RDP 2024-09: The ‘Clean Energy Transition’ and the Cost of Job Displacement in Energy-intensive Industries 2. Data
December 2024
- Download the Paper 1.64MB
In this paper, as well as in OECD (2024[1]), we utilise matched employer-employee data for a total of 14 OECD countries (Australia, Austria, Canada, Denmark, Estonia, Finland, Germany, Hungary, the Netherlands, Norway, Portugal, Spain, France, Sweden). This section defines energy-intensive industries, explains the harmonisation of matched employer-employee data across countries and introduces the empirical strategy for measuring and explaining the costs of displacement between sectors and countries. As such, it provides additional background to the less detailed exposition of the methodology in OECD (2024[1]).
Defining energy-intensive industries
For comparability purposes, we use a uniform definition of energy-intensive industries across countries.[4] We rely on Environmental Accounts data from the World Input-Output Database, covering 27 EU countries, the United Kingdom, Norway, Iceland and Switzerland, for the period 2000-2014. The Environmental Accounts include information on gross energy use in terajoules (TJ) and value added across NACE 2-digit industries (European Commission JRC, 2019[3]). This allows us to define the energy intensity of a sector in terms of energy use over value added and to differentiate between sectors with high-energy intensity and lower energy intensity.
In practice, we define energy-intensive industries as industries appearing in the top two deciles of the energy-intensity distribution across 2-digit NACE industries in at least 15 countries (Figure 1).[5] The definition of energy-intensive sectors comprises sectors with particularly high energy intensity, averaging about 181 terajoule (TJ) per million EUR of gross value added (in constant 2015 prices of the national currency), from sectors with lower energy intensity. The manufacturing of coke and refined petroleum products stands out in terms energy-intensity, with more than 1200 TJ per million EUR of gross value added, significantly driving up the average energy-intensity of energy-intensive industries. Without this sector, energy-intensive industries would average 64 TJ per million EUR of gross value added. Importantly, the definition of energy-intensive industries coincides with that of carbon-intensive sectors, as used in Barreto, Kril and Grundke (2023[4]) and with that of GHG-intensive sectors used in OECD (2024[1]).[6]
To provide more insight on the cost of job displacement across energy-intensive industries, we divide them into three separate sectors:
- Energy supply (“E”). This includes three sectors: i) electricity, gas and air conditioning; ii) coke and refined petroleum; iii) mining and quarrying. The NACE-2 digit level classification is not sufficiently granular to single out renewable energy generation which is expected to expand from fossil fuel energy generation (see e.g. Borgonovi et al. (2023[2])).
- Energy-intensive manufacturing sectors (“heavy manufacturing” or “M”). This includes many sectors commonly defined as “heavy manufacturing” (or sometimes “hard-to-abate”) such as manufacturing of basic metals, chemicals, pulp and paper, and non-metallic mineral products. We refer to this as “heavy manufacturing” (I).
- Energy-intensive service sectors (“transport” or “T”). This includes the part of services related to transport whether by air, sea or land transport. We will therefore refer to energy-intensive services as transport (T). Note that NACE classification does not allow excluding transport based on renewable energy sources such as electrified land transport.
Given the very different nature of these sectors, they are likely to be impacted differently by the clean-energy-transition. For example, energy supply is directly impacted by structural changes in the energy mix of many OECD countries, with a shift from fossil-fuel-based energy-generation to renewables. Heavy manufacturing is indirectly affected by the clean-energy-transition, as it faces changes in energy costs as well as regulatory requirements. The transport sector is affected through technological and infrastructure changes, but also faces increased energy costs as well as regulatory requirements. As such, a sectoral differentiation of the analysis in OECD (2024[1]) may shed additional light on the labour market effects of the clean-energy transition.[7]
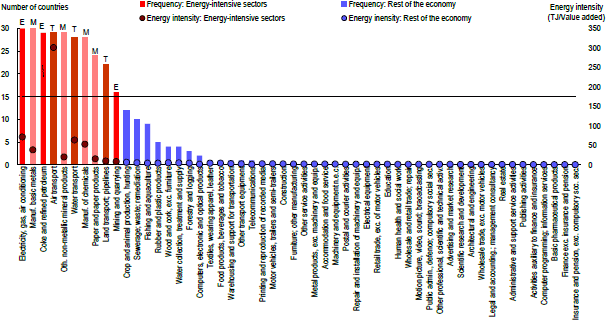
Note: The figure shows on the left axis the frequency with which a particular industry is ranked among the top 20% of most energy-intensive industries across European countries as well as on the right axis the average energy use across countries expressed in TJ per unit of value added. E refers to energy supply sectors, M refers to heavy manufacturing sectors and T refers to transport sectors.
