Research Discussion Paper – RDP 2018-11 Consumer Credit Card Choice: Costs, Benefits and Behavioural Biases
October 2018
1. Introduction
Consumers face economic incentives to hold and use credit cards, such as rewards programs or an interest-free period. Most research that seeks to explain consumers' decisions in the credit card market highlights these incentives, and finds they are important predictors of behaviour (Simon, Smith and West 2010; Ching and Hayashi 2010; Arango, Huynh and Sabetti 2015; Lam and Ossolinski 2015).
But some consumers also incur high net costs from their credit card, in both interest charges and fees. Behavioural biases have been discussed as a potential explanation for at least part of these high costs (Ausubel 1991; ASIC 2015, 2018; Australian Treasury 2016).[1] Such biases may lead some consumers to choose credit cards with a high personal cost when lower-cost alternatives would have been available.
This paper estimates the net annual monetary cost or benefit that consumers receive from holding their credit card, and shows the distribution of these benefits and costs across different groups of consumers. Using these estimates, I test for the presence of behavioural biases and bounded rationality in the Australian credit card market. If they do affect card choice, these biases would likely reduce the value of the net benefit that consumers receive from their credit card relative to their optimal ‘rational’ choice.[2] I focus on three aspects of the decision process when consumers choose which card to hold: consumers' predictions of their likely spending behaviour; their estimates of the value of their options; and, their responses to short-term sign-up offers. I also consider the potential for cognitive barriers to prevent consumers from switching to a different card after they have made their choice.
To facilitate this analysis, I use data from the 2016 wave of the RBA's nationally representative Consumer Payments Survey, which provide a unique and detailed (anonymised) picture of Australians' payment patterns and preferences. In 2016, new questions were added to the survey, asking about respondents' credit card use and repayment patterns, the factors that affected their choice of credit card, and their perception of the overall benefit or loss from holding their credit card. Combining these survey data with data on the universe of credit card offerings in Australia, I estimate the net monetary value of credit card features for each cardholding respondent in the survey.[3] This estimate helps to answer two related research questions:
- How are the monetary costs and benefits of holding a credit card distributed across different consumers?
- Do behavioural biases appear to affect consumers' choice of credit card?
2. Literature
This paper estimates the net monetary value that consumers gain from holding their credit card, and assesses the potential effect of behavioural biases on this value. There are two streams of literature that are relevant to this analysis. First, literature relating specifically to consumer payments, and second, the behavioural science literature, particularly as it relates to consumers' financial decisions.
2.1 What Do We Already Know about Consumer Card Use?
Credit cards provide two distinct functions to consumers – they are both a means of payment and a source of credit. In addition, many credit cards also offer rewards programs, which provide rewards points or rebates that usually increase in proportion to the amount spent on the card. Both the credit function[4] and the offer of rewards points provide monetary incentives for consumers to use credit cards instead of alternative payment methods, such as debit cards or cash; in most other respects, credit cards provide very similar functionality to debit cards.
Previous empirical research has confirmed that, as may be expected, these monetary features do encourage consumers to use credit cards over other payment methods.[5] But they do not fully explain consumers' choice of payment method, and their importance varies across countries. For instance, Carbó-Valverde and Liñares-Zegarra (2011) find that credit card rewards programs have a smaller impact on credit card use in Spain than in Australia (Simon et al 2010) or the United States (Ching and Hayashi 2010).
In Australia, Simon et al (2010) use transaction-level data to estimate that, after holding merchant type, size of transaction and demographic characteristics constant, the presence of a loyalty or rewards program increased the probability of credit card use for a given transaction by 23 percentage points, while access to an interest-free period increased the probability by 16 percentage points.[6]
More recently, Lam and Ossolinski (2015) estimate consumers' willingness to pay a surcharge to use their card, as a measure of the consumer surplus from card use. This method captures both consumers' estimate of the net monetary benefit from using their card for a given transaction (e.g. rewards points, interest-free period), and the net non-monetary benefit (e.g. convenience, widespread acceptance). They find that respondents vary widely in the value they place on using their card, but in general, those with rewards cards had a higher willingness to pay a surcharge, and this preference persists after controlling for demographic characteristics and stated preferences.[7]
These findings suggest that the standard rational choice model does help to predict consumers' payment method choices at the point of sale. For instance, when a consumer is faced with the choice of using a credit card, from which they can earn rewards points and receive an interest-free period, or a debit card from which they earn no benefit, consumers are more likely to choose their credit card after controlling for other factors. It is probable that a similar decision process applies to consumers' choice of which type of credit card to hold. That is, we would expect consumers to choose a card that maximises their net monetary benefit.
But while monetary factors help to predict payment choices, they may not be perfect predictors. For instance, consumers may derive non-monetary benefits from their choices. This might mean that even if consumers appear to face net monetary costs from their credit card, they may receive a non-monetary benefit which outweighs these costs.[8] An additional possibility is that some consumers' decisions are influenced by principles of behavioural economics. Growing academic evidence suggests that consumers may be particularly susceptible to these biases at the time they choose which credit card to hold.
2.2 What Are the Potential Behavioural Biases?
The monetary benefit that consumers receive from their credit card depends both on the way they use their card and on the particular card they choose. To make an optimal card choice, consumers must first accurately estimate their own expected card use. Then, based on that expectation, consumers can choose the card that best suits their needs. Existing evidence suggests consumers' actual choices can systematically deviate from this optimal decision process.
First, consumers may have difficulty accurately estimating their expected card use. Some evidence suggests that consumers possess an ‘optimism bias’, systematically underestimating their likely card expenditure and their probability of incurring interest charges.[9] If, at the time of choosing their card, cardholders do not expect to incur interest charges (e.g. by underestimating their likely spending, or overestimating their future income and ability to repay), the interest rate is unlikely to factor into their decision and they may choose a high-rate credit card (Ausubel 1991; Calem and Mester 1995; Yang, Markoczy and Qi 2007). If that expectation turns out to be a systematic underestimation, these consumers will face substantially higher interest charges than if they had chosen a lower-rate card.
Second, identifying an appropriate card, given expected card use, can be a complex decision. Cards vary in their annual fees, interest rates, generosity of their rewards program, the number of interest-free days they offer, and the size of ad hoc fees – for example, late payment fees (Brainard 2017). This complexity can make the card choice computationally difficult. Consumers may be subject to ‘bounded rationality’, and may rely on heuristics (or simple rules of thumb) instead of attempting to compare their options across all dimensions. For instance, if consumers focus on some of the more salient or more widely-advertised features of the product (such as rewards points), bounded rationality may lead them to overweight the value of rewards points relative to the card's annual fee.
Consumers have been found to display bounded rationality in making these types of complex choices; for instance, in recent research on mobile phone plan choice in Australia. Using experimental data, Friesen and Earl (2015) find that consumers make significantly worse decisions when faced with complex tiered pricing schemes; for instance, where the included dollar value in the plan is different from the amount paid (e.g. a $30 monthly fee with $300 ‘included value’). But they also find that performance depends on consumers' level of expertise, with those who were already knowledgeable about phone plans making better choices. If extrapolated to the credit card market, we may expect a similar dynamic, especially in cardholders' estimates of the value of rewards programs. Rewards programs often reflect a similar multi-tier pricing structure, with no direct comparison between the number of rewards points and their dollar value.
Third, consumers' choice of credit card may also be affected by sign-up offers. If consumers are ‘present-biased’, they may respond to short-term sign-up offers of balance transfer deals, bonus rewards points, or discounted annual fees to choose a card that is relatively costly after those offers expire, leading to higher long-term costs.[10] Present-biased preferences are different from a standard economic model of exponential discounting because they imply some form of dynamic inconsistency – that is, if asked to choose a card for immediate use, present-biased consumers would be more likely to choose a card that has near-term benefits and longer-term costs. But if they were choosing a card today that they would start using in one year's time, they would choose differently.
Evidence of present bias in responding to credit card sign-up offers has been found in the US market. In an experiment, a credit card issuer sent card solicitations with varying introductory interest rate offers to 600,000 consumers (Shui and Ausubel 2005). They found that consumers were significantly more likely to respond to offers with a lower-rate introductory offer lasting just a few months than to offers of a slightly higher introductory rate that lasted longer. This led to higher long-term costs for many of those responding to the short-term offer, who continued to use their card to borrow at the higher interest rate long after the introductory offer had expired. Relatedly, descriptive evidence from Australia suggests that, between 2012 and 2017, around one-third of cardholders who responded to balance transfer deals had higher levels of debt after the offer had expired, suggesting some may have fallen into a ‘debt trap’ (ASIC 2018).
Of course, the effects of the three potential behavioural factors outlined above would only be noteworthy in the presence of substantial cognitive or practical barriers to switching cards. In the absence of any barriers, consumers would realise that they are making an avoidable loss, and switch to a lower-cost card.
In addition to potential practical barriers to switching cards (e.g. search costs, time spent applying for a new card and transferring any direct debits), there may also be behavioural barriers. Consumers may face similar behavioural barriers as with their initial card choice: optimism bias may lead consumers to believe that they will reduce their spending and eliminate interest charges in future, even if they systematically fail to do so; bounded rationality may limit their awareness of better deals and their ability to estimate the potential gain from switching. In addition, particularly if there is some uncertainty over the costs of switching and the value of alternatives, cardholders may exhibit a status quo bias towards their current card, even if it is not an optimal choice (Samuelson and Zeckhauser 1988; Kahneman, Knetsch and Thaler 1991).
A further possibility is that consumers who already experience significant financial stress could be more likely to make sub-optimal financial choices because of this stress (Kell 2016). This tendency is known as ‘cognitive scarcity’. A negative impact of cognitive scarcity due to financial stress has been found in other contexts; for instance, Mani et al (2013) found that low-income consumers, who are more likely to face financial stress, performed similarly to high-income consumers on a cognitive test in normal conditions. But their performance was significantly worse if they were ‘primed’ to think about financial stressors before taking the test, while high-income consumers were unaffected by the priming. If a similar effect is present for some credit card holders, it may help to explain continued use of cards with high net costs, even if lower-cost alternatives are available.
3. Data
The data used in this paper come from two sources: the RBA's 2016 Consumer Payments Survey, and information on advertised credit card features collected from card issuers' websites.
The 2016 wave of the Consumer Payments Survey contains data on 1,510 respondents representative of the Australian adult population, 72 per cent of whom held a credit card.[11] The survey includes information on respondents' demographic characteristics, card ownership and use of various payment methods as logged in a week-long diary. Full details of the survey methodology are available in Doyle et al (2017). Throughout most of this paper, I limit analysis to respondents who owned a credit card, reducing the sample to around 1,080 respondents.[12] I apply survey weights to all regressions, tables and graph data so that the final (reweighted) dataset aligns with demographic benchmarks for the Australian population.
This paper relies on a range of questions that were added to the Consumer Payments Survey in 2016. Questions were asked that were relevant to the behavioural hypotheses outlined above: on the features respondents considered most important when they chose their card, their perception of the monetary value of their card, and whether they signed up to their card with a temporary special offer. The survey also asked whether respondents had switched, or considered switching, cards in the past year.
To facilitate the calculation of the net monetary benefit, respondents were asked about how they use their main credit card. The survey asked about how respondents redeem their rewards points, whether they had paid a discounted annual fee, their usual card repayment behaviour, and which alternative source of credit they would use, if any, if they did not have a credit card. A copy of the relevant sections of the questionnaire is in Appendix F.
In addition to the survey data, I use data on the features of the particular credit cards held by respondents. As part of the Consumer Payments Survey, each respondent identified their main credit card (if they held multiple cards, this was the one they used most often). The detailed features of these cards, including fees, interest rates and rewards programs were obtained from card issuers' websites in late 2016. These data are collected by the RBA for internal analysis, though a range of comparison websites provide similar information.
Much of the information used in this analysis is self-reported. In many respects, self-reported information is valuable. We were able to ask respondents about their preferences and about which factors they considered to be important when they chose their credit card. But self-reported information on financial behaviours – such as frequency of card repayments, total monthly spending and monthly interest charges – may be subject to two potential response biases. First, there is a possibility of misreporting due to social desirability bias, with some evidence suggesting consumers are more likely to respond to surveys in a way that reflects favourably on their financial management skills (Kelly et al 2017). For instance, respondents may state that they ‘always pay off their balance in full’, even if this is not the case. This would lead to an underestimate of the value of interest charges. To minimise this potential bias, respondents were asked to refer to their most recent credit card statement in answering the questionnaire. Second, reliance on self-reported information reduces the sample size, as some respondents did not answer all questions, and others did not enter meaningful information in some fields.[13] As a result, I am unable to calculate the net benefit for around 20 per cent of credit card holders (after applying population weights), though dropping these respondents does not appear to meaningfully affect the results.[14] As an additional check on the representativeness of my sample, I compare components of the net monetary benefit with estimates from other sources in Section 4.3.
4. Estimating the Net Monetary Benefits of Credit Card Holding
4.1 Components of Net Monetary Benefit
In the RBA's 2016 Consumer Payments Survey, respondents were asked to identify the card features that were most important in their decision to hold a credit card at all, and then asked about the features that were most important when they chose their current main credit card. I use this information to identify which features to include in the calculated net monetary benefit.
In their initial decision to hold a credit card, consumers clearly valued a wide range of features.[15] Among the four most common reasons, two were monetary benefits – the interest-free period and rewards points – though a large share of consumers also valued non-monetary features (Figure 1). When asked to select the single most important reason for holding a credit card, three of the four most common responses were also monetary features – the interest-free period, rewards points, and the ability to borrow money and smooth spending. Most non-monetary features that cardholders valued were features that are now also available in debit cards (for instance, convenience, widespread acceptance and the ability to shop online).[16]
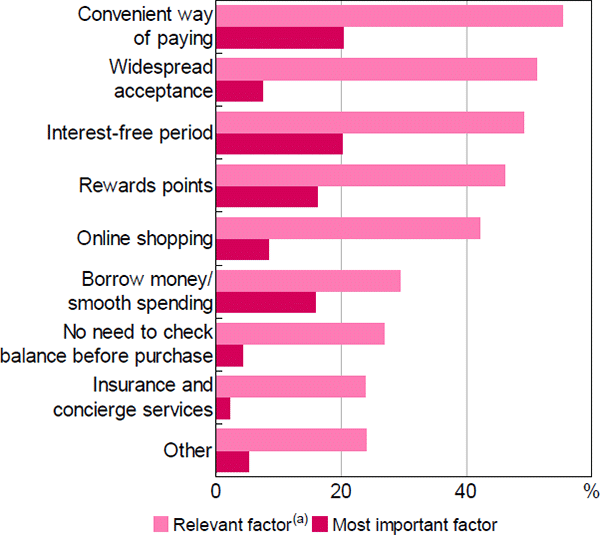
Note: (a) Respondents could select multiple responses
Source: Author's calculations, based on data from Ipsos and RBA
When asked about the reason for choosing their main card, monetary features were somewhat more prominent. The four most commonly selected monetary features were fees, rewards points, the interest rate and the interest-free period (Figure 2).[17] A relatively small number of respondents were motivated by other features that may also carry a monetary value, such as a sign-up offer, a balance transfer deal, or insurance and concierge services. The most common non-monetary factor that influenced card choice was that it was offered by the respondent's main or preferred bank.[18]
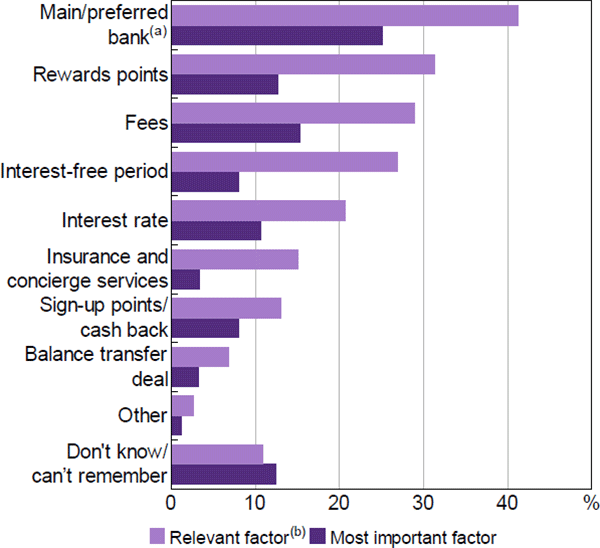
Notes: (a) Includes card offered by main/preferred bank, linked to mortgage/other accounts and customer service or quality of issuer's website/app
(b) Respondents could select multiple responses
Source: Author's calculations, based on data from Ipsos and RBA
This paper provides a first estimate of the distribution of the net monetary benefits that consumers receive from holding a credit card, based on the value of the four card features that respondents cite as most important. However, these are estimates only, as they do not reflect other monetary costs and benefits, such as the value of any insurances provided by the card, or the value of any sign-up offer or balance transfer deal that respondents may have received.
