RDP 2016-11: Identifying Interbank Loans from Payments Data 4. Accuracy of the Algorithm
December 2016
- Download the Paper 3.19MB
To evaluate the accuracy of the algorithm, the daily output is aggregated and compared to IBOC Survey data.
Between 2005 and 2015, the daily correlations between the survey aggregates and the algorithm output are 90 per cent for lending and 92 per cent for borrowing (Figures 3 and 4).[22] As an alternative metric of accuracy (that better accounts for differences in the levels), for both borrowing and lending, our algorithm identifies 89 per cent of the value of IBOC loans between 2005 and 2015.
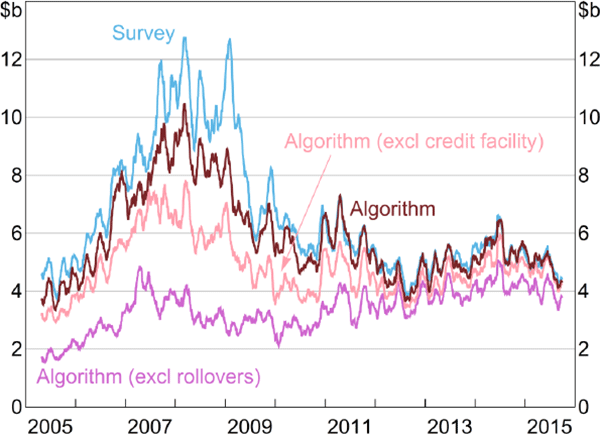
Note: For days when the institution was included in the survey
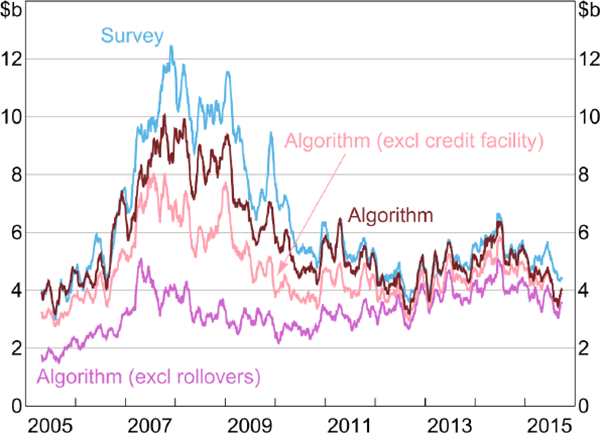
Note: For days when the institution was included in the survey
The full algorithm is noticeably more accurate than either the Furfine-type algorithm alone or the algorithm excluding rollovers.[23] The correlations between the Furfine-type algorithm output and the survey aggregates are 75 per cent for lending and 81 per cent for borrowing. Excluding all rollovers further reduces these correlations, to 24 per cent and 37 per cent, respectively (with the proportions of lending and borrowing identified falling to 51 per cent).
The algorithm is also accurate at the individual bank level. For the 22 banks that were included in the survey from early in the sample, over 60 per cent of their daily lending and borrowing correlations are greater than 80 per cent (Figure 5). That said, there are a few banks with very low daily correlations, due to our algorithm not identifying the majority of their IBOC market activity.[24] These low-correlation banks account for only a small share of IBOC market activity (based on the IBOC Survey data), which is why their low correlations have only a small impact on the aggregates discussed above.
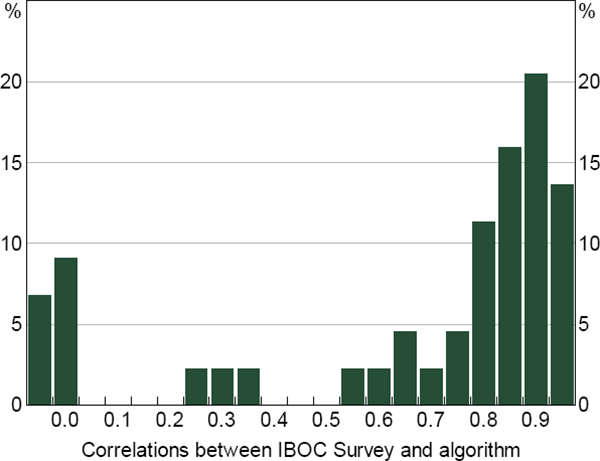
Notes: For days when the institution was included in the survey; horizontal axis by minimum value of bin, bin size of 0.05
It is notable that the algorithm performs very well for the four major Australian banks, with daily lending and borrowing correlations of 96 per cent (Figures 6 and 7).[25] The importance of the credit-facility part of the algorithm is clear in the big spikes in lending between 2006 and 2009. Without the credit-facility part of the algorithm, our algorithm would not have captured these short-term changes in the market.
