RDP 2009-04: Price Incentives and Consumer Payment Behaviour 5. Modelling Consumer Payment Behaviour for Australia
June 2009
In this section, we present the results of an econometric model for the holding and use of various payment instruments: credit cards; debit cards; and cash. As discussed in Section 4, there are three sets of factors that are potentially important determinants of whether a consumer uses a particular payment instrument: demographic factors; transaction characteristics; and our variables of interest – loyalty program participation and the interest-free period.[13] We are especially interested in how these price incentives affect the probability of using different payment instruments. Our dataset, compared with those used in most previous studies, is especially useful for this purpose because it records actual payment instrument use, as opposed to assumed most frequent use.
5.1 Modelling Framework
5.1.1 Credit card holding
In Australia, for the vast majority of everyday transactions, consumers will generally be able to pay with a credit card, debit card or cash. However, while cash and debit cards are held by almost all consumers, many do not have a credit card.[14] Hence, we first examine the main factors determining the probability of a consumer holding a credit card.
For our dependent variable, we only know the outcome of whether the consumer holds a credit card or not, rather than the utility derived from holding a credit card. Therefore, a latent variable approach is used. The dependent variable, CChold, is defined as 1 if the consumer holds a credit card and 0 otherwise and CChold* is the latent variable (that is, the utility derived from holding the credit card):
where j is the individual consumer and there is a set of N independent demographic variables, X, for each consumer. We use a probit model to estimate the probability of holding a credit card:
where Φ(•) is the standard normal cumulative distribution function.
Aside from demographic variables, we also considered the value of total weekly transactions as an explanatory variable for the probability of holding a credit card. We might expect that a consumer with relatively high expenditure would be more likely to hold a credit card. As the bivariate analysis showed, credit cards are used for all but very low-value transactions, and a consumer that makes either high-value or a large number of transactions may prefer to have a choice of card payment instruments rather than carrying cash. However, it may be equally likely that holding a credit card affects the weekly spending of consumers, either increasing it because they have the ability to borrow for an interest-free period and may make purchases earlier than otherwise, or decreasing it because they are paying interest on credit card debt and so have to reduce consumption.[15] This potential reverse causation means that the value of total weekly transactions may be endogenous, and its inclusion in Equation (2) could bias our estimates.
We attempted to control for this potential endogeneity using an instrumental variables approach. Unfortunately, our dataset does not contain a wide range of variables that both explain the total value of weekly transactions and are independent of factors affecting the decision to hold a credit card. The instrumental variables method did not produce meaningful results because the potential instrumental variables were only weakly correlated with the value of total weekly transactions. For this reason, our preferred approach is to exclude the endogenous explanatory variable and estimate the probit model for credit card holding using only demographic explanatory variables.[16]
5.1.2 Loyalty program credit card holding
Given our focus on price incentives, we are also interested in determining the characteristics of credit card holders that have a loyalty program attached to one of their cards. Therefore, for our sample of credit card holders, we estimate the following model:
where LPhold is defined as 1 if the consumer holds a loyalty program credit card and 0 otherwise. As with credit card holding, we estimate a model that only includes demographic variables. Again, we considered whether the value of weekly credit card transactions might influence the probability of holding a loyalty program credit card. However, the value of weekly credit card transactions is potentially endogenous and we do not have suitable instruments.
5.1.3 Credit card, debit card and cash use
Once we establish the characteristics of consumers holding a credit card, as well as credit card holders that have a loyalty program, we turn to the main focus of our paper: whether there is a relationship between price incentives on credit cards and broader consumer payment instrument use. To do this we follow Borzekowski et al (2008) and use our transaction-level data (pooling all transactions of all consumers) to estimate separate probit models for the use of credit cards, debit cards and cash.[17] Hence, for each payment instrument, the probability of use is modelled as:
where the dependent variable, Use, takes the value of 1 if the consumer used the payment instrument for a particular transaction – either credit card, debit card or cash – and 0 otherwise; i denotes an individual transaction, so Xi is a set of demographic variables specific to each transaction; there is a set of M transaction-related variables, T; LP indicates whether the consumer participated in a loyalty program or not; and I indicates whether the consumer regularly pays off their monthly credit card bill or not (that is, whether they are a transactor or a revolver). The standard errors have been adjusted to account for the fact that the observations are only independent across, rather than within, consumers.
We base these regressions only on those individuals who hold a credit card. This approach is consistent with that applied in previous studies; as Ching and Hayashi (2008) note, the focus is on the differences in payment methods between those who participate in a loyalty program and those who do not, rather than between credit card and non-credit card holders.[18]
Ching and Hayashi argue that it is possible that the loyalty program variable is endogenous (at least within the context of their dataset). They suggest three reasons why the loyalty program dummy variable may be positively correlated with unobserved consumer preferences for credit cards: consumers may choose loyalty programs because they use credit cards more, owing to a high preference for credit cards; the incentive of loyalty programs may induce consumers to use the credit card more often, thus the consumer learns the good features of the credit card and views the card more favourably; and card issuers may send pre-approved loyalty program invitations to consumers who have been heavily using credit cards. Their assumption is that there are some unobserved preferences for credit cards that are reflected in loyalty program membership; without controlling for these preferences, they argue the loyalty program dummy variable cannot disentangle the general preference for credit cards from the price effects of loyalty programs.