4.2 Calculation
Based on the above information, the four features that respondents identify as most important in their choice of card, and that carry a monetary cost or benefit, are the annual fee, the rewards program, the interest rate and the interest-free period. To estimate the net monetary benefit of cardholding, I sum the annual value of these components for each respondent:
The value of each component varies depending on the features of the particular card that the respondent holds (for instance, the generosity of the rewards program, the annual fee and the length of the interest-free period), and on the respondent's behaviour (their total spending, how they redeem their rewards points, their use of the interest-free period, the size and frequency of interest payments, and whether and how they would borrow money if they did not have a credit card).
The value of rewards points is based on an estimate of the number of points that each respondent accrues, multiplied by an estimate of the value of each point. The total number of points depends on the annual amount that the respondent spends on their credit card and the number of points their card accrues per dollar spent. The value of each rewards point varies depending on the card, and on how each respondent uses their points. For most respondents, I estimate a ‘conversion rate’ of rewards points to monetary value based on redeeming their points for a $100 gift card. For those who state they use their rewards points for flights, I base the estimate on the price of flights along major routes around the time of the survey, instead of the $100 gift card.
The value of annual fees is based on the fee advertised around the time of the survey. The advertised fee is scaled down by half if respondents stated that their annual fee had been discounted in the past year, and set to zero if they stated that it had been waived.
The value of the interest-free period is based on the respondent's alternative interest rate (e.g. savings deposit rate, mortgage rate, personal loan rate), multiplied by total annual spending and by the length of the interest-free period that the cardholder uses. This length is estimated based on the card's interest-free period (assuming that, on average, spending takes place in the middle of the statement month), and the portion of the interest-free period that the respondent reports that they generally use (for instance, respondents may pay off their card after each purchase, in weekly or fortnightly instalments, or on the due date).
The value of interest paid is reported by respondents who had accrued interest charges in their most recent monthly statement (and is equal to zero for all other respondents). They were asked to enter the value of new interest charges from their most recent monthly statement. This value was then scaled up, for an annual estimate of interest charges; it is multiplied by twelve months for respondents who state that they always repay the monthly minimum and nothing more, multiplied by three months for respondents who report that they sometimes pay off their balance in full, and sometimes not, and treated as a one-off (multiplied by one) for respondents who state that they always pay off their balance in full.
For each cardholder, the calculated net monetary benefit is based on the credit card that they use most often (their main credit card). For about 60 per cent of cardholders, their main credit card was the only card that they held, but some respondents held multiple cards.[19] Respondents with multiple cards are a heterogeneous group. Some appear to hold multiple cards because they are motivated by the rewards points that those cards offer, while others may hold multiple cards because they have reached the borrowing limit on their first card. These different types of respondents may use their main card in a different way from their second or third card, meaning that my estimated net monetary benefit may not fully reflect their overall credit card use patterns.
Importantly, my calculations include only the amount paid or received from owning a credit card, and do not take account of the respondent's opportunity cost or outside options. For instance, some respondents who use their card to borrow may face outside options – such as payday loans – from which they would likely incur higher net losses.
While the survey data provide detailed information, my calculations do require a range of assumptions and simplifications. Importantly, the elements of the net monetary benefit are based on spending and interest charges during a single month, but are scaled up to be expressed in annual terms. I apply a seasonal factor to these estimates to control for any month-specific effects.[20] In addition, card features are based on advertised conditions. Some respondents may have faced different fees or rates to those advertised for all or part of the year, and cardholders may incur incidental benefits or fees (such as late payment fees) that are not included in my calculations.
Full details on how each component is constructed, along with analysis of the sensitivity of my estimates to varying assumptions, are available in Appendix B. While different assumptions affect the value of the average net monetary benefit, the overall distribution of costs and benefits does not appear overly sensitive to my assumptions.
4.3 Comparison with Aggregate Measures
While my estimates of net monetary benefit vary across consumers, they suggest that, on average, consumers receive a net monetary benefit from their card that is not significantly different from zero.[21] This average estimate is different from the net cost or profit to card issuers, most notably because my calculations are based on the value of the interest-free period to the consumer, and not the cost of funds to the issuer.[22] My calculations also exclude a range of ad hoc fees that respondents may incur (such as late payment fees, over-limit fees and international transaction fees), and are based on the assumption that consumers with rewards credit cards use all of their rewards points. This assumption is likely to overestimate the value that consumers receive from their rewards program.[23]
I do not have a benchmark to compare my overall estimates, but I can compare two components of the net benefit with published aggregates: annual fees and aggregate credit card spending. Both comparisons suggest that my estimates are a reasonable approximation of the population average, but appear to be a slight overestimation of the net monetary benefit that the average cardholder receives.
The estimate of average credit card annual fees used in my analysis ($70) is somewhat lower than that from the RBA's 2016 survey of bank fee income ($137; see Fitzpatrick and White (2017)).[24] This difference may reflect over-reporting of the incidence of waived or discounted annual fees by survey respondents, or it may be that my sample includes a disproportionate share of consumers who had their annual fees discounted or waived. My decision to exclude respondents with very high annual fees (of $1,000 or more) from analysis also contributes to the difference. As an alternative to my preferred estimate of annual fees, I re-estimate the average annual fee including these very high-fee cards and using the annual fees advertised for the cards that respondents held, rather than adjusting for self-reported fee waivers or discounts. In this alternative measure, my estimate of respondents' annual fees increases to $130, closer to that of Fitzpatrick and White. However, I do not use this alternative measure in my analysis; I exclude very high-fee cards as I cannot capture the value of monetary benefits associated with these cards. In addition, the value of annual fee discounts and waivers may be important factors in respondents' choice of card; disregarding this information may cause bias in the opposite direction.
The value of credit card spending for each respondent is used as an input to the estimated value of rewards points and of the interest-free period. Higher spending leads to a larger benefit in both components. On average, credit card holders in the Consumer Payments Survey reported spending around $22,000 on their main credit card each year, which, based on the ratio observed in the survey data of main card to total credit card payments, suggests average annual credit card spending of $30,000. This is somewhat higher than an aggregate estimate of consumer credit card spending based on the RBA's monthly retail payment statistics, which suggests credit card purchases totalled around $24,000 per year per cardholder. As a result, my method is likely to overestimate the value cardholders receive from rewards points and from their interest-free period.
5. Distribution of Monetary Costs and Benefits
The net monetary benefit that cardholders receive from their credit card varies widely across individuals. Overall, I estimate that around 30 per cent of respondents incur a net monetary cost – meaning that their annual fee and interest payments were larger than the value of any benefits they receive from their interest-free period and from rewards points. Around 40 per cent gain a net monetary benefit, and around 30 per cent break even (defined as a net monetary benefit between −$50 and $50; Figure 3).
This section describes the distribution of the net monetary benefit across the demographic and stated preference variables that are the most economically and statistically significant predictors of the value of the net benefit (based on a simple regression framework, see Appendix C for regression results).
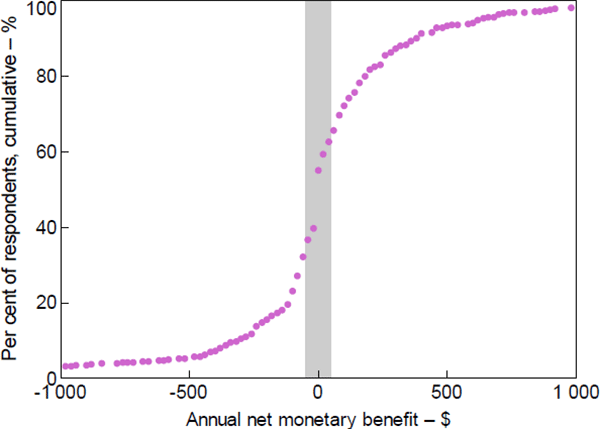
Note: Shaded area denotes the break even range
Source: Author's calculations, based on data from Ipsos and RBA
5.1 Distribution by Demographics
There are clear demographic patterns in the distribution of net monetary benefits. Consumers who receive the largest benefit tend to be those with relatively high incomes; half of those in the top quarter of the income distribution for their age received a benefit of $50 or more, around 25 per cent made a loss of $50 or more, and around 25 per cent broke even (Figure 4). Conversely, in the lowest income quartile, around 40 per cent of cardholders made a net monetary loss, under 20 per cent made a net gain, and around 40 per cent broke even.
While larger losses among lower-income cardholders were partly driven by a higher probability of borrowing, the overall pattern shown in Figure 4 persists even after excluding respondents who incurred interest charges; the distribution of benefits from credit cards across income groups also reflects higher average spending by higher-income respondents, which means they earn more rewards points and derive more value from their interest-free period.
A similar pattern is evident when we instead consider a measure of liquid wealth. Respondents with higher values of bank deposits generally receive larger net monetary benefits from their credit card. As with higher-income respondents, high-wealth respondents were less likely to use their card to borrow and to incur interest charges. They also tended to spend more, leading to larger benefits from their card's interest-free period and from any rewards program (Figure 5).
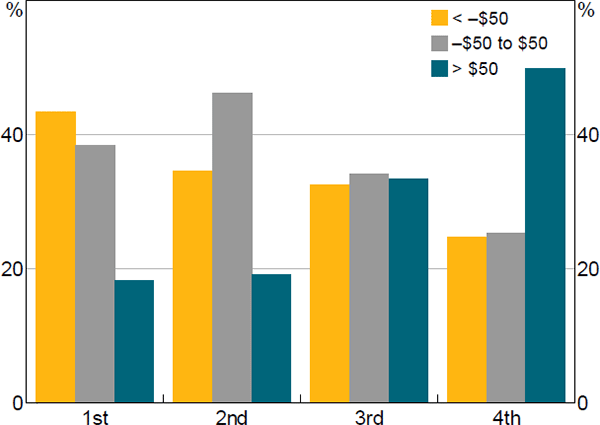
Source: Author's calculations, based on data from Ipsos and RBA
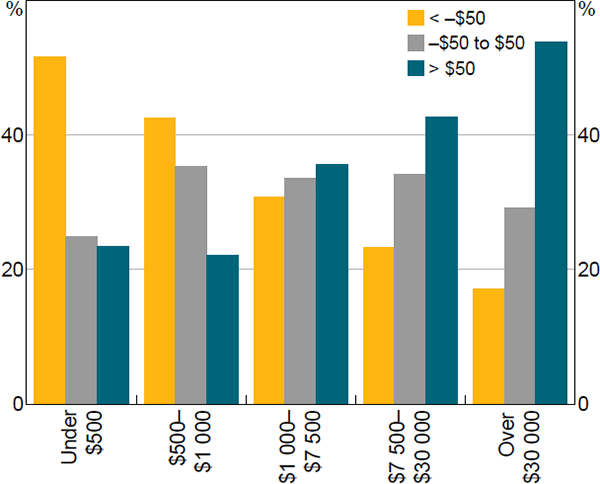
Source: Author's calculations, based on data from Ipsos and RBA
The value of consumers' net monetary benefit also varies with other demographic factors such as their age, education and employment status; in particular, those with positive net benefits tend to be employed, more highly educated and in the middle-age groups (Table 1). However, these demographics are also strongly correlated with income and bank deposits, and therefore they lose significance within a regression framework (see Appendix C).
Loss greater than $50 | Break even (−$50 to $50) |
Benefit greater than $50 | |
---|---|---|---|
Age (years) | |||
18–29 | 24 | 48 | 29 |
30–39 | 27 | 28 | 45 |
40–49 | 26 | 25 | 49 |
50–64 | 38 | 30 | 32 |
65+ | 29 | 38 | 33 |
Employment status | |||
Employed | 26 | 31 | 43 |
Not employed | 43 | 26 | 32 |
Retired | 33 | 38 | 29 |
Education | |||
Did not complete year 12 | 44 | 39 | 17 |
Year 12 | 36 | 29 | 35 |
Certificate/diploma | 34 | 34 | 33 |
University degree | 21 | 29 | 50 |
Source: Author's calculations, based on data from Ipsos and RBA |
5.2 Distribution by Motivation for Holding a Credit Card
Different consumers have different reasons for holding a credit card (see Section 4.1). Based on respondents' stated initial motivation for choosing their card (see Figures 1 and 2), and other relevant survey questions, I identify four prominent ‘types’ of credit card holders: those motivated by borrowing; those who see their card mainly as method of payment; those motivated by rewards points; and those motivated by other benefits that carry a financial value, such as the interest-free period and travel or rental car insurance.
Eleven per cent of cardholders were motivated to use their card to borrow – that is, these cardholders stated that the most important reason for having a credit card was either to borrow money and smooth spending or the interest-free period, and they reported that if they did not have a credit card they would want to borrow money in some other way. As may be expected, these respondents were substantially more likely to use their card to borrow outside of the interest-free period, and as a result incurred higher interest charges. The median respondent in this group made a net loss of $50, with many making substantially higher losses.
The largest group, making up almost 30 per cent of cardholders, were those who said they primarily value their credit card as a method of payment. These were respondents who appear to view and use their card in the same way as a debit card (for instance, reporting they have a credit card because it is a ‘convenient way of paying’), and stated they would not want to borrow money if they did not have a credit card. The median respondent in this group effectively broke even, with an annual net monetary benefit of $11.
A further 43 per cent of cardholders were motivated by either rewards points or other benefits such as the interest-free period and insurance, and stated they would not want to borrow money if they did not have a credit card. Cardholders who were primarily motivated by rewards points received the largest benefits – with just over 60 per cent receiving a net monetary benefit, with a median value of $105 – while the median respondent motivated by other benefits broke even (Figure 6).
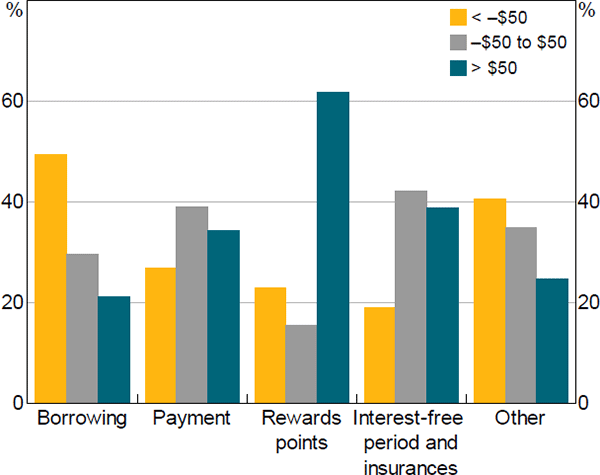
Source: Author's calculations, based on data from Ipsos and RBA
Around 20 per cent of respondents do not fit into any of these groups – the ‘other’ category includes, for instance, those who stated that they were primarily motivated by rewards points or other benefits, but would also want to borrow money if they did not have a credit card. These respondents typically made a net loss, though the average value of losses was smaller than for those motivated by borrowing.
