RDP 2014-04: Home Price Beliefs in Australia 6. Determinants of Home Valuation Differences
May 2014 – ISSN 1320-7229 (Print), ISSN 1448-5109 (Online)
- Download the Paper 801KB
6.1 Panel Regressions
We now examine whether home valuation differences are systematically related to household and regional characteristics. To do so, we estimate several panel regressions of the following form:
where the dependent variable is the estimate of the average valuation difference
()
in each postcode and time period. A higher value for the dependent variable
indicates greater overvaluation relative to the market sale price for a postcode.
The specification includes a set of control variables (Xpt)
capturing average household characteristics in each postcode and period.
The control variables can be separated into three groups that potentially determine
household beliefs. First, there are demographic variables for each postcode,
such as the average age of the household head (Age) and average household tenure (Tenure) (i.e. the number
of years households have spent at their current address). Household tenure
is a proxy for the level of market information – or ‘housing experience’
– of each postcode. Second, there are business cycle variables, such
as the regional unemployment rate (Unemployment), which proxies for
the probability of being unemployed. Third, the controls include proxies for
the economic resources (or affluence) of households, such as (the log of) average
household disposable income (Log income), the share of households
with an education level above year 12 (Education) and the (log of
the) compositionally adjusted home sale prices . With the exception
of home sale prices, all the control variables are available from the HILDA
Survey.
The regression shown by Equation (6) also includes postcode fixed effects (θp) that capture unobservable factors that vary across postcodes but not time. The specification also includes year dummies (δt) that control for factors that are common to all postcodes at a point in time (for example, the business cycle). The results are shown in Table 2.
Age | 0.009* | 0.022*** |
---|---|---|
Age2 | −0.0000 | −0.0002*** |
Tenure | −0.007** | −0.006* |
Tenure2 | 0.0011* | 0.0001 |
Log income | 0.145*** | 0.032 |
Unemployment | −0.029*** | −0.084*** |
Education | 0.085*** | −0.001 |
Sale price ![]() |
−0.132*** | −0.598*** |
Time fixed effects | No | Yes |
Postcode fixed effects | No | Yes |
R2 | 0.139 | 0.721 |
Within R2 | 0.271 | |
Observations | 2,551 | 2,551 |
Notes: Robust standard errors clustered at the postcode level; ***, ** and * indicate significance at the 1, 5 and 10 per cent level, respectively Sources: APM; HILDA Release 11.0; authors' calculations |
Our preferred estimates are based on the postcode and time fixed effects regression results (column 2 of Table 2). The coefficient on the age and age squared variables suggests that home valuation differences are more positive for postcodes with older homeowners, but this effect becomes smaller as average age increases. At the mean, an increase by one year in the average age of homeowners within a postcode is associated with a higher average home valuation difference of about 0.25 percentage points.
In contrast to age, the coefficient on tenure suggests that postcodes with longer tenure on average are more likely to have negative valuation differences. This effect becomes smaller as average tenure rises. At the mean tenure of 12.5 years, an additional year of tenure, on average within a postcode, results in a predicted valuation difference that is 0.4 percentage points lower.
Higher regional unemployment is also associated with lower valuation differences. An increase in the average rate of unemployment in the region by 1 percentage point results in a decline in the predicted average home valuation difference of 8 percentage points.[9] We interpret this result as consistent with the idea that the degree of overvaluation (undervaluation) becomes smaller (larger) as the probability of becoming unemployed increases.
One reason that such an effect is plausible is that the expected costs of overvaluation – for example, spending too much, taking on more leverage or choosing a more risky financial portfolio (as discussed further below) – are likely to be higher the more likely a household is to experience unemployment. In particular, the most costly possible outcome is an extended period of unemployment that culminates in outright default. If households overvalue their home and have only small financial buffers – such as equity in the home or other assets – then the expected costs of optimism can be very high. The idea that households are less likely to be optimistic, or even are conservative (pessimistic), the more likely they are to experience an unemployment shock is consistent with recent literature on the optimal formation of household beliefs (see, for example, Brunnermeier and Parker (2005)).