Source: World Input-Output Database Environmental Accounts
Data sources and harmonisation
For the analysis of job displacement in energy-intensive industries, we make use of harmonised linked employer-employee data for 14 OECD countries: Australia, Austria, Canada, Denmark, Estonia, Finland, France, Germany, Hungary, the Netherlands, Norway, Portugal, Spain and Sweden (see Table 1). These data are derived from administrative records designed for tax or social security purposes or, in a few cases, mandatory employer surveys. Each of these datasets are panels, cover the universe of workers or a large random sample of workers, and contain identifiers for their employers and industries. Each of these features is indispensable to the analysis of job displacement within detailed industries. The resulting dataset typically covers the years 2000 to 2019, and thus avoids potential confounding effects resulting from the COVID-19 pandemic and subsequent energy-cost inflation. For some of the countries, the observation period begins later than 2000 or ends before 2019.
Country | Name | Earnings data source | Sample structure | Time coverage |
---|---|---|---|---|
Australia | Person Level Integrated Data Asset (PLIDA) | Tax administration | 10 % random sample of workers | 2002-2019 |
Austria | AMS-BMASK Arbeitsmarktdatenbank | Social security administration | Universe | 2000-2019 |
Canada | Canadian Employer-Employee Dynamics Database | Tax administration | Universe | 2001-2019 |
Denmark | Integrerede Database for Arbejdsmarkedsforskning (IDA) and other data from Statistics Denmark | Tax administration | Universe | 2000-2019 |
Estonia | Data from the Tax and Customs Board Register | Tax administration | Universe | 2003-2019 |
Finland | FOLK employment data from Statistics Finland, Employer Payroll Report from Tax Admin. | Tax administration | Universe | 2000-2019 |
France | Panel DADS | Social security administration | 1/12 % random sample of workers | 2002-2019 |
Germany | Integrierte Erwerbsbiographien (IEB) | Social security administration | 10% random sample of workers | 2000-2019 |
Hungary | ADMIN –2 – Panel of linked administrative data (OEP, ONYF, NAV, NMH, OH) | Social security administration | 50% random sample of workers | 2003-2017 |
Netherlands | CBS Microdata from Statistics Netherlands | Tax administration | Universe | 2006-2019 |
Norway | Arbeidsgiver- og arbeidstakerregister (Aa-registeret), Lønns- og trekkoppgaveregisteret (LTO) | Tax administration | Universe | 2002-2019 |
Portugal | Quadros de Pessoal | Mandatory employer survey | Universe | 2002-2019 |
Spain | Muestra Continua de Vidas Laborales con Datos Fiscales (MCVL-CDF) | Social security and tax administration | 4% random sample of workers | 2006-2019 |
Sweden | Longitudinell integrationsdatabas för sjukförsäkrings- och arbetsmarknadsstudier (LISA), Företagens ekonomi (FEK), Jobbregistret (JOBB) | Social security administration | Universe | 2002-2018 |
To ensure comparability across the 14 linked employer-employee panels, we standardize the data using a common data preparation procedure for each country. For each of the datasets, we select the primary employer, which is defined as the employer from which an employee receives the highest earnings in a given year.[8] Whenever possible, employers are identified based on establishment identifiers rather than those of the firm which may comprise several establishments, potentially operating in different industries and regions. In countries where there are no establishment identifiers are available, firm identifiers are used instead.[9] Worker tenure at these employers is measured as the duration of employment with the current employer in years.