5.3 Distribution Relative to ‘Optimal’ Card Choice
The monetary benefit that consumers receive from their credit card depends both on the features of the specific card they hold and on the way they use their card. To understand the relative contributions of these two factors on the overall distribution of costs and benefits, I estimate the net monetary benefit that respondents could receive if they had chosen the credit card that would maximise their net monetary benefit (their ‘optimal’ card).
To estimate the ‘optimal’ net benefit, I first calculate the net monetary benefit that each respondent would receive from the range of available cards, based on the way they use their actual card. I then assign to respondents the value of the card that would maximise their net monetary benefit.[25]
This ‘optimal’ net benefit should be seen as an upper limit on the monetary benefit that respondents could receive from a credit card, as it assumes that all respondents would be eligible and approved for their optimal card. The difference between respondents' actual net benefit and their ‘optimal’ net benefit also likely overstates the potential gains from switching cards, as I do not account for search or switching costs.[26] Further, consumers may rationally choose a card other than their ‘optimal’ card due to difficulty in precisely predicting their card use patterns at the time of choosing their card, or because they value other card features not included in my calculation. Nonetheless, these estimates provide a guide for the magnitude of potential gains respondents could receive from choosing a card that is better suited to their actual use patterns.
I estimate that cardholders could be made better off by choosing a card that is better suited to their use patterns (Figure 7; assuming that holding a different card would not change behaviour).[27] The median cardholder's actual net monetary benefit was around $120 lower than it would be if they held their ‘optimal’ card.
Of those who made an actual net monetary loss, around 65 per cent could have avoided that loss altogether by choosing a different card. For these cardholders, the median difference in net monetary benefit between their optimal and actual card was around $150.
Most loss-making respondents who reported that they usually or often pay interest would continue to make a net monetary loss even with an ‘optimal’ card, but these losses would be substantially smaller.[28] Of the 10 per cent of cardholders who would make a net loss under the ‘optimal’ card distribution, the median value of those losses would fall to around $280, down from an actual median loss of around $530 (a reduction of $250).
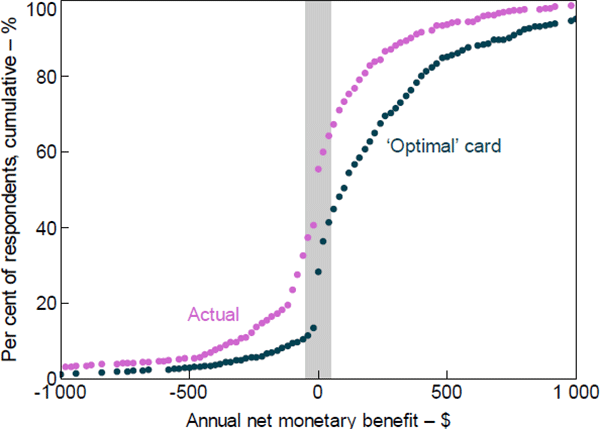
Note: Shaded area denotes the break even range
Source: Author's calculations, based on data from Ipsos and RBA
6. Evidence of Behavioural Biases?
I have found that a relatively high share of credit card holders made a net monetary loss on their card. But the fact that consumers make a monetary loss is not in itself evidence that they face behavioural biases or bounded rationality. Despite incurring a monetary cost, cardholders may still receive other net benefits – in terms of their overall consumer surplus or utility – from holding and using their card. For instance, they may value the ability to smooth their spending by borrowing, or they may value – and be willing to pay for – any non-monetary benefits that their card offers.[29]
The analysis in Section 5.3 suggests, however, that most cardholders would have been better off if they had chosen a different card, while still retaining the ability to borrow money and access to most non-monetary benefits. And most cardholders who made a loss could have avoided or significantly reduced such losses by choosing a card that better suits their card use patterns. This suggests that cardholders face some barriers to selecting an optimal card. To test whether these barriers reflect behavioural biases, I consider three hypotheses corresponding to the principles of behavioural economics outlined in Section 2.2. I assess whether consumers' choices in these three domains appear to depart from a standard economic model of rational behaviour.
For two of the three hypotheses, the data allow me to set up and test each hypothesis within a regression framework, to estimate the potential effect of the bias on consumers' net monetary benefit. For the other, the data do not allow for a formal test of the effect of the bias on the value of the net monetary benefit. Instead, I identify respondents within the dataset who appear to behave consistently with the proposed bias, and use regression analysis to consider demographic and other characteristics that are associated with this behaviour.
6.1 Hypothesis 1: Optimism Bias
The interest rate that a credit card carries is not relevant to cardholders unless they incur interest charges. Consumers who do not intend to use their credit card to borrow may therefore rationally disregard the interest rate when choosing a card. In contrast, those who do intend to borrow on their card may be able to substantially reduce their interest costs by choosing a card with a lower interest rate.
But the survey data show that most people who pay credit card interest did not consider the interest rate when they first chose their card. This is true not just for those who say they paid interest as a one-off or missed their repayment deadline, but also for respondents who regularly pay interest. Just 40 per cent of respondents who reported that they usually or often pay interest, or were paying off a long-term debt, reported that they had considered the interest rate when they chose their card (Figure 8).
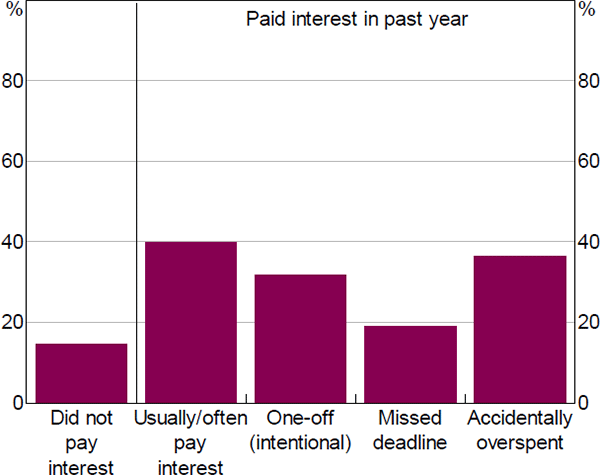
Source: Author's calculations, based on data from Ipsos and RBA
As a result, while some consumers who had paid interest in the past year held a card with a relatively low interest rate, most did not (Figure 9). For instance, among those who stated that they usually or often pay interest, the median interest rate was 19 per cent, just slightly lower than the median rate for respondents who did not pay interest at all (20 per cent).
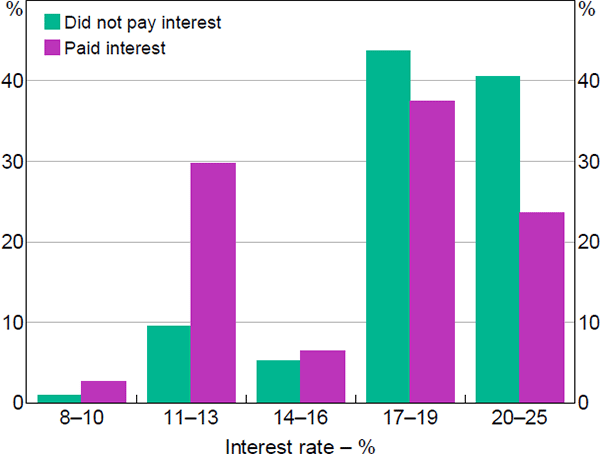
Source: Author's calculations, based on data from Ipsos and RBA
These patterns suggest that a large share of respondents who pay interest may have underestimated their probability of doing so when they first chose their credit card. On average, respondents appear more likely to underestimate this probability than to overestimate it. Twenty-two per cent of respondents had paid interest in the past year but had not considered the interest rate (indicating they may have underestimated their probability of paying interest). In comparison, just 9 per cent of respondents had considered the interest rate but had not paid interest (indicating they may have overestimated their probability of paying interest; Table 2).
These descriptive statistics suggest that at the time when consumers first choose their credit card, a material share may be overly optimistic about whether, and how much interest, they will pay; that is, they may be subject to optimism bias. I test this hypothesis within a regression framework.
Have you paid or owed interest on your credit card in the past year? | ||||
---|---|---|---|---|
Don't know | No | Yes | Total | |
Interest rate influenced choice of card(a) | ||||
No | 1 | 56 | 22 | 79 |
Yes | 1 | 9 | 11 | 21 |
Total | 2 | 65 | 34 | 100 |
Notes: Figures may not sum to column and row totals due to rounding Source: Author's calculations, based on data from Ipsos and RBA |
My null hypothesis is that, on average, cardholders are forward looking in predicting whether and how much they will borrow. If this hypothesis is true, consumers who considered the interest rate when they chose their card should be those who intended to – and on average, did – incur higher interest costs. My alternative hypothesis is that many consumers who ended up paying interest were overly optimistic about whether and how much they would borrow when they first chose their card. If this is the case, cardholders who did not consider the interest rate would, in fact, incur higher interest charges for the same level of borrowing.
To test these hypotheses, I run the following regression:[30]
I use two outcome variables: the dollar value estimate of interest paid over the past year and the value of the net monetary benefit.
considered interest rate is a binary variable equal to 1 if the respondent reported that the interest rate influenced their card choice, and zero otherwise. reason paid interest is a categorical variable indicating the reason for the respondent's most recent interest payment (options were: usually/often pays interest or paying off a long-term debt; one-off intentional interest payment; missed repayment deadline; or, accidentally overspent relative to budget).[31] The omitted category is cardholders who had not paid interest in the past year. X is a set of controls including household income, education, employment status, age and number of credit cards that the consumer holds. Extreme values of the dependent variable are censored using a 5 per cent Winsorisation – this reduces the magnitude of coefficients, but does not affect their level of significance.
I focus on whether respondents had considered the interest rate, rather than whether they held a lower-rate card, to abstract from potential supply-side factors. One possible explanation for the incidence of higher-rate credit cards among those who pay interest is that they may have a less favourable credit history, which would make it more difficult for them to obtain a card with a lower interest rate. If this were the main driver of the prevalence of high interest rates among interest payers, we would expect to see these respondents considering the interest rate as part of their decision, but still holding a higher-rate card.
The interaction term between having considered the interest rate and the reasons for paying interest controls for the composition of interest payers. For instance, as may be expected, many respondents who had paid interest but had not considered the interest rate had paid interest as a one-off. They had missed their repayment deadline or accidentally overspent in a given month. The interaction term in the model allows me to abstract from these one-off cases. I focus on the impact of having considered the interest rate for respondents for whom the interest rate is most important: those who state that they usually or often pay interest.
I calculate the marginal effect of having considered the interest rate for respondents who usually or often pay interest as a one-sided test. If the null hypothesis is true, then respondents who considered the interest rate and usually pay interest would pay higher (or equivalent) interest charges and would receive a lower total net monetary benefit. Conversely, under the alternative hypothesis, those who had considered the interest rate would pay lower interest charges or receive a larger net monetary benefit.
I find that, of those who regularly pay interest, the respondents who had considered the interest rate actually paid lower interest charges and received a larger net monetary benefit than interest payers who did not consider it (Table 3). This finding is consistent with the hypothesis that borrowers who did not consider the interest rate tend to pay higher interest charges for a given level of debt, because they are more likely to hold a higher-rate card. It may also reflect differences in borrowing behaviour across these different types of consumers. We can abstract from the effect of at least some of these differences by adding demographic controls to the model; doing so has only a small influence on the coefficients of interest.
Of the respondents who usually or often pay interest, those who had considered the interest rate incurred, on average, $80 less in interest charges than those who had not (Table 4). This difference persists after adding controls, and was significant at the 5 per cent level in the one-sided hypothesis test. Similarly, these respondents also received an average net monetary benefit about $105 larger, significant at the 5 per cent level. After adding controls, this fell to around $75 and was significant at the 10 per cent level.
Net benefit ($) | Interest paid ($) | ||||
---|---|---|---|---|---|
No controls (1) |
Controls (2) |
No controls (3) |
Controls (4) |
||
considered interest rate | −101.47*** | −82.22*** | −0.03 | 2.56 | |
(25.05) | (27.71) | (0.03) | (4.94) | ||
reason for paying interest (omitted category = did not pay interest in past year)(a) | |||||
Usually/often pay interest | −450.60*** | −394.25*** | 304.64*** | 293.67*** | |
(36.91) | (38.90) | (31.75) | (33.05) | ||
One-off intentional | −143.32*** | −107.36** | 125.63*** | 116.60*** | |
(47.02) | (49.47) | (27.59) | (27.43) | ||
Missed repayment deadline | −183.26*** | −168.90*** | 118.74*** | 114.22*** | |
(55.92) | (55.42) | (34.81) | (34.44) | ||
Accidently overspent | −166.33** | −149.67** | 99.10** | 86.43** | |
(65.22) | (64.10) | (49.00) | (43.36) | ||
Interaction term: considered interest rate × | |||||
Usually/often pay interest | 208.02*** | 158.97** | −78.92 | −83.69* | |
(66.46) | (64.09) | (50.37) | (49.68) | ||
One-off intentional | 119.52* | 75.24 | −44.75 | −33.16 | |
(71.34) | (70.89) | (38.32) | (39.22) | ||
Missed repayment deadline | 24.44 | 53.22 | 119.61* | 99.83 | |
(170.09) | (132.69) | (67.86) | (63.44) | ||
Accidently overspent | −3.32 | 14.52 | 40.31 | 49.21 | |
(80.62) | (78.32) | (62.08) | (56.69) | ||
Constant | 119.92*** | −104.53** | 0.03 | 18.59 | |
(12.36) | (45.41) | (0.03) | (23.64) | ||
Observations | 841 | 816 | 989 | 935 | |
Adjusted R2 | 0.24 | 0.32 | 0.39 | 0.40 | |
Notes: Controls are: household income quartile, age, education, employment status, number of credit cards, and typical value of bank deposits; extreme values of dependent variables are censored using a 5% Winsorisation; ***, ** and * represent statistical significance at the 1, 5 and 10 per cent level, respectively; robust standard errors are in parentheses Source: Author's calculations, based on data from Ipsos and RBA |
Net benefit ($) | Interest paid ($) | ||||
---|---|---|---|---|---|
No controls (1) |
Controls (2) |
No controls (3) |
Controls (4) |
||
106.56* | 76.75 | −78.95 | −81.14 | ||
(61.56) | (58.59) | (50.37) | (49.44) | ||
One-tail test p-value | 0.04 | 0.10 | 0.06 | 0.05 | |
Notes: ***, ** and * represent statistical significance at the 1, 5 and 10 per cent level, respectively, based on a two-tail test; robust standard errors are in parentheses; p-values in table relate to a one-tail test Source: Author's calculations, based on data from Ipsos and RBA |
These findings are consistent with the optimism bias hypothesis. They indicate that many cardholders who pay interest appear to have systematically underestimated their probability of doing so when they first chose their card.[32] But based on the available data, I am unable to entirely rule out other potential explanations for the observed patterns. For instance, it could be that respondents who incur interest charges do so because they have experienced a shock that was unpredictable at the time that they chose their card. If this type of negative shock is combined with barriers to switching to a more appropriate card (see Section 7), we would observe these same patterns. A definitive test of whether my findings represent optimism bias would require more detailed data, with information on whether respondents had experienced shocks or changes in their financial position since choosing their card.
