RDP 2023-07: Identification and Inference under Narrative Restrictions 2. Bivariate Example
October 2023
- Download the Paper 1.10MB
We illustrate the econometric issues that arise when imposing NR in the context of the following bivariate SVAR(0): , for t = 1,…,T, where yt = (y1t, y2t)′ and , with . We abstract from dynamics for ease of exposition, but this is without loss of generality. The orthogonal reduced form of the model reparameterises A0 as , where is the lower-triangular Cholesky factor (with positive diagonal elements) of . We parameterise as
and denote the vector of reduced-form parameters as . Q is an orthonormal matrix in the space of 2×2 orthonormal matrices, (2):
where . This formulation of the model, which follows Baumeister and Hamilton (2015), means that the structural parameters can be expressed as functions of the reduced-form parameters and . Restrictions on the structural parameters and/or functions of the structural shocks can then be interpreted as restricting to some set. In what follows, we discuss properties of this set that are key for analysing identification and inference under NR.
2.1 Shock-sign restrictions
Consider the ‘shock-sign restriction’ that is non-negative for some :
Equation (3) implies that the restricted structural shock can be written as a function . Along with the ‘sign normalisation’ , the shock-sign restriction implies that is restricted to the set
The restriction induces a set-valued mapping from to that depends on the realisation of yk. Giacomini et al (2022a) characterise this mapping in the case where . For example, if , then
where . The direct dependence of this mapping on the realisation of the data implies that the standard notion of an identified set – the set of observationally equivalent structural parameters given the reduced-form parameters – does not apply. Consequently, it is not obvious whether the restrictions are, in fact, set identifying in a formal frequentist sense, nor whether existing frequentist procedures for conducting inference in set-identified models are valid. We analyse identification under these restrictions in Section 4.
When conducting Bayesian inference, AR18 construct the posterior using the conditional likelihood – the likelihood of observing the data conditional on the NR holding. Letting , the conditional likelihood is
The denominator in the first term – the ex ante probability that the NR is satisfied – equals ½, because is standard normal. The conditional likelihood therefore depends on only through the indicator function , which truncates the likelihood, with the truncation points depending on yk. To illustrate, the left panel of Figure 1 plots the conditional likelihood as a function of given two realisations of a data-generating process and fixing to its true value.[5] The conditional likelihood is flat over the interval for satisfying the shock-sign restriction and is zero outside this interval. The support of the non-zero region depends on yk.
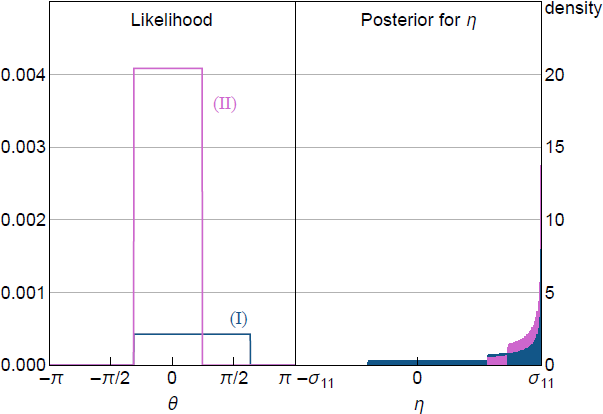
Notes: is known and is the narrative restriction. (I) corresponds to , (II) corresponds to and . Posterior for approximated using 1,000,000 draws of from uniform posterior.
The flat likelihood implies that the posterior for is proportional to the prior in the region where the likelihood is non-zero, and is zero outside this region. The standard approach to Bayesian inference in SVARs under sign restrictions assumes a uniform prior over Q, as do AR18.[6] In the bivariate example, this is equivalent to a prior for that is uniform (Baumeister and Hamilton 2015). This prior implies that the posterior for is also uniform over the interval for where the likelihood is non-zero.
The impact impulse response of y1t to a positive standard deviation shock is . The right panel of Figure 1 plots the posterior for induced by a uniform prior over given the same realisations of the data for which the likelihood was plotted in the left panel. It can be seen that the posterior for assigns more probability mass to more-extreme values of . This highlights that even a uniform prior may be informative for parameters of interest, which also occurs under traditional sign restrictions (Baumeister and Hamilton 2015). One difference is that the prior under sign restrictions is never updated by the data, whereas the support and shape of the posterior for under NR may depend on the realisation of yk through its effect on the truncation points of the likelihood, so there may be some updating of the prior. However, the prior is not updated at values of corresponding to the flat region of the likelihood. Posterior inference about may therefore still be sensitive to the choice of prior, as in standard set-identified SVARs.