We consider the possibility that our estimate for the effect of loyalty programs may be affected by the considerations advanced by Ching and Hayashi (2008). We conclude, however, that differences in the nature of the data we use and institutional differences between the US and Australia mean that there is unlikely to be a meaningful endogeneity problem with the loyalty program variable in our regressions.[19]
Our general premise is that, once the consumer has chosen to hold a credit card[20] (dependent on preferences such as speed and safety), the predominant remaining reason they chose to join a loyalty program is whether there is a net benefit for them to do so; that is, whether they will use the credit card sufficiently to accumulate rewards that outweigh the higher annual fee generally associated with loyalty program credit cards. Loyalty programs do not affect the transactional convenience or other similar attributes of a credit card; thus, conditional on consumers choosing to hold a credit card, the separate decision to enrol in a loyalty program is, we argue, a reflection of a price effect and is precisely the effect we want to capture with our loyalty program variable.
A particular reason for the difference in conclusions is that we have transaction-level data rather than a single observation of the most frequently used payment instrument for each consumer. While it is plausible that a consumer's most frequently used payment method could influence their loyalty program membership within a consumer-level dataset where the observations are not well anchored in time, we think this concern is significantly reduced with transaction-level data. We do not expect that the consumer's use of a credit card for a particular transaction could have any effect on their enrolment in a loyalty program. We therefore suggest that the causation is only one-way – that loyalty program holding has an effect on credit card use but the use of a credit card for a given transaction does not affect a consumer's loyalty program membership over the two-week period of our sample.
In a similar vein, we also suggest that while a loyalty program may increase the use of a credit card – the effect we are trying to measure – it is unlikely that having a loyalty program will change the consumer's perceptions of the non-price features of credit cards (the second endogeneity concern, as raised by Ching and Hayashi 2008). One could argue that these features would be apparent to credit card holders regardless of whether they hold a loyalty program or not – particularly if many of those features are shared with other card-based payment methods to which people have access. These features should already be apparent given that credit cards are a mature and widely accepted payment instrument in the Australian payments system.
Finally, reflecting on Ching and Hayashi's third endogeneity concern, credit card use for a particular transaction in our two-week sample could not reasonably be expected to lead consumers to sign up for, or receive, loyalty program offers within our sample. A reason for this strong conclusion is the significant institutional differences between the Australian and US credit card markets. In particular, third-party credit card providers in Australia do not have access to the ‘positive’ credit reporting data that are used in the US to make credit card offers. Hence, Australian providers could not, in fact, make loyalty program offerings to high credit card users because they are unaware of who those users are.[21]
For these reasons, we conclude that loyalty program participation can be treated as a predetermined and exogenous variable in our regressions for payment instrument use.
5.2 Influences on the Probability of Credit Card Holding
The results from our model of credit card holding are presented in Table 4. The coefficients in the second column allow us to gauge only the direction of the variable's effect. Hence, in the last column we also report the marginal effect on the implied probabilities of a change in each of the independent variables, evaluated at the base case.[22] While some variables are surveyed in continuous form we converted these into dummy variables to be consistent across all our variables. Hence for all variables, the partial effect measures the estimated change in the probability of interest following a discrete change in the dummy from 0 to 1. The model is parsimonious to the extent that variable categories were excluded when the variables in the category were found to be jointly insignificant.[23]
Variable | Coefficient | Base case | Marginal effect (ppts) |
---|---|---|---|
Income (<$20,000) | −0.90*** | $40,000–$59,999 | −34.2 |
Income ($20,000–$39,999) | −0.46** | $40,000–$59,999 | −16.7 |
Income ($60,000–$79,999) | 0.15 | $40,000–$59,999 | 4.5 |
Income ($80,000–$99,999) | 0.23 | $40,000–$59,999 | 6.8 |
Income ($100,000–$119,999) | −0.29 | $40,000–$59,999 | −10.2 |
Income ($120,000+) | 0.80 | $40,000–$59,999 | 18.5 |
Scheme debit card | −0.37*** | No scheme debit card | −13.2 |
Cheque book | 0.41*** | No cheque book | 11.5 |
QLD | 0.34** | NSW/ACT | 9.8 |
VIC | 0.10 | NSW/ACT | 3.1 |
TAS | 0.13 | NSW/ACT | 3.9 |
SA/NT | −0.22 | NSW/ACT | −7.7 |
WA | 0.18 | NSW/ACT | 5.6 |
Regional location | −0.34*** | Capital city location | −11.9 |
Education (< Year 10) | −0.36** | University | −12.9 |
Education (Year 10/11) | −0.11 | University | −3.8 |
Education (Year 12) | −0.52** | University | −19.0 |
Education (Technical qualification) | 0.07 | University | 2.2 |
Constant | 0.64*** | ||
Number of observations = 579 Pseudo R2 = 0.16 |
|||
Notes: ***, ** and * denote the 1, 5 and 10 per cent levels of significance respectively. Marginal effects are evaluated at the base case. |
As shown in Table 4, we find that income has a large effect on the probability of holding a credit card, but it is only significant for lower income categories. We find that relative to a base income of $40,000–$59,999, the probability of a consumer with an annual income of between $20,000–$39,999 holding a credit card is 17 percentage points lower. This effect is even larger for those on an income of less than $20,000, at around 34 percentage points. The finding that low-income earners are less likely to hold a credit card is not surprising; however, what is interesting is that there is no statistically significant difference between incomes of $40,000 and higher. This suggests that the effect at lower income levels could reflect supply-side factors, for example the need to satisfy a certain eligibility criteria to obtain a credit card.
The regional (capital city or not capital city) variable has a significant and negative effect; compared to the ‘base case’ consumer living in a capital city, a person living in a regional location is 12 percentage points less likely to hold a credit card. There also appears to be some variation in credit card holding between the various states and territories.