6.2 Hypothesis 2: Bounded Rationality
Even if consumers are able to accurately predict their own card use behaviour, they may still choose a sub-optimal card due to a constrained ability to discover and compute the value of their options (Simon 1978). That is, consumers may be ‘boundedly rational’ in choosing their card. Factors such as limited time, lack of awareness of alternatives, or information overload may lead cardholders to form an incorrect impression of a card's value. These factors are particularly likely to affect decisions for more complex products.
I consider one potential – and important – consequence of bounded rationality; whether consumers have inaccurate estimates of the net monetary value of their own card.[33] To facilitate this, credit card holders were asked in the RBA Consumer Payments Survey whether they felt that the monetary benefits of their main credit card were greater than, equal to, or less than the monetary costs. Respondents were asked to consider fees, rewards points, interest paid and the interest-free period, so that their responses are comparable with the calculated net monetary benefit used in this analysis.
The distribution of respondents' perceptions of their net benefit lines up reasonably well with my estimates, though the share who believed that they made a net loss was substantially lower than my estimate. In total, around 40 per cent of cardholders believed they were financially better off from their credit card, 40 per cent reported they broke even and 15 per cent reported that the costs of their card outweighed the benefits (Table 5). A further 4 per cent were unsure.
Actual net monetary benefit | ||||
---|---|---|---|---|
Loss greater than $50 | Break even (−$50 to $50) |
Benefit greater than $50 | Total | |
Perceived net monetary benefit(a) | ||||
I'm worse off | 10 | 4 | 1 | 15 |
Neutral | 12 | 16 | 13 | 40 |
I'm better off | 6 | 10 | 24 | 40 |
Not sure | 2 | 2 | 0 | 4 |
Total | 30 | 32 | 39 | 100 |
Notes: Figures may not sum to column and row totals due to rounding Source: Author's calculations, based on data from Ipsos and RBA |
If bounded rationality affects cardholders' valuation of their card, we may expect them to overestimate its value; bounded rationality may lead consumers to place a disproportionate weight on advertised benefits when choosing a card, potentially placing a lower weight on costs which are not as prominently advertised.[34] I find that while most cardholders accurately estimated their position, around 6 per cent of all cardholders – or one in six of the respondents who believed they were making a net monetary benefit – were actually making a loss by my estimates.
Limiting my sample to respondents who, by my estimates, made a net loss on their credit card, I run a regression to understand which types of respondents were more likely to incorrectly believe they were making a net monetary benefit.[35] Within the sub-sample of loss-making respondents, I construct a dependent variable equal to 1 if the respondent perceived that they were making a gain, and zero for respondents who perceived that they were making a loss or breaking even. I regress this dependent variable on motivation for holding a credit card (as defined in Section 5.2), and on demographic and other explanatory variables.
While we saw in Section 5.2 that respondents who were motivated by rewards points were more likely overall to make large net monetary benefits from their card, if they made a loss, they were substantially less likely to be aware of it (Table 6, column (1)). After adding controls to hold demographic characteristics, liquid wealth and number of cards constant, this effect falls by nearly a third, but remains large; loss-making respondents motivated by rewards points were almost 27 percentage points more likely to believe they were making a gain.
Pr perceived gain | Pr perceived gain(a) | Pr perceived gain (Heckman)(b) | |
---|---|---|---|
(1) | (2) | (3) | |
Household income quartile (omitted category = lowest quartile) | |||
2nd | 0.02 | −0.02 | |
(0.06) | (0.04) | ||
3rd | 0.07 | −0.01 | |
(0.08) | (0.04) | ||
4th | 0.01 | −0.02 | |
(0.09) | (0.05) | ||
Employment status (omitted category = employed) | |||
Not employed | −0.07 | −0.00 | |
(0.07) | (0.05) | ||
Retired | 0.04 | −0.01 | |
(0.08) | (0.05) | ||
Education (omitted category = did not complete year 12) | |||
Year 12 | 0.18*** | 0.08 | |
(0.06) | (0.06) | ||
Certificate/diploma | 0.17*** | 0.13** | |
(0.05) | (0.06) | ||
Bachelor or higher | 0.23*** | 0.16** | |
(0.06) | (0.07) | ||
Motivation for holding credit card (omitted category = other) | |||
Rewards points | 0.37*** | 0.27*** | 0.06* |
(0.09) | (0.09) | (0.03) | |
Borrowing | 0.06 | 0.06 | 0.05 |
(0.08) | (0.07) | (0.04) | |
Payment | 0.09 | 0.05 | 0.04 |
(0.06) | (0.06) | (0.03) | |
Interest-free period and insurances | 0.17** | 0.09 | 0.04 |
(0.09) | (0.07) | (0.03) | |
Observations | 265 | 260 | 1,388 |
Notes: ***, ** and * represent statistical significance at the 1, 5 and 10 per cent level, respectively; standard errors are in parentheses Source: Author's calculations, based on data from Ipsos and RBA |
One potential explanation for this finding is that it reflects differences in the salience of benefits, relative to costs, for cardholders motivated by rewards points. While these cardholders may receive benefits from rewards points each month, they pay annual fees – often as an automatic deduction from their account – just once a year. Therefore, these respondents may underweight irregular costs (or equivalently, overweight regular gains) when estimating the value of their card.
This finding is consistent with an interpretation that consumers' inaccurate estimates of the value of their card reflect bounded rationality. For those who borrow and pay interest, interest costs are frequent and highly visible. But for those who do not pay interest, it can be more difficult to accurately weigh up costs and benefits. To add to this challenge, rewards card holders may have difficulty estimating both the number of rewards points they will accrue, and the value of those points relative to their annual fee. As Friesen and Earl (2015) find with mobile phone plans, wide variation in outcomes across respondents may be because some consumers navigate this complexity by developing expert knowledge of the market, while others do not.
Curiously, my results show that loss-making respondents were more likely to believe they were making a gain if they were more highly educated (Table 6, column (2)). The coefficients on education remain significant but decline substantially in magnitude in a Heckman sample selection probit model (column (3); see full results in Appendix D), suggesting that my model overstates the effect of education on the probability of overestimating a card's value.[36]
There are plausible reasons that more highly-educated consumers might overestimate the value of their card. For instance, as with respondents motivated by rewards, they may be less likely to pay interest charges, making costs less salient. Or they may devote less effort to developing expertise in the credit card market as they are able to absorb modest losses. But it should be noted that the demographic and preference variables used in the Heckman selection model can only control for observable factors, which together explain only a small part of selection into the group of loss-making cardholders (with an adjusted R-squared at this stage of just 0.05). Unobservable factors appear to be the main drivers of selection into the group of loss-making cardholders, creating the potential for the estimated effect of education to reflect omitted variable bias.
6.3 Hypothesis 3: Present Bias
Sign-up offers – such as bonus rewards points, a discounted annual fee, a temporary low interest rate or a balance transfer deal – are common in the Australian credit card market. Shui and Ausubel (2005) find that these types of short-term offers can appeal to consumers' present bias, attracting them to products that carry substantial long-term costs with continued use.
In the Consumer Payments Survey, around 30 per cent of credit card holders reported that they had originally signed up to their main card with a special offer (Figure 10). At least 10 per cent of cardholders had signed up to their card with an offer that no longer applied at the time of the survey.[37]
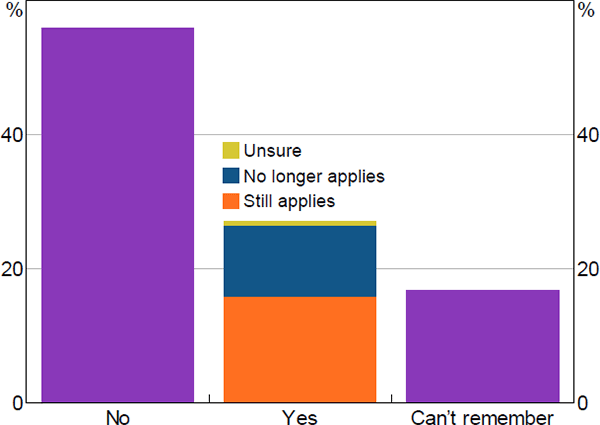
Source: Author's calculations, based on data from Ipsos and RBA
Outcomes for respondents with an expired offer can help to reveal whether consumers are present-biased when choosing a card. If so, we may expect those with an expired sign-up offer to receive a lower net monetary benefit from their card. This is because, at the time of choosing their card, present-biased consumers may overweight the short-term benefits they would receive from a sign-up offer, and may be inattentive to longer-term costs that they will incur after the offer expires.[38] Alternatively, if consumers respond to sign-up offers in line with a standard rational choice model, respondents with expired sign-up offers would be no worse off, after the offer expires, than similar respondents who did not sign up to their card with a special offer.[39]
Before controlling for other factors, it appears that average net monetary benefits for cardholders who had responded to a sign-up offer were substantially higher than for those who had not (Figure 11). The median net monetary benefit for respondents who had signed up with a special offer – whether or not it still applied at the time of the survey – was around $50. That is, respondents with an expired sign-up offer received, on average, a larger net benefit than respondents whose card choice was not affected by a sign-up offer.
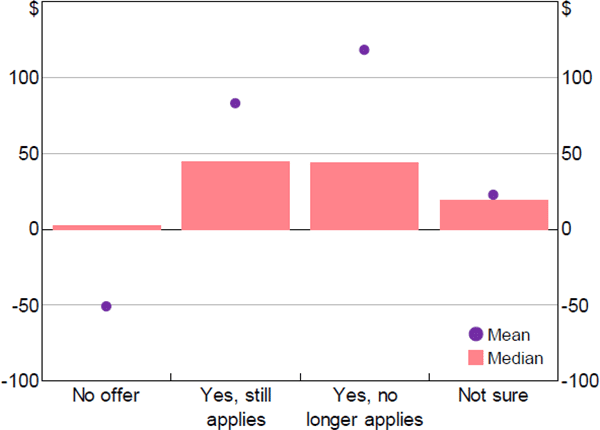
Sources: Author's calculations, based on data from Ipsos and RBA
These descriptive statistics suggest that cardholders do not incur absolute losses due to present bias. But I test for the effect of present bias more formally by estimating the effect of an expired sign-up offer on net monetary benefit, using the following regression:
where X is a set of control variables including household income, age, education, employment status, number of cards held and typical value of bank deposits.
I separate respondents into four categories depending on their self-reported card sign-up behaviour, corresponding to those on the horizontal axis of Figure 11.[40] The omitted category in the regression is respondents who had not signed up to their card with a special offer. I focus on respondents who received an offer that no longer applied at the time of the survey. I run this regression with the net monetary benefit as the dependent variable (Table 7), as well as with three of its components: the value of rewards points, the annual fee, and the amount of interest paid.
OLS coefficients | ||||
---|---|---|---|---|
Net benefit ($) | Rewards points value ($) | Annual fee ($) | Interest paid ($) | |
Opened card with sign-up offer (omitted category = no offer) | ||||
: Still applies | 56.82 | 3.55 | −3.48 | −60.28 |
(70.02) | (29.04) | (11.33) | (52.19) | |
:No longer applies | 128.69 | 106.17 | 5.80 | 30.84 |
(184.78) | (111.05) | (12.26) | (89.17) | |
:Can't remember | 38.78 | 9.88 | 18.89** | −39.41 |
(75.67) | (26.54) | (9.51) | (58.85) | |
Observations | 816 | 913 | 952 | 936 |
Adjusted R2 | 0.04 | 0.05 | 0.01 | 0.08 |
Notes: Base case is that respondent did not sign up to their card with a bonus offer; controls are: household income quartile, age, education, employment status, number of credit cards held and typical value of bank deposits; ***, ** and * represent statistical significance at the 1, 5 and 10 per cent level, respectively; robust standard errors are in parentheses Source: Author's calculations, based on data from Ipsos and RBA |
I test the present bias hypothesis by considering the coefficient . Under the null hypothesis (a rational choice model), the coefficient would be zero or positive. Under the alternative hypothesis (‘present bias’), the coefficient would be negative, which would indicate that respondents who signed up to an offer that no longer applies are worse off.
The results indicate that, consistent with the unconditional relationship shown in Figure 11, respondents who signed up to their card with a special offer that no longer applied were no worse off (Table 7).[41] In fact, the results suggest that if anything these respondents were better off than those who did not respond to a sign-up offer – though this difference is not statistically significant for the net benefit or any of its components.
Based on these findings, I fail to reject the null hypothesis. On average, respondents who signed up to their card with a special offer do not appear to be present-biased in choosing their card. These findings contrast with those of Shui and Ausubel (2005) who found that US consumers were present-biased in responding to temporary low interest rate offers. At face value, my results also appear inconsistent with ASIC's (2018) finding that a substantial share of consumers are worse off after responding to balance transfer deals.
There are a number of potential reasons for this contrast. First, my findings may reflect differences between the credit card markets of Australia and the United States. Most notably, there are important regulatory differences; the type of card solicitation tested by Shui and Ausubel (2005) would contravene Australian regulatory standards.[42]
Relatedly, my findings are likely to reflect differences in the nature of the offers being examined, and the types of consumers they attract. Shui and Ausubel (2005) consider offers of discounted interest rates only, while ASIC (2018) focuses on balance transfer deals. But questions asked in the Consumer Payments Survey cover all types of offers, so I do not have information on the nature of the offer that applies to each respondent. Overall, it appears that the consumers who respond to sign-up offers generally have higher incomes and higher levels of liquid wealth, suggesting that, on average, these offers are targeted at, or are more attractive to, higher-spending customers. It may be that card-issuing banks target offers to these groups, as they may have multiple financial service needs, making them attractive customers (Figure 12).[43] It therefore seems likely that for most cardholders who responded to a sign-up offer, these were offers of bonus rewards points or discounted annual fees, rather than discounted interest rates or balance transfers. It may also be that lower-income consumers or those with a poor credit history are not eligible for the types of cards that these introductory offers usually apply to, or that respondents who would make a loss after the introductory offer expires tend to switch cards in response. It is possible that, in aggregate, the positive or neutral outcomes for higher-income cardholders may outweigh potential negative outcomes for cardholders who respond to balance transfer offers or temporarily low interest rates; more detailed data would be needed to test this hypothesis.
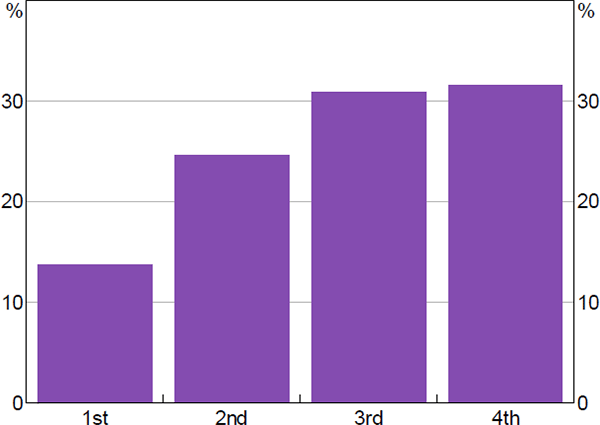
Source: Author's calculations, based on data from Ipsos and RBA
7. Barriers to Switching
While my estimates of consumers' net monetary benefits under an ‘optimal’ card choice (Section 5.3) suggest that most cardholders would be better off if they held a different credit card, only a relatively small number of cardholders switch cards. Around 7½ per cent of cardholders in the Consumer Payments Survey reported having switched their main credit card in the year prior to the survey. This share was marginally higher (9 per cent) for those who I estimate to be making a loss on their card, but the difference is not statistically significant.