Earnings are defined broadly, encompassing regular wages, overtime, and bonuses, all before taxes and social security deductions.[10] In countries where earnings are censored above a certain ceiling (e.g. Germany), earnings are imputed following the procedure proposed by Card, Heining and Kline (2013[5]). Based on the earnings information, we compute a set of harmonized outcomes. Annual earnings are calculated as the total labour income for the respective year, potentially from multiple employers beyond the primary employment spell. Daily wages are derived by dividing annual earnings from the main employer by the number of days worked for the main employer that year, excluding marginal jobs with very low earnings.[11] Following the standard practice in the job displacement literature, we assign zero earnings and missing daily wages during periods of non-employment spells, that is, years without recorded earnings from any employer.
Finally, we apply common sample restrictions across all datasets. Our baseline analysis focuses on workers aged 18-50 years in the private sector, excluding apprentices and self-employed individuals While we do not consider mass-layoff events in the public administration and defence sectors, we retain employment spells in the final datasets to avoid overstating earnings losses when workers transition to the public sector after displacement (see the methodology section for a detailed definition of the mass layoff events).
Descriptive statistics
The average number of workers included in the final datasets is large in all countries and sectors, and its absolute number depends on the data structure (i.e. whether the source dataset is the universe or a random sample) (Table 2). The energy-intensive sector accounts about 9% of total employment in the included countries. Workers in this sector tend to predominantly be male, slightly older on average, and often boast longer tenures compared to workers in other sectors. Average length of tenure is especially high in energy supply and heavy manufacturing, while in transport it is not too different from the rest of the economy. Workers in energy supply and heavy manufacturing are also more frequently employed by high-paying firms. While employment in energy supply and heavy manufacturing has been on a declining trend, employment in the rest of the economy[12] and, in some countries also in the transport sector, has been growing.
As evident in Table 2, the number of workers displaced in mass layoff events – which we define further below – varies considerably across sectors. Among other factors, this is the result of differences in sample size and employment shares as well as country- and sector-level employment trends. Notably, the number of displaced workers tends to be smallest in energy supply. For this reason, estimates of job displacement in energy supply sectors should be interpreted with caution, especially for France and Spain. In practice, we exclude country-sector combinations with fewer than 100 individual layoffs and 10 firms experiencing mass layoffs over the analysis period.