The level of education of the consumer is significant. For example, a consumer whose highest educational qualification is less than Grade 10 is 13 percentage points less likely to hold a credit card than a consumer with a university qualification. This is consistent with Borzekowski et al's (2008) finding that higher levels of education tend to increase the probability of debit card holding. What this tends to suggest is that additional education may increase the probability of holding payment instruments aside from the more traditional methods, such as cash.[24]
It is perhaps not surprising to find that a consumer who holds a scheme debit card is around 13 percentage points less likely to hold a credit card than a consumer without a scheme debit card. For these consumers, the ‘scheme’ card is likely to be a reasonable substitute for a credit card, including for payments that are not face-to-face, such as internet payments.
In contrast, we find that if the consumer holds a cheque book then they are around 11 percentage points more likely to hold a credit card. While these two payment instruments are used for quite different types of payments, one similar feature is that they may assist with the consumer's personal accounting, because both provide a means to record the details of expenditures.
5.3 Influences on the Probability of Loyalty Program Credit Card Holding
Having established the characteristics of consumers who hold a credit card, an additional interest is to ascertain the characteristics of those consumers who hold a credit card with a loyalty program. The results from our parsimonious model of participation in loyalty programs are presented in Table 5.
Variable | Coefficient | Base case | Marginal effect (ppts) |
---|---|---|---|
Income (<$20,000) | −0.87*** | $40,000–$59,999 | −32.3 |
Income ($20,000–$39,999) | −0.28 | $40,000–$59,999 | −11.3 |
Income ($60,000–$79,999) | 0.30 | $40,000–$59,999 | 11.5 |
Income ($80,000–$99,999) | 0.90** | $40,000–$59,999 | 29.5 |
Income ($100,000–$119,999) | 0.55 | $40,000–$59,999 | 20.0 |
Income ($120,000+) | 0.73 | $40,000–$59,999 | 25.1 |
Regional location | 0.37** | Capital city location | 14.1 |
Part-time work | 0.64** | Full-time work | 22.7 |
Looking for work | 0.88 | Full-time work | 29.0 |
Not looking for work | 0.49 | Full-time work | 18.0 |
Retired | 0.62** | Full-time work | 22.2 |
Education (< Year 10) | 0.14 | University | 5.6 |
Education (Year 10/11) | −0.22 | University | −8.9 |
Education (Year 12) | −0.23 | University | −9.3 |
Education (Technical qualification) | 0.40* | University | 14.8 |
Constant | 0.14 | ||
Number of observations = 299 Pseudo R2 = 0.10 |
|||
Notes: ***, ** and * denote the 1, 5 and 10 per cent levels of significance respectively. Marginal effects are evaluated at the base case. |
While the results above suggested that consumers with lower levels of income are less likely to hold a credit card, we find that lower-income households that do hold a credit card are less likely to hold one with a loyalty program attached. This suggests that these lower-income consumers may be more likely to hold a credit card with lower annual fees and possibly lower interest rates, particularly if they are uncertain as to whether they will always be able to pay off their monthly credit card bill. This is also likely a reflection of their expected value from a loyalty program – with low income levels likely to also have low spending levels, meaning that the benefit they receive from the loyalty program may not offset the annual membership fee. We also find some tentative evidence that higher-income consumers may be more likely to hold a credit card with a loyalty program than the base case consumer with an income of between $40,000 to $59,999. Higher-income consumers may be more certain of their ability to pay off their credit card balance; hence, a loyalty program, as opposed to lower annual fees and interest rates, may be a more attractive credit card product.
Interestingly, we find that part-time workers and retirees are more likely to hold a loyalty program credit card than those that work full-time, other things equal. This seems somewhat counter-intuitive, though this variable may be also capturing effects of other debt holding that are not included in the model.[25] For example, compared to retirees, full-time workers are more likely to have other forms of debt, such as a mortgage. Hence, given their potential liquidity constraints, full-time workers may prefer a lower-rate credit card without a loyalty program to avoid high interest charges if they do not pay their credit card bill in full by the due date.
Despite the fact that consumers who live in regional areas are less likely to hold credit cards, those that do are more likely to have a loyalty program attached (than credit card holders in capital cities).
5.4 Influences on the Probability of Credit Card Use
We use the same set of demographic variables, as well as transaction-related and price incentive variables, to determine the main factors affecting payment instrument use. Hence, the variable we are interested in explaining is whether the consumer used a credit card or not for a particular transaction. For these models we include the same set of explanatory variables across the credit card, debit card and cash models for comparative purposes. Table 6 presents a summary of the results for the probability of credit card use.[26] Some explanatory variables have been excluded for presentation purposes; the full results are in Appendix B.