These patterns suggest that cardholders may face some barriers to switching cards.[44] These barriers might be less binding for those who break even or receive a net monetary benefit from their card; they may feel no impetus to switch cards, even if they could receive a larger net monetary benefit if they did so. But we might expect that more than 9 per cent of those who make a loss – most of whom were aware they were making a loss – would want to switch to a lower-cost alternative. Respondents' stated preference to hold a credit card with their main or preferred bank (see Figure 2) may explain part of this reluctance to switch, though most banks offer a range of options, meaning that cardholders are able to switch to a lower-cost card issued by the same bank.
There are a number of possible reasons that respondents who make a loss might not switch cards. First, while I estimate that most cardholders would benefit from switching, for some, these benefits could be modest. For instance, cardholders who are paying interest and already have a relatively low-rate card may consider that any gain from switching cards could be outweighed by the time costs involved. In addition, a small number of cardholders state that they receive non-monetary benefits that they believe outweigh the monetary costs of their card (survey respondents were not asked to specify the nature of these benefits, see Appendix A). Collectively, these reasons appear to apply to just under 30 per cent of loss-making cardholders (Table 8).
A second explanation is that respondents may consider switching cards, but run into practical barriers and decide not to follow through. For instance, they may have found that they were ineligible for a lower-cost card, or may have investigated their options and decided that the cost of switching, in terms of the administrative burden and time involved, potentially including establishing a relationship with a new bank or card issuer, was too high.[45] This appears to be the case for a relatively small share of respondents (Table 8). Overall, around one in five cardholders had considered switching cards in the past year but had not followed through. Subtracting the respondents who already held a lower-rate card and who value the non-monetary benefits of their current card from this share, I estimate that these practical barriers could apply to up to 16 per cent of loss-making respondents.[46]
Share of loss-making cardholders (%) | Net monetary loss ($) | Share who considered switching (%) | Median annual fee ($) | Median interest rate (if paid interest) (%) | ||
---|---|---|---|---|---|---|
Median | Interquartile range | |||||
Switched card(a) | 9 | 209 | 324 to 89 | 100 | 99 | 14 |
Already holds suitable card | ||||||
Pays interest, has a lower-rate card | 25 | 224 | 862 to 81 | 36 | 59 | 13 |
Values non-monetary card features | 3 | 284 | 284 to 85 | 63 | 95 | 20 |
Not holding suitable card and | ||||||
Considered switching | 16 | 170 | 369 to 87 | 100 | 95 | 20 |
Did not consider switching – potential behavioural reasons | 47 | 170 | 341 to 82 | 0 | 95 | 20 |
Note: (a) Net monetary loss figures shown relate to the respondent's new card, which was their main credit card at the time of the survey Source: Author's calculations, based on data from Ipsos and RBA |
The above reasons appear to collectively apply to around half of loss-making respondents. The remaining half, therefore, appear more likely to face behavioural, rather than practical, barriers to switching. These consumers held cards with above-average fees or interest rates and they did not report that non-monetary features outweigh the monetary costs of their card, but they had not considered switching cards in the past year (Table 8). On average, these respondents made a median net monetary loss of $170, but with a wide range around that average. Their median loss would have been $230 lower had they chosen their ‘optimal’ card (as defined in Section 5.3).
There could be a range of reasons why respondents who did not appear to be holding a suitable card did not consider switching. It may be that these consumers were not aware of, or underestimated, the potential benefits from switching. Alternatively, they may have been aware of the potential benefits of switching, but faced behavioural barriers, such as status quo bias, present bias or cognitive scarcity, that prevented them from following through with researching and switching.
The survey data do not allow for identification of which, if any, of these potential behavioural explanations was the most important reason that these respondents did not consider switching cards. However, I find that the respondents who were more likely to consider switching (and more likely to actually switch) cards were those with higher incomes and higher levels of education.[47]
As may be expected, respondents who perceived that they were making a loss were more likely to consider switching cards than those who were not aware that they were making a loss (Table E1). While the estimates are imprecise, the effect of making a perceived loss appears to increase with income, with higher-income respondents who perceived they were making a loss being more likely to consider switching cards (Figure 13; see Appendix E for full regression results).[48] This difference across income levels may reflect differing options available to these different types of respondents; perhaps some low-income respondents do not consider switching because they do not believe it will be possible to find a card with lower costs. It may also reflect levels of financial literacy, or it may reflect behavioural biases, whose negative impact may be largest for consumers who use their card to borrow money (typically lower-income, liquidity-constrained individuals).
As noted in Section 2.2, one potential behavioural barrier to switching cards may be cognitive scarcity due to stress, which can impair consumers' ability to make optimal choices. Cognitive scarcity, or limited mental ‘bandwidth’, may lead consumers with pre-existing stressors to choose, and retain, cards that are inappropriate for their needs. For a small number of respondents, the stress itself may be caused by credit card debt, which may lead them to focus narrowly on repaying current debts, rather than taking steps – such as switching cards – to reduce future interest charges. Literature seeking to identify the effect of cognitive scarcity due to stress has generally focused on low-income consumers, who are most likely to face financial stress (Mullainathan and Shafir 2013). This effect may therefore help to explain why higher-income cardholders were more likely to devote time and mental bandwidth to considering switching cards.
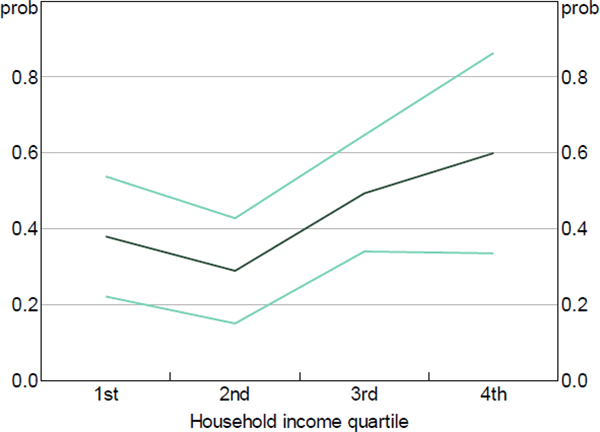
Notes: Probit model, includes demographic controls; bands show 95 per cent confidence intervals
Source: Author's calculations, based on data from Ipsos and RBA
8. Conclusion
Credit cards provide a convenience to consumers, acting as both a method of payment and a flexible credit instrument. We may expect then that most consumers would pay a modest net monetary cost to access this convenience. But the wide distribution of costs and benefits across cardholders instead suggests extensive variation in the credit card market, with some consumers paying relatively high net costs, and others receiving substantial net monetary benefits.
My estimates show that while around 40 per cent of credit card holders receive a net monetary benefit from their card, 30 per cent pay a net monetary cost, while the remaining cardholders break even. Consumers with lower levels of income and liquid wealth were more likely to incur net costs, partly, but not entirely, because they were more likely to use their card to borrow, and therefore incur interest charges. At the same time, higher-income respondents were more likely to hold rewards cards, and to receive substantial benefits from those rewards and from their cards' interest-free periods.
My analysis suggests that most consumers do not hold a credit card that is particularly well suited to their use patterns. Findings from the Consumer Payments Survey are consistent with consumers exhibiting certain behavioural biases when they first choose their credit card. In particular, I find suggestive evidence of optimism bias; that cardholders systematically underestimate their probability of incurring credit card interest charges. This would help to explain why the majority of consumers who regularly pay interest hold a card with a relatively high interest rate, which increases costs for these individuals.
In addition, I find some evidence that a small but substantial share of cardholders overestimate the net monetary value of their card, and believe they are making a gain when in fact they are likely making a loss. These cardholders are more likely to be motivated by rewards points, and less likely to have paid interest in the past year. This finding is consistent with the hypothesis that, while for respondents who regularly pay interest, the costs of their card may be highly salient, those who do not pay interest may experience bounded rationality; they may overweight benefits, such as rewards and the interest-free period, to form an inflated estimate of their card's value.
In contrast to evidence from the United States, I do not find that temporary credit card sign-up offers appeal to consumers' present bias; consumers who signed up to their card with a temporary offer were no worse off after the offer expired. If anything, these consumers were typically better off than other cardholders. This appears to reflect the mix of offers, with higher-income respondents – who are already more likely to make a net monetary benefit from their card – more likely to respond to, or to be targeted by, these offers in the Australian market.
Finally, I find suggestive evidence of cognitive barriers to switching cards. While I estimate that the vast majority of cardholders would be better off if they held a different card, less than 10 per cent had switched cards over the preceding year. Barriers to switching are likely to be both practical and cognitive, but I identify a group of around half of loss-making respondents who appear most likely to have been influenced by behavioural biases. The data do not allow me to identify the nature of these biases.
My findings represent a first estimate of the presence and impact of behavioural biases in the Australian credit card market. While the survey data do not allow me to rule out other potential explanations for the patterns we see, they provide suggestive evidence that behavioural biases do indeed influence consumer credit card choice in adverse ways. My findings also support earlier conjectures that these biases appear to explain an apparent lack of competition in credit card interest rates.
Future work, using more detailed survey or experimental data, would help to confirm my findings, and would enable a more precise estimate of the impact of these biases. For instance, surveys that collect more detailed information on personal circumstances, card choice and switching behaviour (such as asking respondents if they had experienced any adverse financial shocks since choosing their card, and asking for reasons that respondents do or do not choose to switch cards) would help to confirm (or otherwise) these patterns that I interpret as evidence of behavioural biases. Experimental evidence would allow a cleaner estimate of the effect of such biases.
Appendix A: Monetary and Non-monetary Benefits
This paper calculates the net monetary benefit of respondents' main credit card. As noted in Section 2, the net monetary benefit is not necessarily equivalent to the consumer's total benefit (or consumer surplus) from their card. But the survey evidence suggests they are very closely related.
In the 2016 Consumer Payments Survey, respondents were asked first about the monetary value of their card (weighing up the monetary costs and benefits), and then about the total value of their card (including both monetary and non-monetary costs and benefits). Most respondents gave the same answer to both questions. The share who reported they were better off on net was only slightly higher when respondents were asked to consider both monetary and non-monetary features, than when they considered monetary features alone (Figure A1). This suggests that my estimated net monetary benefit can be considered a close approximation of consumers' overall benefit from their card, and is consistent with the vast majority of respondents having fee-free access to most non-monetary card benefits through a debit card.
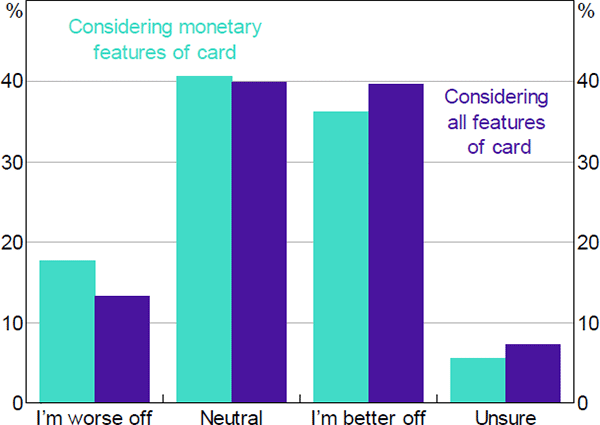
Source: Author's calculations, based on data from Ipsos and RBA
Appendix B: Estimation and Sensitivity Testing of Net Monetary Benefit
B.1 Value of Rewards Points
Just over half of credit card holders accrue rewards points on their main card. For these consumers, I estimate the value of rewards points earned over the past year using the card's rewards points earn rate per dollar spent, multiplied by an estimate of the respondent's total annual spend on their main card, and scaled by a seasonal factor.[49] This yields the estimated value of any applicable rewards program over one year, and is equal to zero for respondents who do not have a rewards program on their main card.
I calculate the earn rate based on the two most common ways that respondents redeem their rewards points: gift cards and flights. According to the survey, gift cards are the most common way that consumers redeem their points, used by just over one-third of rewards card holders (Figure B1). A further one-fifth of rewards card holders redeem their points for flights. For other respondents, who report redeeming their rewards points for cash back or shopping or who had not redeemed their rewards points before, the value of their rewards points is estimated based on the earn rate for a gift card. The value of rewards points redeemed for flights varies with the market price of the flight; my estimate is based on advertised prices around the end of 2016.[50] To test whether this variation affects the overall distribution of monetary benefits, I recalculate my estimates based on all respondents redeeming their rewards points for a gift card; the results do not vary much, especially at the lower end of the distribution (Figure B2).
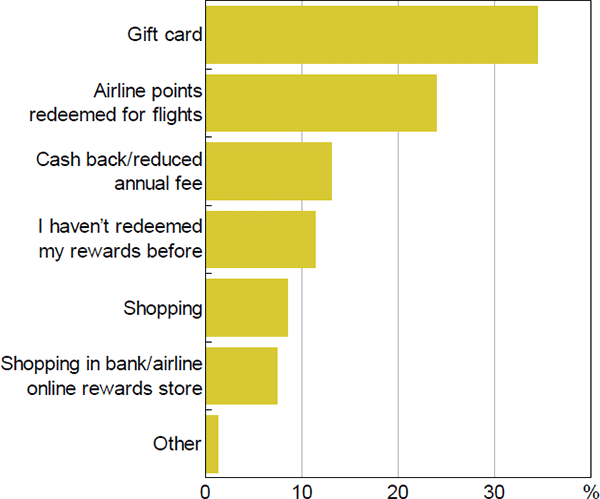
Source: Author's calculations, based on data from Ipsos and RBA
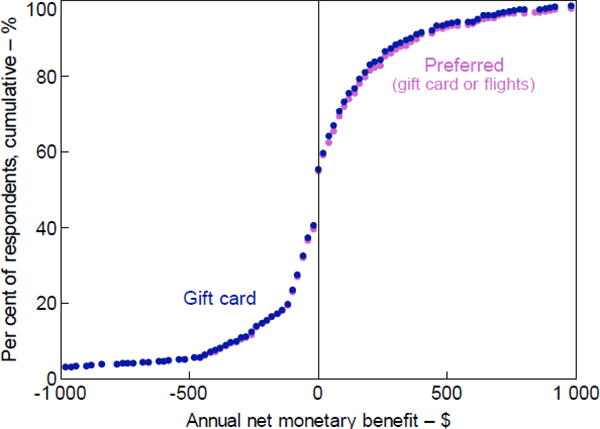
Source: Author's calculations, based on data from Ipsos and RBA
Two measures of annual credit card spending are available. One option is to use the transaction data from the 7-day diary component of the Consumer Payments Survey, by calculating the total value of transactions that respondents make on their main credit card, and scaling this weekly total up for an annual estimate. A second option is to use respondents' self-reported value of spending on their main credit card based on their latest monthly credit card statement.[51] The monthly spend figure yields an estimate with lower variance, but is missing data for 10 per cent of cardholders who selected ‘prefer not to answer’ to this question.[52] In my preferred measure, I use the monthly figure, but results do not vary meaningfully – especially for the group who make a loss from their card – when using the weekly estimate (Figure B3).