Finally, it should be noted that the within-postcode explanatory power of the fixed effects regression is higher than the overall explanatory power of the least squares regression (27 per cent versus 14 per cent). This is due to the inclusion of time fixed effects, capturing the common trend in home valuation differences across all postcodes, due to, for example, the national housing cycle. Abstracting from these effects, the fixed effects model is able to account for around 10 per cent of the variation in home valuation differences within postcodes.
6.2 Quantile Panel Regressions
The previous results show the effect of household characteristics on average home valuation differences assuming these effects are constant across the distribution of beliefs. However, the effect of household characteristics on average home valuation differences may vary across postcodes depending on the extent to which they have a positive or negative valuation difference.
To account for this, a more complete analysis is undertaken by estimating a quantile function, which allows us to examine variation in the parameters over the full distribution of home valuation differences. This is useful because it allows us to see whether certain household characteristics affect either the bias or accuracy of average homeowners' beliefs. More specifically, if the household characteristic solely affects the level of bias, then the sign on the estimated coefficient should stay the same across the full distribution of beliefs. If the household characteristic affects the accuracy of beliefs, then the estimated coefficients should reverse sign between the top and bottom halves of the distribution.
To this end, for two selected characteristics – tenure and the local unemployment
rate – Figure 5 plots the quantile regression estimates for ranging from the 5th percentile of the home valuation difference distribution (the
far left of each panel, where valuation differences are large and negative)
to the 95th percentile (the far right of each panel, where valuation differences
are large and positive).
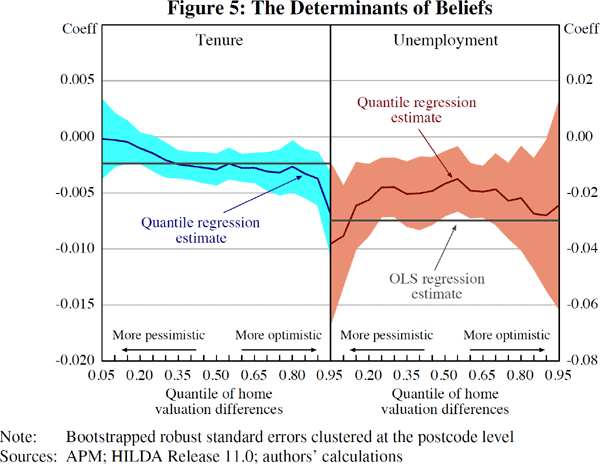
For example, focusing on the left panel, at the 5th percentile – a postcode with homeowners that appear very pessimistic on average – the estimated marginal effect of tenure is zero. In contrast, at the 95th percentile – a postcode with homeowners that appear very optimistic on average – the marginal effect of tenure is about −0.7 percentage points. This suggests that greater tenure attenuates optimism for postcodes with homeowners that appear very optimistic. The weak change in coefficient sign suggests that tenure may be capturing housing market information – or experience – within postcodes. In other words, this suggests that tenure affects the accuracy of home valuations rather than bias per se.
In contrast, the effect of the regional unemployment rate is estimated to be consistently negative across the distribution of beliefs, and so is about bias rather than accuracy. Interestingly, unemployment matters most (has the largest estimated marginal effect) at both tails. That is, in postcodes that are either very optimistic or very pessimistic, a higher local unemployment rate will reduce average valuation differences by more. For those postcodes who are unbiased or near the 50th percentile, the estimated effects of a higher local unemployment rate are statistically significant and negative, but smaller than the effects at the tails.
Footnote
The regional unemployment rate is measured at the city level. These results are robust to estimates of the unemployment rate at a more disaggregated level (the Australian Statistical Geography Standard, SA4). [9]