Variable | Coefficient | Base case | Marginal effect (ppts) |
---|---|---|---|
No loyalty program | −0.65*** | Loyalty program | −22.9 |
Revolver | −0.43*** | Transactor | −15.9 |
Male | 0.27** | Female | 10.9 |
Scheme debit card | −0.37*** | No scheme debit card | −14.1 |
Transaction ($0–$10) | −1.64*** | Transaction ($51–$60) | −41.0 |
Transaction ($11–$20) | −0.70*** | Transaction ($51–$60) | −24.6 |
Transaction ($21–$30) | −0.23*** | Transaction ($51–$60) | −9.1 |
Transaction ($31–$40) | −0.05 | Transaction ($51–$60) | −2.1 |
Transaction ($41–$50) | −0.08 | Transaction ($51–$60) | −3.1 |
Transaction ($61–$70) | −0.08 | Transaction ($51–$60) | −3.2 |
Transaction ($71–$80) | 0.11 | Transaction ($51–$60) | 4.4 |
Transaction ($81–$90) | 0.09 | Transaction ($51–$60) | 3.5 |
Transaction ($91–$100) | −0.07 | Transaction ($51–$60) | −2.7 |
Transaction ($101–$110) | 0.36** | Transaction ($51–$60) | 14.2 |
Transaction ($111–$120) | 0.45*** | Transaction ($51–$60) | 17.6 |
Transaction ($121–$130) | 0.16 | Transaction ($51–$60) | 6.3 |
Transaction ($151–$160) | 0.43** | Transaction ($51–$60) | 17.1 |
Transaction ($161–$170) | −0.07 | Transaction ($51–$60) | −2.7 |
Transaction ($171–$180) | 0.00 | Transaction ($51–$60) | 0.0 |
Transaction ($181–$190) | 0.63* | Transaction ($51–$60) | 24.4 |
Transaction ($191–$200) | 0.12 | Transaction ($51–$60) | 4.7 |
Transaction ($201+) | 0.03 | Transaction ($51–$60) | 1.0 |
Holiday/travel | 0.64*** | Retailer | 24.5 |
Health/medical | 0.20** | Retailer | 7.8 |
Petrol | 0.09 | Retailer | 3.7 |
Supermarket | −0.08 | Retailer | −3.3 |
Restaurant | −0.44*** | Retailer | −16.5 |
Small food store | −0.51*** | Retailer | −18.8 |
Professional services | −0.52*** | Retailer | −19.1 |
Sporting/entertainment | −0.66*** | Retailer | −23.4 |
Pub/bar | −0.75*** | Retailer | −25.7 |
Take-away | −0.93*** | Retailer | −30.4 |
Constant | −0.13 | ||
Number of observations = 8,575 Pseudo R2 = 0.28 |
|||
Notes: ***, ** and * denote the 1, 5 and 10 per cent levels of significance respectively. Marginal effects are evaluated at the base case. |
5.4.1 Demographic factors
Previous research has shown that demographic factors have an important effect on the probability of using different payment methods (Bounie and François 2006; Klee 2006; Borzekowski et al 2008; Emery et al 2008). In Section 5.2 we found that personal income can help to explain the probability of a consumer holding a credit card. However, once a consumer holds a credit card, income does not appear to have a statistically significant effect on the probability that the consumer will use their credit card for a given transaction. This is not entirely inconsistent with other studies; for example, Bounie and François (2006) do not find an intuitive income effect in their results for payment instrument use. In contrast, gender does not have a statistically significant effect on credit card holding, but it does play a role in determining the probability of credit card use, with males 11 percentage points more likely to use a credit card for a given transaction than females.
If the consumer also holds a scheme debit card, we find that the probability that the consumer will use a credit card for a given transaction is 14 percentage points lower than otherwise. This result provides some confirmation that scheme debit cards may be substitutes for certain types of transactions, particularly internet transactions. In contrast, despite cheque book holding having a significant effect on credit card holding, it does not significantly affect the probability of using a credit card. This is consistent with the likelihood that these two types of payment instruments are used for quite different types of payments.
5.4.2 Transaction characteristics
Our estimated model in Table 6 controls for a range of transaction-related factors that could affect credit card use. Low-value transaction amounts are significantly less likely to be associated with using a credit card, controlling for all other factors. For example, relative to a base case transaction of $51–$60, a consumer is 41 percentage points less likely to make a transaction with their credit card if the value is under $10. For these low transaction values it is more convenient in terms of tender time to pay with other payment instruments, particularly cash. Further, while this result controls for loyalty program participation, for transaction values of this small size, the benefit of rewards would be quite small because they are accumulated as a proportion of transaction value. For example, a $10 transaction would only accumulate around 6 cents in terms of a shopping voucher. Figure 6 shows, for a base case consumer, that as the transaction amount increases, the predicted probability of using a credit card tends to increase.[27]
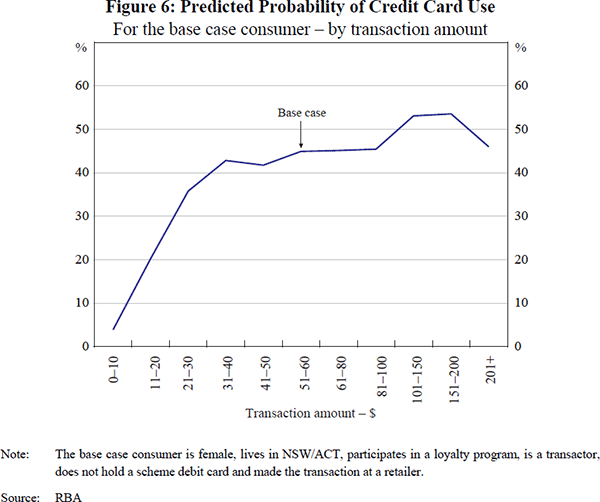
We also find that the type of merchant has a significant effect on the probability of the consumer using a credit card. Figure 7 shows the predicted probability of a base case consumer using a credit card at various merchant types. Our results suggest that relative to a purchase at a retail store, such as a department store, consumers are more likely to use a credit card when booking a holiday or travel and paying for health/medical purchases. The effect of holiday purchases may partly capture the relative likelihood that these payments are made online, compared to other merchant categories; a factor that is not included in these regressions because of potential collinearity with the merchant category variables. In Australia, because online EFTPOS is not available, credit cards have tended to dominate the online payments market. In contrast, there are a range of merchants for which the consumer is less likely to use a credit card (relative to the retailer base case); the largest effects were found for education/childcare, take-away and pub/bar purchases. For education/childcare the results likely reflect a lack of merchant acceptance; the survey is likely to include a number of babysitting or tutoring payments, and few tuition or textbook payments, because the survey was conducted around the middle of the school year. For pubs and take-away stores the results may reflect the slow tender time of a credit card transaction; as the consumer typically needs to sign for a credit card transaction, they may feel they are ‘holding up’ queues in these generally busy merchants. Alternatively, these merchants may not accept credit cards for similar reasons. As the availability of personal identification numbers (PINs) becomes more widespread for credit cards in Australia, the probability of credit card use at these types of merchants may increase.