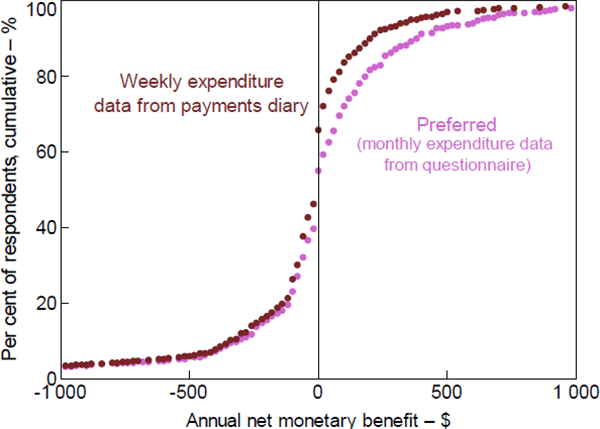
Source: Author's calculations, based on data from Ipsos and RBA
B.2 Annual Fee
The annual fee for each respondent's card is taken from a database of card features. This is combined with information from the Consumer Payments Survey on whether respondents had their most recent annual fee waived or discounted. If the respondent reported paying a discounted fee, I assume a discount of 50 per cent – this is based on the average advertised sign-up discount across a broad range of cards. If the respondent reported that their card does not have a fee, when the card features database suggests that it does, I assume the fee had been waived.
B.3 Value of Interest-free Period
The value of the interest free period is estimated as:
where the alternative interest rate is based on the respondent's answers to a question about whether and how they would borrow money if they did not own a credit card. I use the average mortgage and average personal loan interest rates in November 2016 (from the RBA's statistical table F5 Indicator Lending Rates) for respondents who say they would use their mortgage offset account or take out a personal loan, respectively. For the majority of respondents, who say they would not want to borrow money without a credit card, I use the average savings account interest rate (from RBA statistical table F4 Retail Deposit and Investment Rates). The results do not change substantially when I vary this assumption (Figure B4).
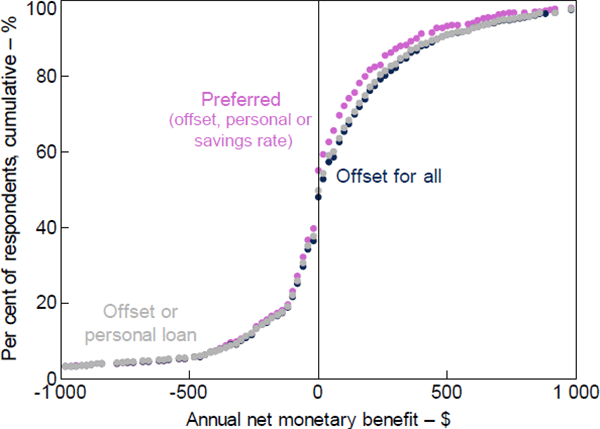
Source: Author's calculations, based on data from Ipsos and RBA
B.4 Interest Charges
For respondents who had paid interest in the past year, I include an estimate of the annual value of those payments. I use information they provided on the amount of interest charges in their most recent monthly statement, scaled by their self-reported frequency of paying interest.[53] For consumers who say that they sometimes or always pay interest, I multiply their interest payments by 3 or 12 months, respectively. For those who say that they do not usually pay interest but did pay interest in their most recent monthly statement, I treat this as a one-off.
For those consumers who do regularly pay interest, this is generally the most important component of their total estimated net monetary benefit. To allow for the possibility of misreporting (for instance, some respondents may have reported their total balance outstanding rather than just the interest charges in the most recent month), I also estimate the net monetary benefit assuming that the most recent interest payments were a one-off for all respondents (Figure B5). This leads to a substantial decrease in the share of respondents making very large losses, but does not affect the share of respondents making a loss at all, which is the main metric presented in this paper.
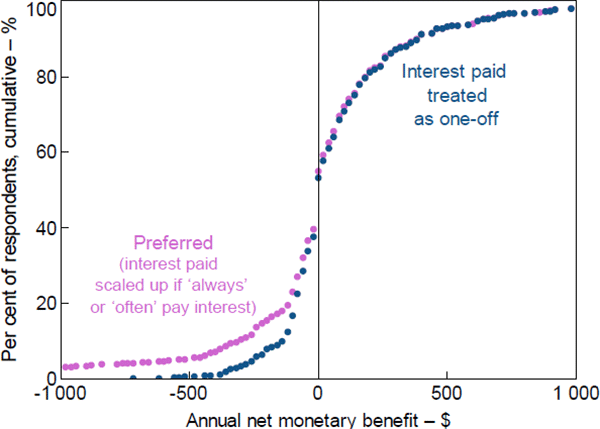
Source: Author's calculations, based on data from Ipsos and RBA
Appendix C: Regression Analysis of Overall Distribution of Net Benefit
The monetary value that consumers receive from their credit card varies substantially with demographic factors, card use patterns and self-reported motivation for having a credit card. To determine which factors are the most important predictors of net monetary benefit, I employ a regression framework. This exercise is largely descriptive; while certain relationships are likely to be causal, others may be endogenous, and my method does not allow me to directly disentangle any causal relationship.
C.1 Overall Net Benefit
I estimate the following model:
Table C1 presents the regression results. Overall, the most important predictors of the value of the net monetary benefit are income, liquid wealth and motivation for holding a credit card. However, the adjusted R-squared is low across all models, suggesting demographics have relatively low explanatory power over the value of the net monetary benefit that respondents receive from their credit card.
Model 1 | Model 2 | Model 3 | Model 4 | |
---|---|---|---|---|
Household income quartile (omitted category = lowest quartile) | ||||
2nd | 31.12 | 4.19 | 25.07 | 22.06 |
(76.27) | (81.52) | (73.36) | (73.61) | |
3rd | 234.30** | 197.14** | 226.20** | 184.66** |
(91.17) | (87.17) | (97.16) | (93.42) | |
4th | 313.73*** | 249.36*** | 276.41*** | 240.21** |
(95.81) | (95.14) | (102.46) | (94.03) | |
Age (years) (omitted category = 18–29) | ||||
30–39 | 35.76 | 37.08 | 28.53 | 10.48 |
(98.07) | (102.17) | (101.94) | (104.57) | |
40–49 | 32.09 | −12.82 | −19.39 | −29.40 |
(62.59) | (64.63) | (63.68) | (69.75) | |
50–64 | 28.45 | 1.30 | 10.82 | 25.20 |
(118.61) | (120.98) | (116.23) | (124.14) | |
65+ | 228.98** | 201.68* | 204.18* | 186.88 |
(107.17) | (117.49) | (120.94) | (129.93) | |
Employment status (omitted category = employed) | ||||
Not employed | −20.94 | 29.31 | 34.24 | 34.27 |
(74.99) | (89.11) | (87.35) | (87.25) | |
Retired | −10.92 | −35.06 | −18.50 | −51.29 |
(94.41) | (99.81) | (105.28) | (101.79) | |
Education (omitted category = did not complete year 12) | ||||
Year 12 | 86.67 | 38.09 | 25.67 | 16.88 |
(81.21) | (79.11) | (75.79) | (79.26) | |
Certificate/diploma | 46.12 | 44.56 | 51.87 | 54.59 |
(68.94) | (71.76) | (71.82) | (72.42) | |
Bachelor or higher | 180.77** | 111.62 | 110.02 | 95.59 |
(89.20) | (94.60) | (89.30) | (92.97) | |
Typical value of bank deposits (omitted category = less than $500) | ||||
$500–1,000 | 216.40 | 229.20 | 270.79 | |
(170.77) | (172.02) | (181.79) | ||
$1,000–7,500 | 300.95* | 304.96* | 292.76* | |
(164.76) | (160.76) | (155.33) | ||
$7,500–30,000 | 395.79** | 398.59** | 363.83** | |
(164.65) | (166.75) | (153.83) | ||
$30,000+ | 564.21*** | 567.22*** | 503.14*** | |
(178.91) | (182.00) | (168.29) | ||
Number of credit cards (omitted category = 1 card) | ||||
2 | 90.83 | 69.24 | ||
(76.34) | (83.19) | |||
3+ | −216.43 | −221.14 | ||
(158.24) | (153.31) | |||
Motivation for holding credit card (omitted category = other) | ||||
Rewards points | 148.40 | |||
(131.39) | ||||
Borrowing | −398.52* | |||
(216.12) | ||||
Payment | 5.85 | |||
(115.17) | ||||
Interest-free period and insurances | 47.73 | |||
(120.49) | ||||
Constant | −351.31*** | −589.75*** | −614.34*** | −542.87*** |
(85.10) | (154.73) | (159.43) | (129.03) | |
Observations | 840 | 816 | 816 | 816 |
Adjusted R2 | 0.01 | 0.03 | 0.04 | 0.06 |
Notes: ***, ** and * represent statistical significance at the 1, 5 and 10 per cent level, respectively; robust standard errors are in parentheses Source: Author's calculations, based on data from Ipsos and RBA |
C.2 Components of Net Monetary Benefit
The above specification is based on the total dollar value of respondents' net benefit. Because the value of interest payments by some consumers is very high, the results are likely to be dominated by the factors that are most important in determining interest payments. But credit cards have two distinct functions: the credit function and the payment function. To more fully understand the predictors of gains and losses, I estimate my model using each of the four components of net monetary benefit as dependent variables. I also show the credit and payment function subtotals as dependent variables.
The variables that are important for each element of the net monetary benefit are somewhat different from the variables that are significant for its overall value (Table C2). Household income is an important predictor of the value that cardholders receive from their rewards points and interest-free period – likely reflecting higher spending rates among higher-income consumers – and of the value of their annual fees, though income is not a significant determinant of the amount of interest charges. Instead, the value of bank deposits had a large negative impact on interest charges, and a small positive impact on the value of benefits, suggesting, as expected, that liquidity constraints are an important determinant of the amount of interest paid.
Value of rewards points | Annual fee | Net payment function (1) – (2) |
Value of interest-free period | Interest paid | Net credit function (4) – (5) |
|
---|---|---|---|---|---|---|
(1) | (2) | (3) | (4) | (5) | (6) | |
Household income quartile (omitted category = lowest quartile) | ||||||
2nd | 2.55 | 2.14 | 6.98 | 5.44 | 11.31 | 12.17 |
(17.32) | (8.64) | (19.65) | (7.37) | (62.76) | (68.21) | |
3rd | 55.38 | 2.73 | 64.12* | 22.85* | −77.98 | 114.80 |
(34.45) | (9.93) | (36.05) | (11.92) | (65.77) | (79.80) | |
4th | 99.25*** | 26.40** | 85.64** | 46.49*** | −65.42 | 150.34* |
(32.78) | (12.60) | (36.60) | (14.77) | (66.41) | (79.53) | |
Age (years) (omitted category = 18–29) | ||||||
30–39 | 68.48** | 0.59 | 78.53** | 41.62*** | 100.22 | −70.59 |
(27.79) | (12.05) | (32.16) | (11.97) | (81.38) | (96.30) | |
40–49 | 46.00* | 17.33 | 34.58 | 30.86** | 96.68* | −64.11 |
(27.09) | (12.69) | (29.36) | (12.80) | (53.72) | (61.16) | |
50–64 | 133.56* | 47.84*** | 103.32 | 63.03** | 163.09*** | −80.88 |
(71.63) | (11.87) | (74.26) | (26.22) | (60.67) | (71.95) | |
65+ | 166.91*** | 54.52*** | 146.72** | 74.38** | 59.07 | 32.30 |
(63.93) | (17.45) | (68.55) | (30.62) | (62.43) | (81.64) | |
Employment status (omitted category = employed) | ||||||
Not employed | −15.34 | −10.17 | −5.61 | −22.88** | −53.79 | 39.24 |
(32.97) | (8.84) | (35.68) | (11.46) | (56.62) | (72.44) | |
Retired | −74.73 | 3.06 | −93.60* | −35.28 | −85.71* | 43.17 |
(51.11) | (14.69) | (56.36) | (24.76) | (50.48) | (63.91) | |
Education (omitted category = did not complete year 12) | ||||||
Year 12 | 95.48*** | 16.34 | 85.06*** | 15.13 | 83.53 | −70.84 |
(27.48) | (12.70) | (28.20) | (9.45) | (70.43) | (71.71) | |
Certificate/diploma | 36.82* | 0.46 | 37.92* | 24.37*** | −10.72 | 12.65 |
(20.00) | (9.36) | (21.92) | (9.13) | (58.34) | (65.02) | |
Bachelor or higher | 69.12* | −1.47 | 71.69* | 22.06 | −26.22 | 19.42 |
(37.20) | (9.82) | (38.55) | (14.90) | (71.38) | (75.52) | |
Typical value of bank deposits (omitted category = less than $500) | ||||||
$500–1,000 | 3.59 | −0.36 | 5.17 | −9.99 | −225.47 | 263.06 |
(21.22) | (12.27) | (26.45) | (15.92) | (144.03) | (180.13) | |
$1,000–7,500 | 24.38 | −11.15 | 32.53 | 7.29 | −203.75 | 254.55* |
(20.42) | (10.91) | (25.14) | (12.12) | (125.93) | (151.53) | |
$7,500–30,3000 | 19.86 | −10.06 | 24.32 | 16.46 | −285.57** | 338.95** |
(23.74) | (12.55) | (28.78) | (14.34) | (124.03) | (149.39) | |
$30,000+ | 113.26* | −7.52 | 119.99* | 41.40* | −291.00** | 378.96*** |
(60.73) | (13.55) | (65.79) | (24.67) | (119.66) | (143.47) | |
Number of credit cards (omitted category = 1 card) | ||||||
2 | 132.62*** | 33.80*** | 92.89* | 36.23** | 78.42* | −29.57 |
(49.77) | (9.55) | (49.97) | (18.35) | (46.92) | (51.08) | |
3+ | 67.48** | 46.66*** | 19.78 | 30.26* | 265.27** | −240.88* |
(34.35) | (12.21) | (35.82) | (17.32) | (132.67) | (145.99) | |
Motivation for holding credit card (omitted category = other) | ||||||
Rewards points | 39.61 | 41.75*** | 8.16 | −31.68 | −139.53*** | 145.65** |
(82.54) | (10.56) | (83.70) | (30.32) | (48.61) | (62.23) | |
Borrowing | −81.00 | 18.41 | −100.88* | −2.35 | 276.25 | −290.51 |
(57.81) | (13.08) | (60.60) | (27.62) | (169.83) | (199.37) | |
Payment | −73.40 | −2.37 | −68.56 | −51.06** | −107.02** | 79.93 |
(68.83) | (7.91) | (69.49) | (25.60) | (46.64) | (59.35) | |
Interest-free period and insurances | −63.25 | 1.38 | −65.20 | −40.01 | −141.54*** | 122.06** |
(71.72) | (9.49) | (72.72) | (27.20) | (46.41) | (61.98) | |
Constant | −105.37*** | 12.33 | −133.13*** | −22.19 | 315.63*** | −402.03*** |
(30.22) | (17.08) | (36.19) | (13.86) | (96.81) | (121.37) | |
Observations | 913 | 952 | 862 | 853 | 936 | 816 |
Adjusted R2 | 0.06 | 0.12 | 0.03 | 0.04 | 0.05 | 0.05 |
Notes: ***, ** and * represent statistical significance at the 1, 5 and 10 per cent level, respectively; robust standard errors are in parentheses Source: Author's calculations, based on data from Ipsos and RBA |
Appendix D: Heckman Sample Selection Models
Motivation only | Motivation plus controls | |
---|---|---|
(1) | (2) | |
Household income quartile (omitted category = lowest quartile) | ||
2nd | −0.02 | |
(0.04) | ||
3rd | −0.01 | |
(0.04) | ||
4th | −0.02 | |
(0.05) | ||
Age (years) (omitted category = 18–29) | ||
30–39 | −0.04 | |
(0.05) | ||
40–49 | −0.07 | |
(0.06) | ||
50–64 | −0.14** | |
(0.06) | ||
65+ | −0.03 | |
(0.06) | ||
Employment status (omitted category = employed) | ||
Not employed | −0.00 | |
(0.05) | ||
Retired | −0.01 | |
(0.05) | ||
Education (omitted category = did not complete year 12) | ||
Year 12 | 0.08 | |
(0.06) | ||
Certificate/diploma | 0.13** | |
(0.06) | ||
Bachelor or higher | 0.16** | |
(0.07) | ||
Typical value of bank deposits (omitted category = less than $500) | ||
$500–1,000 | 0.04 | |
(0.06) | ||
$1,000–7,500 | 0.08 | |
(0.05) | ||
$7,500–30,000 | 0.11** | |
(0.05) | ||
$30,000+ | 0.17*** | |
(0.05) | ||
Motivation for holding credit card (omitted category = other) | ||
Rewards points | 0.37 | 0.06* |
(0.61) | (0.03) | |
Borrowing | 0.11 | 0.05 |
(0.15) | (0.04) | |
Payment | 0.14 | 0.04 |
(0.16) | (0.03) | |
Interest-free period and insurances | 0.24 | 0.04 |
(0.29) | (0.03) | |
Observations | 1,388 | 1,388 |
