RDP 2010-04: Employment Composition: A Study of Australian Employment Growth, 2002–2006 3. Data
June 2010
- Download the Paper 309KB
In this paper, we estimate an econometric model that links individuals' employment status to a range of personal characteristics, and then test whether these relationships have changed over time. To do this, we use unit-record data on a large number of individuals from the HILDA Survey. Although it covers a relatively short time period, the HILDA Survey provides a wider range of information than that contained in the ABS Labour Force Survey (LFS) or in the Household Expenditure Survey.[3]
The dependent variable in our analysis is the current labour force status of the individual in the week prior to the interview for the HILDA Survey, which occurs each year around August and September. The definitions of employment, unemployment, marginal attachment and not in the labour force are identical to those used for the LFS.
The right-hand-side variables include a range of characteristics described in the literature as being relevant to employment status – that is, they influence either the supply of labour by an individual or the demand for the type of labour that might be provided by that individual. The literature in this area is extensive; for example see Carroll (2006) on the characteristics of the unemployed, Gray et al (2002) on the characteristics of the marginally attached, and Belkar, Cockerell and Edwards (2007) for a study of those not in the labour force. Borland (2000) and Le and Miller (2000) provide a review of earlier Australian studies. A robust finding of the literature is that factors likely to influence an individual's productivity, such as education, occupation and age, are positively related to the probability of employment, while factors that potentially constrain supply, such as having young children or alternative income sources, are associated with a low probability of employment.
In addition to these personal characteristics, we relate employment status to two variables capturing individuals' labour market history.[4] One is a measure of recent employment experience and the other a measure of lifetime employment experience. Recent employment experience is measured as the percentage of the previous financial year spent in employment. Defined this way, the current labour force status does not overlap with recent employment experience. Lifetime employment experience is measured as the percentage of time spent in employment since finishing full-time education. Besides capturing the potential effect of ‘scarring’ on future labour market outcomes, previous outcomes may also imply something about an individual's unobserved productivity level and her propensity to supply labour.[5] Including explana tors that capture past employment activity, therefore, controls for the unobserved heterogeneity across individuals, reducing the potential bias in the estimates of the coefficients on other variables.[6]
Continuous variables such as wealth and labour income appear to have a non-linear relationship with employment status (see Figure 2). To avoid specifying the functional form of the non-linear relationship, we converted all continuous variables into categorical dummies. The same general story emerges from the data if instead the models are specified using continuous variables and polynomial terms are included to account for non-linear relationships.
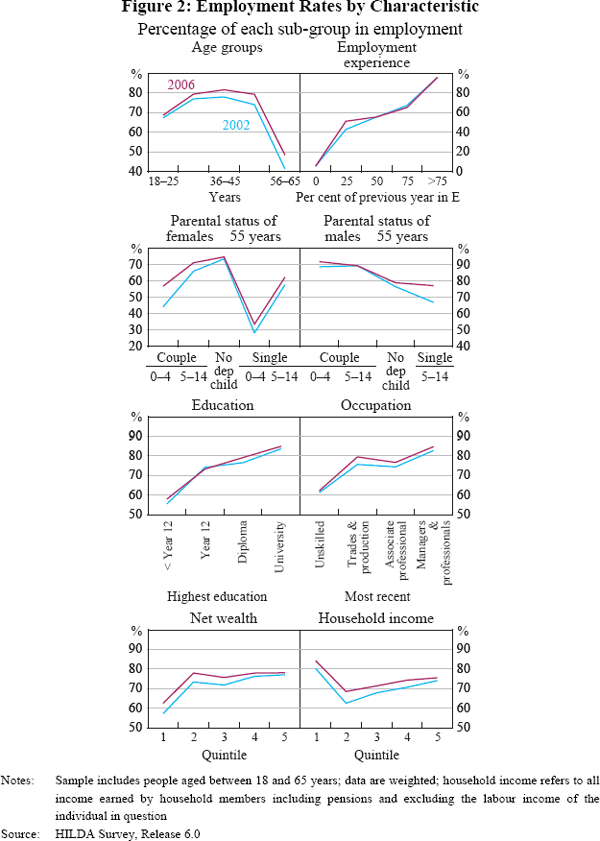
Following Belkar et al (2007), household income is defined as total income earned by all members of the household (including pension income) less the wages of the individual in question. It measures the income a person can access without working, assuming that the household pools the income of all members.[7] Net household wealth is calculated using information from the wealth modules in 2002 and 2006 and is defined as total household assets less total household debt. The debt-to-income ratio is total household debt from the wealth module divided by household income as described above. Defined this way, it measures the ability of the household to service its debts if the individual in question does not work.
Our sample consists of 17,606 individuals: 8,952 in 2002 and 8,654 in 2006. We restrict this further by considering only individuals aged 18 to 65 years (losing 5,227 observations), those who have had at least some work experience (losing 244 observations) and those with missing information for other explanators (losing 2,869 observations). Hence our results are conditional upon being of working age and having been previously employed.
The bivariate relationships between some key characteristics and the propensity for employment are displayed in Figure 2 for 2002 and 2006. From this we highlight two general results. First, there is clear evidence that certain characteristics are more strongly associated with employment: prime-aged workers are more likely to be employed than those aged over 55 years; individuals with higher education and managers and professionals are more likely to be employed than others; and women with older children or no dependents are more likely to be employed than those with younger children. In part, this reflects variation in the willingness of people at different stages of life to participate in the workforce, but it is also likely to reflect stronger demand for more productive individuals.
The second result, and our primary interest, relates to how these relationships have changed between 2002 and 2006. Across all characteristics shown here, the propensity for employment increased, reflecting the higher aggregate employment rate in 2006. Also, the relative propensity for employment across groups shows much the same pattern; for example, those with less than a high school education had lower employment rates in both 2002 and 2006. However, in most cases, characteristics associated with lower-than-average employment rates in 2002 tended to be associated with larger increases in employment between 2002 and 2006. In other words, there appears to have been a broadening of employment between 2002 and 2006, consistent with existing time series evidence regarding periods of economic expansion.
Footnotes
Developments reported by the HILDA Survey are also generally consistent with the LFS: the employment rate in HILDA increased from 62.0 per cent to 64.7 per cent between 2002 and 2006, compared to an increase from 59.4 per cent to 62.1 per cent in the LFS. A drawback of using the HILDA dataset for our study is that recent immigrants, who account for a large proportion of recent increases in labour supply, are under-represented. [3]
This places our model in the class of inertia models, which include variables measuring past employment experience to capture unobserved characteristics; see Le and Miller (2000). [4]
Scarring refers to the negative effects of a spell outside employment, which can be long-lasting (Gregg 2001). [5]
An alternative approach is to exploit the panel aspect of the HILDA data using a fixed-effects model. The main advantage of the fixed-effects model is that it controls for the effect of unobserved characteristics, potentially reducing omitted variable bias. However, the fixed-effects model cannot include any time-invariant explanators, such as sex, and the coefficient estimates may be biased if a lagged dependent variable is included. Further, any selectivity bias introduced by non-random attrition and measurement error in the explanators is exacerbated. We prefer the inertia model, which provides some control for unobserved characteristics and allows us to include time-invariant explanators. [6]
It also gives an imperfect indicator of the partner's employment status, which may influence an individual's labour supply (Le and Miller 2000; Breunig, Cobb-Clark and Gong 2005). [7]