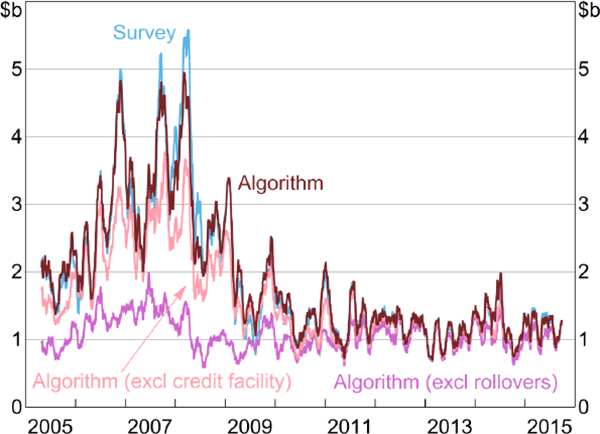
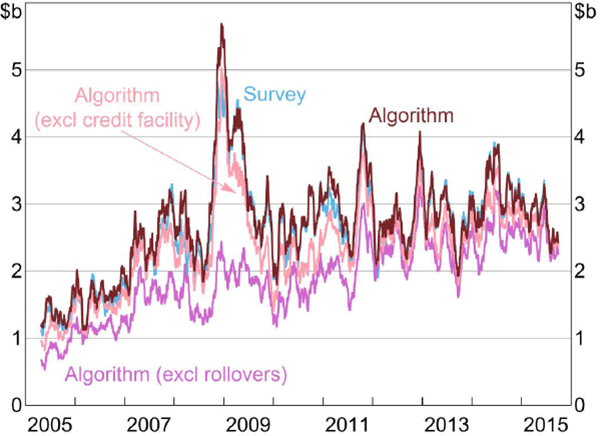
Our algorithm performs well relative to existing studies. This is due to both the algorithm design and the features of the Australian market. With IBOC loans almost always occurring at the target cash rate, we are able to set our implied interest rate range to 0 basis points (around the target cash rate), so there should be very few false positives (some studies exhibit high rates of false positives; see Appendix A).[26] By allowing for rollovers, our aggregate results exhibit high daily correlations with the survey data and identify 89 per cent of the value of all IBOC loans between 2005 and 2015 (the longest time series of any Furfine-type research of which we are aware). This suggests that the incidence of false negatives is comparable to, or lower than, existing studies (see Appendix A).
Footnotes
Even after extracting the trend components of these series (by taking first
differences), the daily correlations are little changed (84 per cent for lending and 83
per cent for borrowing).
For some of the sample period, the survey did not include all market participants, but
aimed only to capture the majority of loans (by value). As a result, in this section,
daily aggregates of the algorithm output only include participants who were surveyed on
that day. With only a subset of market participants included in the survey, the
aggregate value of lending need not equal the aggregate value of borrowing.
While the correlations are based on daily data, the high volatility of the daily data
necessitated the use of 30-day moving averages in the figures.
[22]
‘Excluding rollovers’ excludes the credit-facility algorithm and any loans identified by the Furfine-type algorithm that would not be identified if the algorithm only looked for repayments on the following trading day. That is, ‘excluding rollovers’ are the loans that would be identified by the existing algorithms in the literature. [23]
There might be some aspect of the lending/borrowing by these banks that our algorithm cannot pick up. For example, these banks may combine loan repayments with other transactions. [24]
The four major Australian banks are Australia and New Zealand Banking Group Limited,
Commonwealth Bank of Australia, National Australia Bank Limited and Westpac Banking
Corporation.
As noted in Section 2,
small over-identification for some banks could be due to these banks transacting on
behalf of other client banks.
[25]
For term lending to cause false positives, the rates on these loans would need to perfectly forecast future overnight rates and there would need to be no term premium. Secured loans are also unlikely to cause false positives, as the principal of these loans is typically based on the market value of the collateral, and is therefore unlikely to meet the ‘rounded’ requirement of our algorithm. [26]