Notes: Heckman sample selection model controls are: income, age, perceptions of the cost, convenience and usefulness for managing finances of credit cards, typical value of bank deposits, internet access, employment status, and education; ***, ** and * represent statistical significance at the 1, 5 and 10 per cent level, respectively; standard errors are in parentheses Source: Author's calculations, based on data from Ipsos and RBA |
Basic controls | Full controls | Full controls with income × perceived benefit interaction | |
---|---|---|---|
Net monetary benefit (omitted category = neutral) | |||
Gain | −0.03 | −0.08* | −0.07 |
(0.04) | (0.05) | (0.05) | |
Loss | 0.10** | 0.04 | 0.04 |
(0.04) | (0.05) | (0.05) | |
Household income quartile (omitted category = lowest quartile) | |||
2nd | 0.02 | −0.05 | −0.04 |
(0.04) | (0.05) | (0.05) | |
3rd | 0.17*** | 0.07 | 0.08 |
(0.04) | (0.05) | (0.05) | |
4th | 0.13*** | −0.00 | 0.02 |
(0.05) | (0.06) | (0.06) | |
Perceived net benefit (omitted category = neutral) | |||
Better off | −0.04 | −0.04 | |
(0.04) | (0.04) | ||
Worse off | 0.20*** | 0.23*** | |
(0.06) | (0.06) | ||
Education (omitted category = did not finish year 12) | |||
Year 12 | 0.08 | 0.07 | |
(0.06) | (0.06) | ||
Certificate/diploma | 0.09* | 0.08 | |
(0.05) | (0.05) | ||
Bachelor or higher | 0.17*** | 0.17*** | |
(0.05) | (0.05) | ||
Motivation for holding credit card (omitted category = other) | |||
Rewards points | −0.03 | −0.04 | |
(0.06) | (0.06) | ||
Borrowing | −0.01 | −0.01 | |
(0.06) | (0.06) | ||
Payment | −0.12** | −0.12** | |
(0.05) | (0.05) | ||
Interest-free period and insurances | −0.15*** | −0.15*** | |
(0.05) | (0.05) | ||
Credit card interest rate | −0.01 | −0.01 | |
(0.01) | (0.01) | ||
Number of bank branches in home postcode | −0.00 | −0.01 | |
(0.00) | (0.00) | ||
Card type (omitted category = standard) | |||
Low-rate | −0.02 | −0.01 | |
(0.08) | (0.09) | ||
Rewards | 0.09** | 0.10** | |
(0.04) | (0.04) | ||
Observations | 813 | 813 | 813 |
Notes: ***, ** and * represent statistical significance at the 1, 5 and 10 per cent level, respectively; standard errors are in parentheses Source: Author's calculations, based on data from Ipsos and RBA |
Appendix E: Switching Behaviour Regression Output
Basic controls | Full controls(a) | Full controls with income × perceived benefit interaction(a) |
|
---|---|---|---|
Net monetary benefit (omitted category = neutral) | |||
Gain | −0.04 | −0.07 | −0.06 |
(0.04) | (0.04) | (0.04) | |
Loss | 0.12*** | 0.05 | 0.05 |
(0.04) | (0.05) | (0.05) | |
Household income quartile (omitted category = lowest quartile) | |||
2nd | 0.02 | −0.05 | −0.03 |
(0.04) | (0.05) | (0.05) | |
3rd | 0.18*** | 0.08 | 0.08 |
(0.04) | (0.05) | (0.05) | |
4th | 0.12** | 0.01 | (b) |
(0.05) | (0.06) | ||
Perceived net monetary benefit (omitted category = neutral) | |||
Better off | −0.04 | −0.04 | |
(0.04) | (0.04) | ||
Worse off | 0.18*** | 0.21*** | |
(0.06) | (0.06) | ||
Education (omitted category = did not finish year 12) | |||
Year 12 | 0.08 | 0.07 | |
(0.05) | (0.05) | ||
Certificate/diploma | 0.09** | 0.09* | |
(0.05) | (0.05) | ||
Bachelor or higher | 0.18*** | 0.17*** | |
(0.05) | (0.05) | ||
Motivation for holding credit card (omitted category = other) | |||
Rewards points | −0.02 | −0.03 | |
(0.06) | (0.06) | ||
Borrowing | 0.01 | 0.01 | |
(0.06) | (0.06) | ||
Payment | −0.12** | −0.12** | |
(0.05) | (0.05) | ||
Interest-free period and insurances | −0.14*** | −0.14*** | |
(0.05) | (0.05) | ||
Observations | 841 | 816 | 814 |
Notes: ***, ** and * represent statistical significance at the 1, 5 and 10 per cent level, respectively; standard errors are in parentheses Source: Author's calculations, based on data from Ipsos and RBA |
Basic controls | Full controls(a) | |
---|---|---|
Net monetary benefit (omitted category = neutral) | ||
Gain | −0.03 | −0.06** |
(0.02) | (0.03) | |
Loss | 0.02 | −0.03 |
(0.03) | (0.03) | |
Household income quartile (omitted category = lowest quartile) | ||
2nd | 0.03* | 0.02 |
(0.01) | (0.02) | |
3rd | 0.08*** | 0.05** |
(0.02) | (0.02) | |
4th | 0.10*** | 0.06** |
(0.03) | (0.03) | |
Perceived net benefit (omitted category = neutral) | ||
Better off | 0.02 | |
(0.04) | ||
Worse off | −0.02 | |
(0.02) | ||
Education (omitted category = did not finish year 12) | ||
Year 12 | −0.00 | |
(0.03) | ||
Certificate/diploma | 0.02 | |
(0.02) | ||
Bachelor or higher | 0.08*** | |
(0.03) | ||
Motivation for holding credit card (omitted category = other) | ||
Rewards points | −0.04 | |
(0.04) | ||
Borrowing | 0.00 | |
(0.04) | ||
Payment | −0.10*** | |
(0.03) | ||
Interest-free period and insurances | −0.09*** | |
(0.03) | ||
Card type (omitted category = standard) | ||
Low-rate | 0.00 | |
(0.01) | ||
Rewards | 0.02 | |
(0.04) | ||
Observations | 816 | 816 |
Notes: ***, ** and * represent statistical significance at the 1, 5 and 10 per cent level, respectively; standard errors are in parentheses Source: Author's calculations, based on data from Ipsos and RBA |
Appendix F: End of Survey Questionnaire
QUESTION 7: USING YOUR CREDIT CARD(S)
Q7.1. Do any of your credit cards accrue rewards, loyalty points or other benefits?
- ◻ Yes
- ◻ No – please go to question 7.2
Q7.1.1. How do you normally redeem your credit card rewards? (Please tick one box only)
[NOTE] If your points are automatically converted (e.g. into a gift card or airline points), please select the product they are converted into.
- ◻ A gift card
- ◻ Other products/shopping in my bank's rewards store
- ◻ Airline points and redeem them for flights
- ◻ Airline points and redeem them for other products
- ◻ Shopping (online or in-store)
- ◻ Cash back/reduce annual fee
- ◻ I haven't redeemed my rewards before
- ◻ Other, please specify ______________
Q7.2. Have you paid or owed interest on a credit card in the past year?
- ◻ Yes
- ◻ No – please go to question 7.3
- ◻ Don't know – please go to question 7.3
Q7.2.1. Thinking about the last time you had interest outstanding on a monthly credit card bill – what was the main reason? (Please tick one box only)
- ◻ I usually or often pay interest on my card/I am paying off a long-term debt
- ◻ I used my credit card for a cash advance
- ◻ I missed the repayment deadline
- ◻ I accidently spent more than I budgeted for, but I usually don't
- ◻ I decided to spend more than I could pay back, but I usually don't
- ◻ Other, please specify ______________
For the following questions, please answer thinking about your main credit card (the card you most commonly use).
Q7.3. Did an annual fee apply to your main credit card this year? (Please tick one box only)
- ◻ Yes
- ◻ Yes, but I paid a discounted rate
- ◻ No, because it was waived
- ◻ No, because my card has no annual fee
- ◻ Don't know
For the following questions, you may need to refer to your most recent credit card statement for your main credit card.
Q7.4. What is the total amount that you spent on your main credit card in your most recent monthly statement (to the nearest whole dollar)?
AMOUNT: $ ______________
- ◻ I prefer not to answer
Q7.4.1. How much were you charged in credit card interest in your most recent monthly statement?
[NOTE] In most cases, you can find your interest charges either in the summary at the beginning of your statement, or at the end of your list of transactions. Please report the sum of ‘interest charged on purchases’ and ‘interest charged on cash advances’ if they are listed separately on your statement.
AMOUNT: $ ______________
- ◻ None
- ◻ I prefer not to answer
QUESTION 8: CREDIT CARD CHOICE
For all remaining questions, please answer thinking about your main credit card (the card you most commonly use).
Q8.1. Did you sign up to your main credit card with a special offer (e.g. bonus rewards points, annual fee waiver or 0% balance transfer)?
- ◻ Yes
- ◻ No – please go to question 8.2
- ◻ Can't remember – please go to question 8.2
Q8.1.1. Does this offer still apply to your card now?
- ◻ Yes
- ◻ No
- ◻ I'm not sure
Q8.2. Have you considered changing your main credit card in the past year? (Please tick one box only)
- ◻ Yes, I have opened a new credit card and made it my main card
- ◻ Yes, I closed my main credit card and now I usually use one of my other credit cards
- ◻ Yes, but I have not actually switched cards
- ◻ No
Q8.3.1. Thinking about credit cards in general, what are the key features that made you decide to have a credit card at all? (Please tick all boxes that apply)
- ◻ Interest-free period (that is, the time between when you make a purchase and when repayment is due – usually 40–60 days)
- ◻ An easy way to borrow money
- ◻ Reward points or flight points
- ◻ Travel insurance or extended warranty
- ◻ Other benefits (e.g. concierge, entertainment)
- ◻ Ability to shop online
- ◻ Widespread acceptance in Australia
- ◻ Acceptance overseas
- ◻ It helps me smooth spending between pay periods
- ◻ Convenience of not having to check how much money is in my account before making a purchase
- ◻ Fraud protection
- ◻ A credit card is a convenient way of paying
- ◻ Other, please specify ______________
Q8.3.2. Thinking about credit cards in general, what is the most important feature that made you decide to have a credit card at all? (Please tick one box only)
- ◻ Interest-free period (that is, the time between when you make a purchase and when repayment is due – usually 40–60 days)
- ◻ An easy way to borrow money
- ◻ Reward points or flight points
- ◻ Travel insurance or extended warranty
- ◻ Other benefits (e.g. concierge, entertainment)
- ◻ Ability to shop online
- ◻ Widespread acceptance in Australia
- ◻ Acceptance overseas
- ◻ It helps me smooth spending between pay periods
- ◻ Convenience of not having to check how much money is in my account before making a purchase
- ◻ Fraud protection
- ◻ A credit card is a convenient way of paying
- ◻ Other, please specify ______________
Q8.4.1. Thinking about when you first opened your main credt card account, what factors influenced your decision to choose this card over other credit cards? (Please tick all boxes that apply)
- ◻ The fees
- ◻ The interest rate
- ◻ A bonus points/flight/cash back offer
- ◻ A balance transfer deal
- ◻ Reward points or flight points
- ◻ The interest-free period
- ◻ Travel insurance or extended warranty
- ◻ Other benefits (e.g. concierge, entertainment)
- ◻ Card offered by my main/preferred bank
- ◻ Linked to my mortgage/other bank accounts
- ◻ Customer service or quality of the card issuer's website/apps
- ◻ Less costly to use overseas
- ◻ Other, please specify ______________
- ◻ Don't know/can't remember – please go to question 8.5
Q8.4.2. Thinking about when you first opened your main credit card account, what was the most important factor that influenced your decision to choose this card over other credit cards? (Please tick one box only)
- ◻ The fees
- ◻ The interest rate
- ◻ A bonus points/flight/cash back offer
- ◻ A balance transfer deal
- ◻ Reward points or flight points
- ◻ The interest-free period
- ◻ Travel insurance or extended warranty
- ◻ Other benefits (e.g. concierge, entertainment)
- ◻ Card offered by my main/preferred bank
- ◻ Linked to my mortgage/other bank accounts
- ◻ Customer service or quality of the card issuer's website/apps
- ◻ Less costly to use overseas
- ◻ Other, please specify ______________
- ◻ Don't know/can't remember
Q8.5. If you did not have a credit card, would you borrow money in some other way? (Please tick one box only)
- ◻ No, I would not want to
- ◻ I would want to but I don't think I would be able to get a loan
- ◻ I would use money from my offset account or increase my mortgage
- ◻ Yes, I would take out a personal loan
- ◻ Yes, other, please specify ______________
Q8.6. Thinking of all the monetary features of your card (annual fee, rewards, the interest you pay, the interest-free period), overall, how do you see the monetary benefits and costs of owning your card? (Please tick one box only)
- ◻ Neutral – the benefits and costs are roughly equal
- ◻ Overall I'm worse off – I pay more in fees and interest than I receive in benefits
- ◻ Overall I'm better off – I receive more in benefits and rewards than I pay in fees and interest
- ◻ Not sure
Q8.6.1. Now consider both the monetary and non-monetary features of your card (e.g. fraud protection, widespread acceptance, convenience, insurance, concierge services). Overall, how do you see the benefits and costs of owning your card? (Please tick one box only)
- ◻ Neutral – the benefits and costs are roughly equal
- ◻ Overall I'm worse off – I pay more in fees and interest than I receive in benefits
- ◻ Overall I'm better off – I receive more in benefits and rewards than I pay in fees and interest
- ◻ Not sure
References
Arango C, KP Huynh and L Sabetti (2015), ‘Consumer Payment Choice: Merchant Card Acceptance versus Pricing Incentives’, Journal of Banking & Finance, 55, pp 130–141.
ASIC (Australian Securities and Investments Commission) (2015), ‘Senate Standing Committee on Economics Inquiry into Matters Relating to Credit Card Interest Rates: Submission by the Australian Securities and Investments Commission’, Submission to the Senate Economics References Committee Inquiry into Matters Relating to Credit Card Interest Rates, August.
ASIC (2018), ‘Credit Card Lending in Australia’, Report 580, July.
Australian Treasury (issuing body) (2016), Credit Cards: Improving Consumer Outcomes and Enhancing Competition, Consultation Paper, May, Australian Treasury, Parkes.
Ausubel LM (1991), ‘The Failure of Competition in the Credit Card Market’, The American Economic Review, 81(1), pp 50–81.