Country | Average number of workers | Age | Share of women | Tenure (years) | Firm size | Log daily wage | AKM Firm FE | Number of displaced workers | Number of mass-layoff firms | Average share of sector in total employment | Employment change (first year – last year) |
---|---|---|---|---|---|---|---|---|---|---|---|
Panel A. Energy supply | |||||||||||
AUS | 15,405 | 38.69 | 19.83 | 3.42 | 441 | 5.58 | 0.40 | 776 | 367 | 2.43 | 35.62 |
AUT | 31,713 | 41.67 | 16.96 | 10.44 | 566 | 4.79 | 0.12 | 530 | 17 | 0.98 | 1.44 |
CAN | 321,768 | 41.17 | 22.15 | 7.68 | 4,449 | 11.23 | 0.41 | 10,345 | 40 | 3.22 | 20.87 |
DEU | 32,941 | 43.53 | 20.15 | 9.98 | 794 | 4.95 | 0.32 | 107 | 181 | 1.17 | −25.62 |
DNK | 12,556 | 45.00 | 22.78 | 5.92 | 115 | 7.08 | 0.15 | 33 | 18 | 0.60 | 2.07 |
ESP | 2,797 | 44.07 | 15.01 | 6.98 | 329 | 4.47 | 0.61 | 72 | 85 | 0.43 | −21.26 |
EST | 12,848 | 45.86 | 24.04 | 3.83 | 1,225 | 9.23 | 0.32 | 40 | 6 | 2.28 | −35.75 |
FIN | 10,530 | 43.11 | 19.55 | 7.33 | 1,395 | 4.72 | 0.09 | 396 | 44 | 0.98 | −17.81 |
FRA | 16,893 | 42.43 | 23.35 | 8.65 | 350 | 4.80 | 0.17 | 41 | 61 | 0.97 | −22.73 |
HUN | 17,239 | 43.32 | 24.40 | 5.43 | 1,666 | 9.35 | 0.55 | 339 | 18 | 1.05 | −27.19 |
NLD | 38,604 | 43.98 | 20.45 | 3.30 | 1,674 | 4.96 | 0.31 | 10 | 11 | 0.55 | 26.24 |
NOR | 65,377 | 43.25 | 19.76 | 5.51 | 5,128 | 7.54 | 0.43 | 1,043 | 14 | 2.68 | 57.53 |
PRT | 19,726 | 44.13 | 13.59 | 14.97 | 1,239 | 3.89 | 0.36 | 169 | 1 | 0.83 | −15.78 |
SWE | 36,275 | 44.91 | 22.80 | 7.17 | 949 | 7.05 | 0.10 | 225 | 20 | 1.00 | 22.20 |
Average | 45,334 | 43.22 | 20.35 | 7.19 | 1,451 | 6.40 | 0.31 | 1,009 | 63 | 1.37 | −0.01 |
Panel B. Heavy manufacturing | |||||||||||
AUS | 9,927 | 39.6 | 22.2 | 4.0 | 393 | 5.2 | 0.15 | 335 | 1,128 | 1.57 | −36.94 |
AUT | 106,058 | 39.5 | 18.9 | 7.3 | 536 | 4.7 | 0.20 | 3,047 | 194 | 3.27 | 2.95 |
CAN | 263,721 | 43.5 | 19.3 | 8.6 | 2,252 | 10.9 | 0.20 | 15,500 | 480 | 2.64 | −35.77 |
DEU | 94,626 | 43.0 | 19.6 | 11.0 | 2,067 | 4.7 | 0.23 | 955 | 2,327 | 3.37 | −15.55 |
DNK | 34,408 | 44.2 | 24.5 | 7.1 | 140 | 6.9 | 0.11 | 2,012 | 138 | 1.64 | −33.99 |
ESP | 12,751 | 43.0 | 18.0 | 7.2 | 355 | 4.2 | 0.36 | 501 | 932 | 1.94 | −25.81 |
EST | 8,372 | 41.8 | 30.0 | 3.7 | 230 | 9.1 | 0.20 | 363 | 25 | 1.48 | 14.26 |
FIN | 28,909 | 42.3 | 29.4 | 7.5 | 474 | 4.7 | 0.08 | 1,153 | 89 | 2.69 | −27.92 |
FRA | 37,400 | 42.5 | 24.7 | 7.6 | 434 | 4.6 | 0.17 | 836 | 715 | 2.14 | −38.20 |
HUN | 39,246 | 40.9 | 31.1 | 4.7 | 837 | 8.9 | 0.25 | 2,515 | 107 | 2.40 | −5.44 |
NLD | 106,617 | 45.3 | 15.2 | 4.3 | 1,172 | 4.7 | 0.19 | 2,461 | 123 | 1.52 | −12.16 |
NOR | 38,487 | 43.2 | 19.0 | 7.6 | 1,802 | 7.1 | 0.23 | 4,209 | 142 | 1.58 | −38.13 |
PRT | 66,232 | 41.6 | 26.6 | 11.6 | 208 | 3.5 | 0.11 | 2,613 | 139 | 2.78 | −14.98 |
SWE | 102,541 | 43.9 | 20.8 | 8.8 | 1,069 | 6.9 | 0.09 | 2,431 | 143 | 2.82 | −18.33 |
Average | 67,807 | 42.47 | 22.81 | 7.21 | 855 | 6.15 | 0.18 | 2,781 | 477 | 2.27 | −20.43 |
Panel C. Transport | |||||||||||