2.2 Historical decomposition restrictions
The historical decomposition is the contribution of a particular structural shock to the observed unexpected change in a particular variable over some horizon. The contribution of the first shock to the change in the first variable in the kth period is
while the contribution of the second shock is
Consider the restriction that the first structural shock in period k was positive and (in the language of AR18) the ‘most important contributor’ to the change in the first variable, which requires that . Under these restrictions, must satisfy a set of inequalities that depends on and yk. As in the case of the shock-sign restriction, this set of inequalities generates a set-valued mapping from to that depends on yk.
Let represent the indicator function equal to one when the NR are satisfied and equal to zero otherwise, and let denote the indicator function for the same event in terms of the structural shocks rather than the data. The conditional likelihood given the restrictions is then
In contrast to the case of shock-sign restrictions, the probability in the denominator now depends on through the historical decomposition. Intuitively, changing changes the impulse responses of y1t to the two shocks and thus changes the ex ante probability that . Consequently, the likelihood is not necessarily flat when it is non-zero.
To illustrate, the top panel of Figure 2 plots the conditional likelihood under the historical decomposition NR using the same data-generating process as in Figure 1. The bottom panel plots the probability in the denominator of the conditional likelihood. The likelihood is again truncated, but it is no longer flat – it has a maximum at the value of that minimises the ex ante probability that the NR are satisfied (within the set of values of that are consistent with the restriction given the realisation of the data). The posterior for induced by the usual uniform prior will therefore assign greater posterior probability to values of that yield a lower ex ante probability of satisfying the NR.
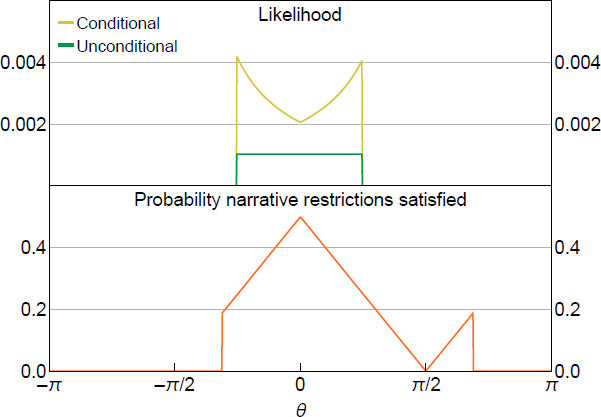
Notes: is known, and and are the narrative restrictions. is approximated using 1,000,000 Monte Carlo draws.
If we view the narrative event () as observable and its probability of occurring depends on the parameter of interest, then conditioning on the narrative event implies that we are conditioning on a non-ancillary statistic. This is undesirable when conducting likelihood-based inference, because it represents a loss of information about the parameter of interest. Unlike for the shock-sign restriction, the probability that the historical decomposition restriction is satisfied depends on , so the event that the NR are satisfied is not ancillary. Conditioning on this event means that the shape of the likelihood (within the non-zero region) is fully driven by the inverse probability of the conditioning event.
Based on this consideration, we therefore advocate constructing the posterior using the joint (or unconditional) likelihood of observing the data and the NR holding:
For all types of NR, the unconditional likelihood is flat with respect to (when it is non-zero) and depends on only through the points of truncation. Of course, this means that posterior inference based on the unconditional likelihood may be sensitive to the choice of prior, as when using the conditional likelihood under shock-sign restrictions. In Section 5.2, we propose how to deal with this posterior sensitivity.
Footnotes
The data-generating process assumes vec(A0) = (1,0.2,0.5,1.2)′, which implies that and with Q equal to the rotation matrix. We assume the time series is of length T = 3 and draw sequences of structural shocks such that T is a small number to control Monte Carlo sampling error. The analysis with set to its true value replicates the situation with a large sample, where the likelihood for concentrates at the truth. It also facilitates visualising the likelihood, which otherwise is a function of four parameters. [5]
See, for example, Uhlig (2005), Rubio-Ramírez, Waggoner and Zha (2010) and Arias, Rubio-Ramírez and Waggoner (2018). [6]