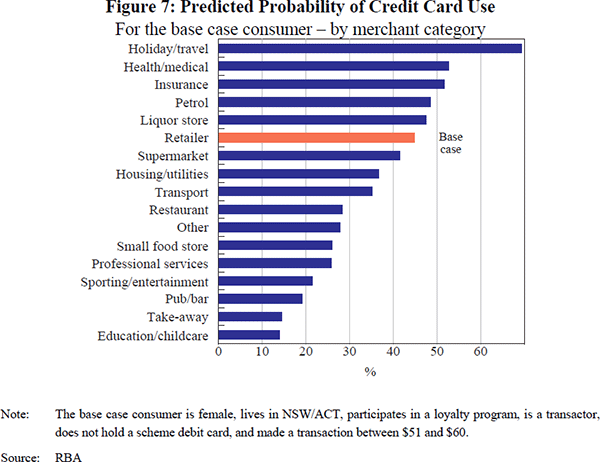
5.4.3 Price incentives
As foreshadowed by both the aggregate time-series data and the bivariate analysis of our survey data, price incentives on credit cards appear to play a significant role in credit card use. Our model shows a strong relationship between participation in a loyalty program and the probability of credit card use. The results indicate that the probability of a credit card holder using a credit card to make a payment is 23 percentage points lower for a base case cardholder that does not participate in a loyalty program, than a cardholder that does have a loyalty program. The effect is reasonably large given that the predicted probability of our base case person using a credit card is 45 per cent. In other words, there is a 45 per cent chance that a credit card holder with a loyalty program (who is female, is a transactor and does not have a scheme debit card), spending $51–$60 at a retail store will use a credit card to make the payment. This falls to a 22 per cent chance if the same cardholder does not have a loyalty program.
The results also suggest that access to an interest-free period on a consumer's card and the probability of credit card use are related. The results suggest that the probability of a credit card holder using a credit card decreases by 16 percentage points if the base case cardholder does not usually receive the benefit of the interest-free period. This effect is smaller than the 23 percentage point effect found for loyalty programs. One reason for this might be that some revolvers may still need to use their credit card owing to liquidity constraints, despite having to pay interest on these purchases, whereas a transactor that does not have a loyalty program does not face such constraints. The results in the next section also suggest that there is a qualitative difference between loyalty programs and interest-free periods that show up in the substitution patterns implied by the results for debit card and cash use.
5.5 Credit Card Price Incentives and the Use of Debit Cards and Cash
Tables 7 and 8 present a summary of the probit regression results for the use of debit cards and cash (the full results tables are provided in Appendix B). While the tables include the set of significant demographic and transaction-related variables, we focus our discussion on the effects of the credit card price incentives on these other payment instruments.
Variable | Coefficient | Base case | Marginal effect (ppts) |
---|---|---|---|
No loyalty program | 0.14 | Loyalty program | 4.0 |
Revolver | 0.58*** | Transactor | 18.9 |
Income (<$20,000) | −0.36*** | $40,000–$59,999 | −7.9 |
Income ($20,000–$39,999) | −0.05 | $40,000–$59,999 | −1.4 |
Income ($60,000–$79,999) | −0.15 | $40,000–$59,999 | −3.7 |
Income ($80,000–$99,999) | −0.33 | $40,000–$59,999 | −7.5 |
Income ($100,000–$119,999) | −0.74** | $40,000–$59,999 | −13.2 |
Income ($120,000+) | −0.26 | $40,000–$59,999 | −6.0 |
Transaction ($0–$10) | −1.27*** | Transaction ($51–$60) | −16.7 |
Transaction ($11–$20) | −0.51*** | Transaction ($51–$60) | −10.5 |
Transaction ($21–$30) | −0.28*** | Transaction ($51–$60) | −6.5 |
Transaction ($31–$40) | −0.25** | Transaction ($51–$60) | −5.8 |
Transaction ($41–$50) | −0.00 | Transaction ($51–$60) | −0.1 |
Transaction ($61–$70) | 0.10 | Transaction ($51–$60) | 2.8 |
Transaction ($71–$80) | −0.04 | Transaction ($51–$60) | −1.1 |
Transaction ($81–$90) | 0.11 | Transaction ($51–$60) | 3.0 |
Transaction ($101–$110) | −0.13 | Transaction ($51–$60) | −3.3 |
Transaction ($111–$120) | −0.10 | Transaction ($51–$60) | −2.5 |
Transaction ($121–$130) | −0.15 | Transaction ($51–$60) | −3.6 |
Transaction ($151–$160) | −0.29 | Transaction ($51–$60) | −6.6 |
Transaction ($161–$170) | 0.13 | Transaction ($51–$60) | 3.5 |
Transaction ($171–$180) | 0.15 | Transaction ($51–$60) | 4.3 |
Transaction ($191–$200) | 0.19 | Transaction ($51–$60) | 5.4 |
Transaction ($201+) | −0.20 | Transaction ($51–$60) | −4.7 |
Holiday/travel | −0.29 | Retailer | −6.7 |
Supermarket | 0.26*** | Retailer | 7.6 |
Restaurant | −0.62*** | Retailer | −11.9 |
Small food store | −0.28*** | Retailer | −6.5 |
Professional services | −0.41** | Retailer | −8.8 |
Pub/bar | −0.98*** | Retailer | −15.3 |
Take-away | −0.51*** | Retailer | −10.4 |
Housing/utilities | −0.74*** | Retailer | −13.3 |
Constant | −0.91*** | ||
Number of observations = 8,575 Pseudo R2 = 0.22 |
|||
Notes: ***, ** and * denote the 1, 5 and 10 per cent levels of significance respectively. Marginal effects are evaluated at the base case. |
Variable | Coefficient | Base case | Marginal effect (ppts) |
---|---|---|---|
No loyalty program | 0.35*** | Loyalty program | 13.6 |
Revolver | −0.08 | Transactor | −2.9 |
Scheme debit card | −0.14 | No scheme debit card | −5.1 |
Age (18–29 years) | −0.35** | Age (45–59 years) | −11.7 |
Age (30–44 years) | −0.15 | Age (45–59 years) | −5.2 |
Age (60+ years) | 0.23** | Age (45–59 years) | 8.8 |