AUS | 17,833 | 40.46 | 24.36 | 3.31 | 372 | 5.06 | 0.09 | 282 | 2,454 | 2.82 | −9.23 |
AUT | 109,124 | 39.95 | 18.61 | 4.64 | 284 | 4.30 | −0.07 | 2,940 | 473 | 3.36 | 38.15 |
CAN | 359,332 | 43.80 | 24.19 | 7.40 | 4,509 | 10.61 | 0.01 | 6,275 | 650 | 3.59 | 29.44 |
DEU | 60,875 | 43.43 | 19.28 | 6.97 | 1,219 | 4.31 | −0.09 | 418 | 1,331 | 2.17 | 35.13 |
DNK | 74,950 | 43.81 | 18.87 | 4.03 | 59 | 6.82 | 0.05 | 1,910 | 278 | 3.58 | −12.45 |
ESP | 18,637 | 44.54 | 13.04 | 6.99 | 721 | 3.96 | 0.11 | 378 | 803 | 2.83 | −0.51 |
EST | 22,273 | 44.88 | 18.36 | 2.87 | 243 | 8.73 | −0.11 | 312 | 37 | 3.95 | 19.64 |
FIN | 86,761 | 40.88 | 28.47 | 6.81 | 318 | 4.72 | 0.04 | 1,229 | 149 | 8.09 | −18.10 |
FRA | 61,343 | 41.45 | 18.98 | 5.91 | 933 | 4.43 | 0.06 | 729 | 1,320 | 3.52 | 37.39 |
HUN | 33,437 | 41.19 | 16.25 | 3.35 | 802 | 8.45 | −0.12 | 502 | 81 | 2.04 | 142.14 |
NLD | 214,825 | 44.50 | 19.62 | 3.29 | 3,361 | 4.49 | 0.07 | 2,360 | 320 | 3.06 | −2.96 |
NOR | 91,982 | 43.30 | 15.85 | 4.45 | 4,892 | 6.88 | 0.04 | 1,943 | 217 | 3.77 | −8.96 |
PRT | 85,156 | 41.72 | 13.42 | 8.16 | 937 | 3.45 | 0.07 | 2,241 | 171 | 3.58 | 37.11 |
SWE | 122,923 | 44.53 | 15.57 | 5.53 | 1,052 | 6.78 | −0.01 | 1,956 | 241 | 3.38 | 18.49 |
Average | 97,104 | 42.75 | 18.92 | 5.27 | 1,407 | 5.93 | 0.01 | 1,677 | 609 | 3.55 | 21.81 |
Panel D. Rest of economy | |||||||||||
AUS | 579,944 | 36.83 | 49.86 | 3.15 | 372 | 4.86 | −0.01 | 14,108 | 82,527 | 91.58 | 20.54 |
AUT | 2,973,153 | 38.18 | 48.14 | 5.41 | 379 | 4.34 | 0.00 | 79,992 | 10,104 | 91.65 | 38.51 |
CAN | 8,915,632 | 40.55 | 50.35 | 7.63 | 3,844 | 10.49 | −0.03 | 244,620 | 21,830 | 89.13 | 31.59 |
DEU | 2,600,800 | 41.39 | 47.05 | 7.59 | 819 | 4.41 | −0.01 | 26,034 | 78,629 | 92.52 | 19.47 |
DNK | 1,945,936 | 42.10 | 50.09 | 4.66 | 56 | 6.73 | 0.00 | 51,187 | 5,704 | 92.95 | 15.40 |
ESP | 579,140 | 41.88 | 46.45 | 5.45 | 555 | 3.86 | 0.02 | 7,907 | 31,695 | 88.02 | 6.25 |
EST | 508,767 | 41.44 | 54.03 | 2.98 | 641 | 8.84 | −0.01 | 8,419 | 1,059 | 90.16 | 8.40 |
FIN | 942,091 | 39.96 | 44.54 | 5.74 | 1,781 | 4.57 | −0.01 | 22,653 | 3,275 | 87.79 | 42.59 |
FRA | 1,611,195 | 39.51 | 48.76 | 4.91 | 596 | 4.34 | −0.01 | 17,930 | 33,628 | 92.39 | 40.82 |
HUN | 1,506,675 | 40.02 | 51.53 | 3.68 | 7,768 | 8.64 | 0.01 | 30,349 | 3,648 | 92.04 | 15.63 |
NLD | 6,598,902 | 40.95 | 47.52 | 3.18 | 7,496 | 4.32 | −0.01 | 79,300 | 9,595 | 93.86 | 10.76 |
NOR | 2,187,498 | 40.73 | 49.85 | 4.70 | 9,844 | 6.76 | −0.02 | 60,289 | 7,753 | 89.55 | 26.75 |
PRT | 2,170,234 | 38.85 | 47.52 | 7.13 | 1,048 | 3.29 | −0.01 | 55,924 | 5,482 | 91.15 | 35.83 |
SWE | 3,352,449 | 43.34 | 46.83 | 7.06 | 4,298 | 6.81 | 0.00 | 42,679 | 5,855 | 92.14 | 23.22 |
Average | 2,605,172 | 40.41 | 48.75 | 5.23 | 2,821 | 5.88 | −0.01 | 52,957 | 21,485 | 91.07 | 23.98 |
Note: Sample means and totals over the time coverage of each dataset. Source: National linked employer employee data, see Table 1 for details. |
Footnotes
For a discussion of the measurement of polluting and energy-intensive occupations, see OECD (2024[]) and Causa, Nguyen and Soldani (2024[]). [4]