Brainard L (2017), ‘Where Do Consumers Fit in the Fintech Stack?’, Keynote Address given at ‘FinTech Risks and Opportunities: An Interdisciplinary Approach’, Third Annual Financial Stability Conference Co-hosted by the Office of Financial Research and the University of Michigan's Center on Finance, Law, and Policy, Ann Arbor, 16–17 November.
Calem PS and LJ Mester (1995), ‘Consumer Behavior and the Stickiness of Credit-Card Interest Rates’, The American Economic Review, 85(5), pp 1327–1336.
Carbó-Valverde S and JM Liñares-Zegarra (2011), ‘How Effective are Rewards Programs in Promoting Payment Card Usage? Empirical Evidence’, Journal of Banking & Finance, 35(12), pp 3275–3291.
Chatterjee P and RL Rose (2012), ‘Do Payment Mechanisms Change the Way Consumers Perceive Products?’, Journal of Consumer Research, 38(6), pp 1129–1139.
Ching AT and F Hayashi (2010), ‘Payment Card Rewards Programs and Consumer Payment Choice’, Journal of Banking & Finance, 34(8), pp 1773–1787.
Doyle M-A, C Fisher, E Tellez and A Yadav (2017), ‘How Australians Pay: Evidence from the 2016 Consumer Payments Survey’, RBA Research Discussion Paper No 2017-04.
Fitzpatrick R and G White (2017), ‘Banking Fees in Australia’, RBA Bulletin, June, pp 35–39.
Fraser BW (2011), Banking Services: Cost-Effective Switching Arrangements, Australian Treasury, Parkes.
Friesen L and PE Earl (2015), ‘Multipart Tariffs and Bounded Rationality: An Experimental Analysis of Mobile Phone Plan Choices’, Journal of Economic Behavior & Organization, 116, pp 239–253.
Kahneman D, JL Knetsch and RH Thaler (1991), ‘Anomalies: The Endowment Effect, Loss Aversion, and Status Quo Bias’, Journal of Economic Perspectives, 5(1), pp 193–206.
Kell P (2016), ‘ASIC and Behavioural Economics: Regulating for Real People’, Address given at The Impacts of Behavioural Economics on Financial Markets and Regulations Symposium, Hosted by the Queensland Behavioural Economics (QuBE) Group, Brisbane, 18 October.
Kelly N, T Harpel, A Fontes, C Walters and J Murphy (2017), ‘An Examination of Social Desirability Bias in Measures of College Students' Financial Behavior’, College Student Journal, 51(1), pp 115–128.
Lam T and C Ossolinski (2015), ‘The Value of Payment Instruments: Estimating Willingness to Pay and Consumer Surplus’, RBA Research Discussion Paper No 2015-03.
Mani A, S Mullainathan, E Shafir and J Zhao (2013), ‘Poverty Impedes Cognitive Function’, Science, 341(6149), pp 976–980.
Mullainathan S and E Shafir (2013), Scarcity: Why Having Too Little Means So Much, Times Books, Henry Holt & Company LLC, New York.
Samuelson W and R Zeckhauser (1988), ‘Status Quo Bias in Decision Making’, Journal of Risk and Uncertainty, 1(1), pp 7–59.
Shui H and LM Ausubel (2005), ‘Time Inconsistency in the Credit Card Market’, Unpublished manuscript, University of Maryland, January. Available at <http://www.ausubel.com/creditcard-papers/time-inconsistency-credit-card-market.pdf>.
Simon HA (1978), ‘Rationality as Process and as Product of Thought’, The American Economic Review, 68(2), pp 1–16.
Simon J, K Smith and T West (2010), ‘Price Incentives and Consumer Payment Behaviour’, Journal of Banking & Finance, 34(8), pp 1759–1772.
Thomas M, KK Desai and S Seenivasan (2011), ‘How Credit Card Payments Increase Unhealthy Food Purchases: Visceral Regulation of Vices’, Journal of Consumer Research, 38(1), pp 126–139.
Yang S, L Markoczy and M Qi (2007), ‘Unrealistic Optimism in Consumer Credit Card Adoption’, Journal of Economic Psychology, 28(2), pp 170–185.
Acknowledgements
I would like to thank Michele Bullock, David Emery, Chay Fisher, Gianni La Cava, Ed Tellez, Tony Richards, John Simon and Robert Slonim for their thoughtful feedback. Thank you also to seminar participants at the Reserve Bank of Australia and to others who provided helpful assistance and advice; in particular James Bishop, Dilhan Perera and Anirudh Yadav. This research builds on earlier analysis by Rebecca Fairbrother, Paul Ryan and Hao Wang. In addition, thanks to my colleagues Kimberly Francis, Sean Langcake, Jiamin Lim, Christian Maruthiah and Stephen Mitchell for help collecting some of the data used in this analysis. The views in this paper are my own and do not reflect the views of the Reserve Bank of Australia. Any errors are my own.
Footnotes
This issue has also attracted political attention recently, with the Australian Senate Economics Reference Committee's 2015 Inquiry into Matters Relating to Credit Card Interest Rates, and in discussions during the House of Representatives Standing Committee on Economics' Review of Australia's Four Major Banks. [1]
Note that a negative net monetary benefit does not directly imply that the respondent is ‘irrational’. Credit cards provide a payment service to cardholders; even abstracting from the credit function, we may expect consumers to be willing to pay a fee for this service. In addition, even if cardholders do not use their card to borrow, they may place some value on the option to access liquid funds in case of an emergency. [2]
My calculations are likely to overestimate the net monetary benefits, on average, that credit card holders receive, for reasons detailed in Section 4.3. However, this should not affect my main conclusions, which relate to the cross-sectional distribution of net monetary benefits. [3]
On most cards, consumers may borrow for up to two months interest free; during this period, consumers receive a monetary benefit from the interest-free loan, for instance, by earning interest on their savings or paying down higher-interest debts, rather than paying for their purchases upfront. [4]
In addition, but outside of the scope of this paper, some evidence suggests that using a credit card can lead consumers to increase their total spending. This effect may be driven by an alleviation of liquidity constraints, or by behavioural factors (Thomas, Desai and Seenivasan 2011; Chatterjee and Rose 2012). [5]
Simon et al (2010) explore the possibility of endogeneity, noting that consumers self-select into holding a rewards card, potentially based on unobserved preferences to use credit, and this self-selection may upwardly bias their estimates. They conclude this is less of a concern in their case. [6]
Adding stated preferences to the regression helps to control for potential unobserved factors relating to self-selection into holding a rewards credit card (Ching and Hayashi 2010). [7]
However, our survey data suggest that most respondents place a very small value on non-monetary benefits and are primarily motivated by monetary features when they choose their card (see Appendix A). [8]
Similarly, cardholders may be myopic and fail to consider the possibility of interest payments at all. The effects would be the same. [9]
Some consumers may also sign up expecting to review their choice when the offer expires, but subsequently forget, or delay doing so. [10]
Due to different response rates across demographic categories, survey weights are used so that the final (weighted) dataset aligns with population benchmarks, one of which is credit card ownership. After weighting, 54 per cent of survey respondents held a credit card. [11]
I also exclude 13 respondents who held a credit card with an annual fee above $1,000, as I was unable to quantify the value of certain non-reward points benefits that these cards carry. [12]
For instance, respondents were asked to select their credit cards from a list and, if they could not find their card on the list, to enter the name of their card as free text. I was able to reclassify most free text responses, but 4.5 per cent of cardholders entered free text from which I was unable to identify the particular card. [13]
Overall, the characteristics of respondents with missing data are similar to the sample average, though they tended to be younger and to have lower incomes. They were also a little more likely to report that they regularly pay interest charges, and generally they held less positive attitudes towards credit cards than other respondents. If anything, dropping these respondents is likely to upwardly bias my estimates of the net monetary benefit. Appendix B presents sensitivity tests that calculate the net monetary benefit using alternative variables that are available for more respondents. These changes increase the sample size, but do not meaningfully affect the distribution of net monetary benefits. [14]
See Doyle et al (2017) for analysis of how these preferences differ by age group. [15]
Respondents were able to select from a list of reasons, or enter their own reason under the ‘Other’ option. Of those who selected ‘Other’, the most common reason was that they hold a credit card in case of emergencies. [16]
The monetary value of the interest-free period is the benefit cardholders would receive from maintaining funds in a savings account (to earn interest) or in a mortgage offset account (to reduce their interest liability) rather than paying for their purchases upfront. This value varies across individuals, and would be higher for those who typically spend more on their card. [17]
This preference may itself reflect a behavioural bias. However, most banks offer a range of credit card options, so a preference to remain with a single bank is unlikely to affect the features of the card that respondents choose. [18]
One-quarter of respondents held two cards, and 13 per cent held three or more. The vast majority (three-quarters) of credit card purchases recorded in the 2016 Consumer Payments Survey were made using respondents' main card. [19]
The seasonal factor is derived from the time series of total personal credit card spending in the RBA's monthly retail payment statistics. [20]
The mean net monetary benefit is $2, with a 95 per cent confidence interval ranging from –$66 to $69. The median is $9. [21]
As a rough approximation, if we instead replace the value of the interest-free period to consumers with the overnight interest rate (as a proxy for bank funding costs) and adjust for non-use of rewards points, the average falls to a net loss to cardholders of $40. [22]
Over 10 per cent of rewards card holders stated that they had never redeemed their rewards points before (see Appendix B). [23]
Note that many credit card issuers are not banks. Fees on cards issued by non-banks are not included in the RBA survey of bank fee income, but they are included in the Consumer Payments Survey. [24]
The ‘optimal’ net benefit is calculated based on advertised card features. Note that in the Australian credit card market, cardholders incurring interest generally pay the advertised interest rate. This differs from other markets, such as the United States, where, for the same card product, different individuals may be offered individualised interest rates based on their credit history. [25]
Note also that the potential gain from choosing an ‘optimal’ card is estimated here for the marginal individual. Card issuers would likely adjust their pricing models if a large portion of consumers were to behave in this way. [26]
The estimated ‘optimal’ net benefit makes some simplifying assumptions (for instance, the value of rewards points is based on points required for a $100 gift card, and not on the respondent's actual method of redeeming rewards points). I re-estimate the actual net benefit with these same assumptions to ensure comparability. As a result, the distribution of the actual net monetary benefit in Figure 7 is slightly different from the preferred estimate shown in Figure 3. [27]
28 ASIC (2018) estimated that consumers carrying balances on higher-rate cards could save an average of roughly $340 if they held a lower-rate card (that is, ASIC estimate that around 1.8 million cardholders could save an aggregate of $621.5 million in interest charges). My estimated potential savings for interest payers is close to, but slightly below ASIC's estimate, likely due to methodological differences. One important difference is that ASIC's figures are based on potential interest savings for cardholders carrying balances on cards with an interest rate of 20 per cent or higher, while my estimates relate to all interest-paying cardholders for whom a lower-cost card is available. I estimate a mean saving of $245 (and a median of $145) for respondents who usually or often pay interest. [28]
Though, as is evident from the analysis in Section 4.1 and Appendix A, most survey respondents appear to view monetary features as more important than non-monetary features. [29]
Note that the sample for this regression includes only respondents who hold a credit card. [30]
Because this variable is reported based on activity in the past year, it is not perfectly correlated with the value of interest paid (which is based on the month of the most recent credit card statement). [31]
An alternative explanation, with the same effect, is that respondents are ‘myopic’; that is, it is not that they underestimated their probability of paying interest, but that they failed to consider the probability at all. [32]
An ideal test for whether bounded rationality is important in this context would be to consider whether respondents with more complex cards (e.g. those that have more features, incidental fees, or complex rewards programs) receive lower net monetary benefits than those with similar, but less complex card products. Unfortunately, I do not have a measure of product complexity that is independent of other card features that affect the net monetary benefit. [33]
Note that the perceived monetary benefit is measured as at the time of the survey, not at the time of choosing the card; if, for instance, respondents revise their estimate of the value of their rewards program after using their card for some time, the survey results would incorporate this learning. [34]
Although respondents who broke even or made a net benefit may also overestimate the value of their cards, I am unable to observe this based on the categorical measure of perceived gain or loss. [35]
The Heckman sample selection model re-estimates the model in column (2), but accounts for selection into the loss-making cardholder group based on observable factors. [36]
The actual share of respondents with expired sign-up offers is likely to be larger than 10 per cent, and include some of the 17 per cent of respondents who could not remember whether a sign-up offer had applied to their card. [37]
These long-term costs may include the time cost of switching cards, if consumers intend to choose a different card after their offer expires, but fail to follow through. Relatedly, but outside of the scope of this paper, the sign-up offer may lead beneficiaries to form habits that persist after the offer expires. For instance, those with a temporary low interest rate may develop spending patterns based on that temporary low cost of debt. [38]
More precisely, a forward-looking consumer may compare the time-discounted costs and benefits of a card with a sign-up offer to a card without an offer, and choose the card with the highest net present value. If costs increased substantially after the offer expired, we would expect forward-looking consumers to switch cards when the offer expires such that we would not observe them with expired offers. [39]
The value of the offer itself would not be captured in the net benefit calculation unless it was a discounted annual fee. [40]
If instead of respondents' self-reported sign-up behaviour, I use an indicator of whether respondents listed ‘bonus points, flights or a cash back offer’ as an important reason for choosing their card (from Figure 2), I also find no significant effect. [41]
Australian regulations prohibit card issuers from sending consumers unsolicited pre-approved offers of credit or credit limit increases. [42]
Income and liquid wealth are controlled for in the regressions in Table 7. Findings in Section 6.2 suggest that some highly-educated consumers believe they are making a net gain from their card when in fact they are making a loss. But the value of these losses is modest as these consumers are less likely to incur interest charges. As discussed in Section 5.1, most highly-educated respondents received a net benefit from their card. These findings are therefore consistent with banks potentially using credit card sign-up offers as a ‘loss leader’ to attract customers to other financial products. [43]
Similar observations have been made about bank account switching more broadly. For a recent discussion of account switching arrangements and the potential barriers to switching in Australia, see Fraser (2011). [44]
These costs may include searching for and identifying a new card, applying for the card (for which some consumers may need to provide documentation or visit a branch), switching any direct debits or other automatic payments to the new card, and closing the previous card's account. [45]
Some of these respondents may have considered switching cards, but not followed through for ‘behavioural’, not practical, reasons – potentially due to cognitive scarcity, present bias or status quo bias. I am unable to separate out these reasons in the survey data. [46]
These observations are based on a probit model of the probability of considering switching on a range of demographic factors (see Appendix E). The unconditional effect of income is significant and large, but this effect declines in both significance and magnitude after adding controls. Results are similar when the probability of considering switching is modelled as a two-stage process (accounting for self-selection into holding a credit card using a Heckman sample selection model, see Table D2). This finding suggests that the relationship between switching behaviour and income may be due to both observed and unobserved characteristics of high-income cardholders. [47]
This finding relates to the relationship between income and switching behaviour within the sample of credit card holders. In this regression I do not use the Heckman selection model mentioned above. [48]
I use the October 2016 seasonal factor from the aggregate consumer credit card payment series in the RBA's monthly retail payment statistics, generated using X-12 seasonal adjustment. [49]
The value of rewards points redeemed for flights is naturally variable, but I estimate it based on the sale fare value of rewards points redeemed on 13 common domestic and international routes. [50]
Respondents were asked ‘What is the total amount you spent on your main credit card in your most recent monthly statement?’. [51]
Note that it is the difference in estimated spending for respondents for whom both weekly and monthly expenditure measures are available that drives the differences shown in Figure B3 – not the addition of the approximately 80 respondents for whom monthly spending data was not provided. [52]
Respondents were asked ‘How much were you charged in credit card interest in your most recent monthly statement?’ to capture new interest charges, rather than existing interest balances. [53]