Transaction ($0–$10) | 1.99*** | Transaction ($51–$60) | 60.5 |
Transaction ($11–$20) | 1.03*** | Transaction ($51–$60) | 39.1 |
Transaction ($21–$30) | 0.52*** | Transaction ($51–$60) | 20.4 |
Transaction ($31–$40) | 0.32*** | Transaction ($51–$60) | 12.5 |
Transaction ($41–$50) | 0.13 | Transaction ($51–$60) | 4.7 |
Transaction ($61–$70) | −0.17 | Transaction ($51–$60) | −5.9 |
Transaction ($71–$80) | −0.08 | Transaction ($51–$60) | −3.0 |
Transaction ($81–$90) | −0.32** | Transaction ($51–$60) | −10.7 |
Transaction ($91–$100) | −0.07 | Transaction ($51–$60) | −2.6 |
Transaction ($101–$110) | −0.38 | Transaction ($51–$60) | −12.5 |
Transaction ($111–$120) | −0.41** | Transaction ($51–$60) | −13.3 |
Transaction ($121–$130) | −0.02 | Transaction ($51–$60) | −0.8 |
Transaction ($151–$160) | −0.47* | Transaction ($51–$60) | −15.1 |
Transaction ($161–$170) | −0.11 | Transaction ($51–$60) | −3.8 |
Transaction ($171–$180) | −0.22 | Transaction ($51–$60) | −7.6 |
Transaction ($181–$190) | −0.56 | Transaction ($51–$60) | −17.4 |
Transaction ($191–$200) | −0.53* | Transaction ($51–$60) | −16.6 |
Transaction ($201+) | −0.32*** | Transaction ($51–$60) | −10.7 |
Holiday/travel | −0.35* | Retailer | −11.7 |
Health/medical | −0.22** | Retailer | −7.6 |
Petrol | −0.30*** | Retailer | −10.2 |
Transport | 0.42*** | Retailer | 16.5 |
Restaurant | 0.79*** | Retailer | 30.6 |
Small food store | 0.60*** | Retailer | 23.4 |
Pub/bar | 1.15*** | Retailer | 42.9 |
Take-away | 0.91*** | Retailer | 35.2 |
Constant | −0.42** | ||
Number of observations = 8,575 Pseudo R2 = 0.38 |
|||
Notes: ***, ** and * denote the 1, 5 and 10 per cent levels of significance respectively. Marginal effects are evaluated at the base case. |
A comparison of Tables 6 to 8 suggests that participation in a loyalty program or access to an interest-free period increases credit card use at the expense of using debit cards and cash. For debit cards we find that an interest-free period (on a credit card) has a significant effect on use but loyalty program participation does not. A consumer is 19 percentage points more likely to use their debit card if they do not usually pay off their credit card in full and therefore do not have access to an interest-free period; this finding is consistent in sign, but larger in magnitude than the effect found in Zinman (2008).[28]
For the cash regression we find virtually the opposite effect of credit card price incentives to those found for the debit card regression.[29] Access to a credit card interest-free period does not have a statistically significant effect on the probability of cash use. Consumers without a loyalty program, however, are 14 percentage points more likely to use cash for a particular transaction than another payment method.
It is worth examining why the degree of substitution between credit cards, debit cards and cash differs across price incentives and payment instruments. Consider first the effect of the interest-free period on the use of the three payment instruments. Based on interest costs alone, the price of using a credit card with an interest-free period is lower than that of using a debit card, which, in turn, is lower than a credit card where interest (at relatively high rates) is payable on a purchase. It is therefore logical that a cardholder paying interest might use a debit card rather than a credit card.[30] Of more interest is why there is little substitution towards cash when the cardholder does not have an interest-free period. Perhaps for transactions that a consumer already prefers to perform on a credit card, debit cards are a closer substitute.[31] Some support for this interpretation comes from the information in Section 4 on the use of cash; it is most commonly used for low-value transactions, such as take-away meals, while cards are used for larger transactions where speed of the transaction is less important.
The results for the loyalty program variable are somewhat more difficult to reconcile. Indeed, the finding that participation in a loyalty program has no significant effect on debit card use is somewhat at odds with US studies. For example, using a number of similar variables to our model, Ching and Hayashi (2006) and Sprenger and Stavins (2008) find fairly clear evidence that if the consumer has a loyalty program this increases the probability of credit card use and decreases the probability of both debit card and cash/paper-based instrument use. Although we suspect our loyalty program effect might not be precisely estimated,[32] the results could also suggest that consumers have strong preferences about the type of purchases made on debit cards and credit cards. For example, whether or not the purchase is made in a supermarket has a statistically significant effect on debit card use, which, as discussed in Section 4, may reflect factors such as the availability of EFTPOS cash-out at these merchants. Such factors apparently outweigh the benefit of earning reward points if the same purchase is made on a credit card.
