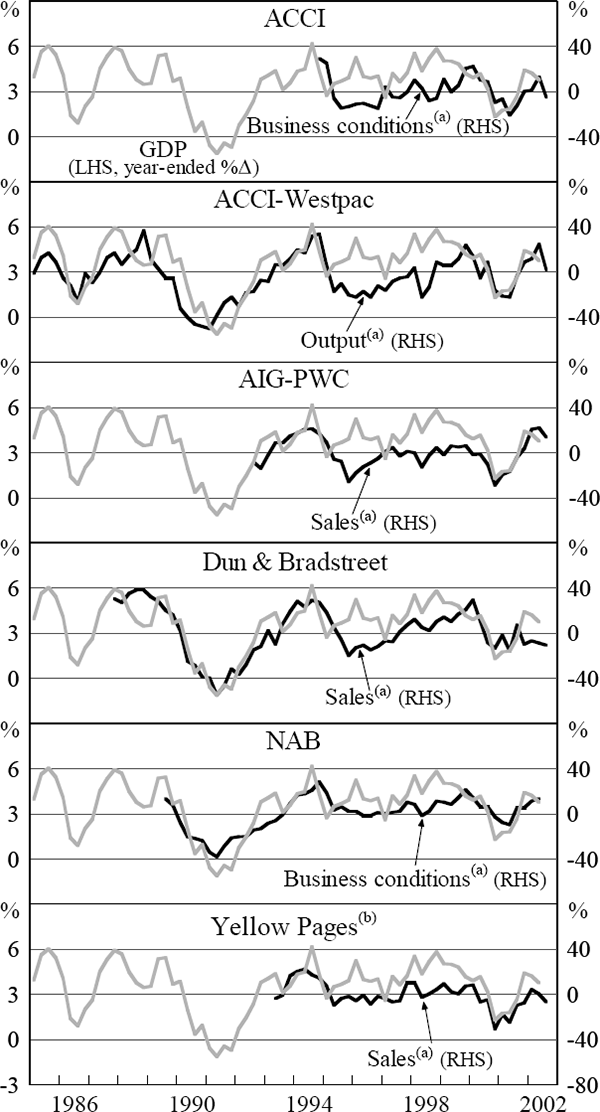
Notes:
(a) Deviation from long-run average.
(b) Prior to August 2001 data are for small businesses only.
More precise measures of the relationship between business survey indicators and economic variables can be obtained by:
- examining the pair-wise correlation coefficients of the business survey indicators with selected economic variables;
- adding survey information to a simple autoregressive model of an economic variable; and
- performing tests of Granger causality between business survey indicators and the various economic variables.
Correlation coefficients measure the degree of association between two variables. In the work that follows, correlation coefficients are measured over the full history of each series (so as to indicate how well the survey has performed over the life of the series) as well as over a common sample period in order to facilitate comparisons across surveys. The common sample period begins after the early 1990s recession period. Unless stated otherwise, correlations of expected business survey indicators and economic variables are undertaken based on the period to which the expectation refers. For example, the observation for the 12 months ahead that was published in the Yellow Pages June 2001 survey would be measured against growth in GDP over the year to June 2002.
The main appeal of correlation coefficients is that they provide a simple rule of thumb for judging the usefulness of a survey variable. However, they have limited predictive ability relative to regression-based techniques. An alternative approach is to add survey information to an autoregressive model of an economic activity variable. This allows us to examine whether the survey outcomes tell us any more about the economic activity variable than lags of the variable itself. To do this, we consider the following autoregressive models:
where ΔGDP refers to the quarterly growth rates of GDP; BC refers to the level of the net balance (relative to its long-run average); i refers to the individual survey; a refers to actual outcomes; and e refers to expected outcomes. (For illustrative purposes we use GDP and the survey variable, which in this case is ‘business conditions’ – other survey variables are investigated using other economic variables.)
Equation (1) focuses on actual business conditions as reported in a survey in the current period, whereas Equation (2) relates to expectations held in the previous period for the current period. From a forecasting perspective, both offer advantages in terms of timeliness, particularly the expected variable. The results of the tests are informative in a number of ways. First, a statistically significant survey variable implies that the survey indicator provides information in the estimation of the economic variable in addition to the variable's own history. Second, the coefficient on the survey variable, if significant, indicates the sensitivity of the quarterly growth rate of the economic variable to a one-unit change in the survey variable (all other variables remaining constant). This is, of course, a minimum test since there may be other variables which perform as good a role as the business sentiment indicator and be just as timely. Roberts and Simon (2001), for example, find that lagged economic indicators (such as changes in GDP, job vacancies and the cash rate) can explain a substantial proportion of the variation in a number of backward and forward-looking sentiment indices.
Another test, which focuses on how forward or backward-looking the survey responses might be, is the Granger causality test. Using the variables outlined above as an example, we begin with a simple two-variable model using six lags. The equations estimated are:
The lags of these models are further truncated on the basis of tests of significance, though for each survey the explanators in the two equations are kept identical.
Tests for Granger causality are then undertaken by testing the joint significance of business conditions in Granger-causing GDP (Equation (3)) and also the joint significance of GDP in Granger-causing business conditions (Equation (4)).[8] The results of the tests do not imply any strict causality or determination. In fact, our interpretation of the results is very pragmatic – rejecting the hypothesis that a survey indicator does not Granger-cause an economic variable implies that past values of the survey indicator provide some information which is useful in the estimation of the economic variable in addition to the variable's own history.
Footnote
The test is a Wald test, evaluated under a chi2 distribution. [8]