This approach is comparable to those using GHG-intensity in OECD (2024[1]) and carbon-intensity in Barreto, Krill and Grundke (2023[4]) with the exception that we use a threshold of appearing in the top two deciles of the energy-intensity distribution in at least 15 countries as compared to 10 in the other two classifications. This ensures that the sewerage and waste-collection sector as well as the agricultural sector are omitted from the list of energy-related industries, as these are expected to grow and/or are essential for the clean-energy transition (see also OECD (2024[1])). [5]
Using the same approach for GHG-intensive sectors instead yields two differences. The sewerage and waste collection sector and the agriculture sector are both characterised by relatively high emissions but low energy-intensity. However, in the final classification of high emission sectors in OECD (2024[1]) they are omitted as they are not expected to decline. This partly reflects the role of policies since sewerage and waste collection is very needed for the net-zero transition (D'Arcangelo, Kruse and Pisu, 2023[]). [6]
It is important to note that the present classification is based exclusively on energy demand but – partly by coincidence – also captures key aspects of energy supply. This is most obvious in the case of energy supply. Coke and refined petroleum is energy-intensive because it uses high levels of energy per unit of valued and not because its main activity is energy generation. To a certain extent the same applies to also to energy-intensive manufacturing and services. For example, non-metallic mineral products are energy-intensive in production, but are also potentially relevant for energy supply, e.g. through manufacturing of relevant products derived from critical minerals. Similarly, the definition of energy-intensive service sectors also incorporates parts of the economy directly related to energy supply in the form of distribution (e.g. pipelines in land transport, oil freight in sea transport). [7]
In the event a worker has several jobs with identical total earnings, the spell that occupies most of the worker's time is selected. If total time also identical or no information on working time is not available, the primary job is based on a random draw among those with the highest total earnings (or time worked). [8]
Countries where firm identifiers are used are Australia, Canada, Estonia, the Netherlands and Portugal. [9]
All monetary values are expressed in real terms using the Consumer Price Index with 2015 as the base year. [10]
We account for implausibly low and high earnings by removing employment spells with earnings falling below 20% of median earnings and winsorising earnings at the top 0.1% of the distribution. This adjustment is done based on daily wages, whenever available, annual earnings otherwise. Daily wages are used instead of hourly wages to maximize country coverage. [11]
Note that when we refer to “rest of the economy” we refer to non-energy-related sectors throughout this paper. [12]