Our results do, however, show substitution from cash to credit cards if the cardholder has a loyalty program. This can also be seen quite clearly in our raw data on the use of payment instruments across transaction values. For credit card holders without a loyalty program, cash is the most frequently used payment method for transaction values up to around $60, whereas for cardholders with a loyalty program, cash is only dominant for transactions up to around $30 (Figure 8). At higher transaction values, the presence of a loyalty program also appears to increase the use of credit cards.
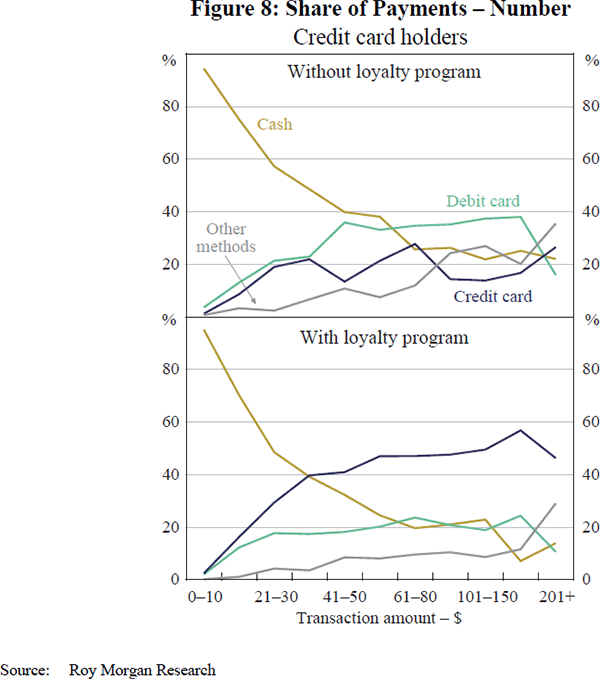
5.6 Implications of Results for Aggregate Payment Patterns
The results above provide some interesting insights into what factors drive consumers' choices about payment instruments. The results may also be able to give us some insight into broader policy issues, particularly in light of the Bank's reforms, which have affected the price signals facing consumers. In this section, we present a few hypothetical calculations to provide a sense of the aggregate economic significance of these modelling results.[33]
If, for some reason, loyalty programs had been removed from all credit cards in 2008, the above results suggest that the total value of credit card spending in 2008 would have been around 20 per cent lower.[34] Or, put another way, the total value of credit card payments in 2008 would have been around $163 billion rather than the observed $204 billion. Naturally, a change in loyalty programs would not occur in isolation and there would undoubtedly have been other changes occurring if this had happened. Hence, this estimate is best used to judge the economic significance of our parameter estimates rather than as a prediction of what might occur if loyalty programs were removed.
Nonetheless, the scenario still provides a basis for considering some developments that have occurred in the market for credit cards since the reforms. One of these has been a reduction in the value of rewards of around 0.2 percentage points of the amount spent (from around 0.8 percentage points in 2003).[35] Another development has been an emergence of low-interest rate credit cards generally without loyalty programs, though still with an interest-free period. These cards are aimed at revolver consumers, who represent around 34 per cent of credit card holders.[36] Over the past five years there has been a reduction in the accumulation of reward points from these two sources. The results from our hypothetical scenario suggest that the combination of such changes may be large enough to at least partly explain the observed fall in the growth of credit card use since the beginning of the reforms.
We can apply a similar hypothetical calculation to data for the late 1990s to obtain a lower-bound sense of the effect of loyalty programs over the past decade or so. For this earlier period, our estimates suggest that without loyalty programs, annual compound growth between 1994 and 1999 would have been around 12 per cent, compared to actual growth of around 20 per cent. As such, the widespread introduction of loyalty programs in the second half of the 1990s appears to account for some of the rapid growth in credit card use over that period.[37] A rough interpolation of our 1990s and 2008 calculations also suggests that without loyalty programs for credit cards, debit cards may have been the dominant payment instrument on a per capita basis throughout the entire period.
Footnotes
Loyalty program participation and interest-free period are captured by dummy variables. Loyalty program participation is defined as: 1 if consumer does not have a loyalty program and 0 otherwise. The interest-free period is defined as: 1 if the consumer does not have an interest-free period (is a revolver) and 0 otherwise (is a transactor). Owing to data limitations, there may be some transactors in the sample whose card does not have an interest-free period. This problem is likely to be relatively small, however, as at the aggregate level only 6 per cent of personal credit card accounts do not have an interest-free period, and revolvers are probably more likely than transactors to choose to hold these cards since they do not usually receive the benefit of any interest-free period. [13]
In Australia, an EFTPOS card is typically issued with the opening of a transaction account; hence, most individuals have a debit card. According to a Roy Morgan Research e-mail, 16 September 2008, around 91 per cent of individuals hold a debit card of some sort, compared to 53 per cent who hold a credit or charge card. [14]
Access to a credit card cannot affect the long-run level of consumption – it merely allows some inter-temporal smoothing of consumption expenditure. In the long run, income will determine consumption, but our sample may include people at differing points in this inter-temporal smoothing cycle, leading to some temporary effect. [15]
Klee (2006) and Borzekowski et al (2008) also estimate payment instrument holding on a set of demographic variables alone. [16]
Because this problem can also be treated as one where the consumer has a number of alternative payment options that are mutually exclusive and exhaustive, we could also use a multinomial logit model, similar to Bounie and François (2006). Our preliminary results from a multinomial logit model suggested similar effects in both sign and magnitude to those found for the less complex probit models. [17]
Also see Bounie and François (2006), Borzekowski et al (2008) and Zinman (2008). This focus on the sub-sample of credit card holders is one reason we do not model the two stages of credit card holding and credit card use in the Heckman selection framework. Kohler and Rossiter (2005) provide more detail on other reasons for preferring a two-part model over a selection model. [18]
In addition to the discussion below, we apply the two-step Rivers and Vuong (1988) endogeneity test on the transaction-level data. This test finds that we cannot reject the null hypothesis that loyalty program holding is exogenous. There are, however, some issues with applying this test in our particular model set-up; because we have a mix of both transaction-level and consumer-level data, adjustments for clustering on consumer-level variables need to be considered. Given that these adjustments are not established for this test, we do not emphasise these results here. Further, our first-stage regression for holding a credit card with a loyalty program does not have particularly high explanatory power. Notwithstanding these concerns, we consider the results to provide some independent grounds to proceed with our assumption that the loyalty program variable is exogeneous within the context of our model and dataset. [19]
As mentioned above, our question of interest requires us to restrict our sample to credit card holders. Therefore, any potential bias from credit card holding on credit card use has been eliminated. [20]
An additional facet of our data is that the loyalty program membership variable is recorded at a date prior to the survey rather than after the survey, so our variable is predetermined. [21]
As most of our explanatory variables are multiple-category dummy variables, it is more appropriate to evaluate the marginal effects at the base case, rather than at sample means. Our base case for each dummy variable category was generally chosen as the category with the highest proportion of the sample. An exception to this in the regression for credit card holding was personal income for which we chose a base case of $40,000 to $59,999 to reflect the average income of a person with (or currently completing) a university degree. [22]
We additionally required that all the excluded dummy variable categories were jointly insignificant. [23]
In their sample, only around 52 per cent of households held a debit card. [24]
We do not have any variables on the level or existence of other debt available in our survey. There is also evidence to suggest multicollinearity between the employment status and revolver variables; the employment status variables are jointly insignificant when revolver is also included in the regression. Not surprisingly, a revolver is less likely to hold a loyalty program credit card and instead probably hold lower-rate credit cards without reward programs attached. [25]
Again, our base case categories have generally been determined as the category with the highest proportion of the sample for each set of dummy variables. The exception in these regressions is the categories for transaction value. In this case we took the category corresponding to the average transaction value ($57, for those that hold a credit card); the highest proportion of the sample is accounted for by low-value transactions, that is $0–$10. [26]
The predicted probabilities shown in Figure 6 are based on a regression with wider transaction-value categories at higher transaction levels than in our preferred regression in Table 6. While our preferred regression illustrates the same point – that the predicted probability of credit use tends to increase with the transaction amount – the estimated effects are more variable for higher transaction values where sample sizes in the narrower categories are relatively small. [27]
Zinman estimates a marginal effect of around 17 percentage points for 2004 data and around 10 percentage points from the pooled 1995–2004 sample, using his preferred control variable specification. [28]
This different finding for the debit card and cash regressions is not driven by multicollinearity between the revolver and loyalty program variables in the cash regression, although there appears to be some interaction between the variables in the debit card regression. [29]
Ching and Hayashi (2008) find that substitution of debit for credit may not occur at all merchants. For example, access to an interest-free period was not found to have a significant effect on the choice of payment instrument at department and discount stores. They suggest that this may be because consumers ‘... borrow from their future incomes when purchasing items that are relatively more expensive’ (p 21). [30]
To test whether the presence of a loyalty program has a different effect across transaction values for credit cards, debit cards and cash, we estimated models, using wider transaction-value categories, including variables of the interaction of each transaction value dummy variable with the loyalty program dummy variable. The results are somewhat suggestive of the substitution effects described, although a more detailed analysis is an issue for further work. [31]
The effect of loyalty program is not particularly robust to various debit card model specifications. As mentioned above in Footnote 29, we suspect there are potential effects of multicollinearity. [32]
Ching and Hayashi (2008) conduct a similar policy experiment; however, their conclusions focus on the change in total card payment instruments (debit and credit), rather than just the change in credit card use. [33]
For this hypothetical scenario we assume that if there were no loyalty programs there would be a shift from the use of credit cards to cash in line with our regression results. We do not consider the proportion of credit card transactions that may shift to an instrument other than cash; that is, our regression results suggest that if the consumer does not have a loyalty program, they are 23 percentage points less likely to use a credit card for a particular transaction, but 13.6 percentage points more likely to use cash for a particular transaction. Specifically, we use the diary study and retail payment statistics collected by the Reserve Bank to estimate the number of cash payments in 2008 at around 7.7 billion, representing 58 per cent of the share of total payments. Data from the diary study over our estimation sample suggest that 85 per cent of credit card transactions are made by consumers with a loyalty program. Hence, if loyalty programs had been removed, the share of cash transactions would have increased by 13.6 percentage points on the 85 per cent of credit card transactions made by consumers with a loyalty program. This would have increased the overall cash share of total transactions to 69 per cent. This implies a hypothetical number of cash transactions of 9.3 billion – an increase of 1.6 billion. Multiplying this by the average cash transaction of around $26 implies an increase of $41 billion in cash payments. Subtracting this $41 billion from the actual value of credit card payments in 2008 implies a decrease of 20 per cent. [34]
Sourced from bank websites for the following cards: ANZ Telstra Rewards Visa card; Commonwealth Bank MasterCard Awards card; National Australia Bank Visa Gold card; and Westpac Altitude MasterCard. [35]
According to the diary study data. [36]
RBA and Australian Competition and Consumer Commission (2000). [37]