RDP 2005-10: Housing and the Household Wealth Portfolio: The Role of Location 4. Empirical Model and Results
December 2005
- Download the Paper 626KB
Before we present the model and estimation results, we first discuss the choice of our central variable, a measure of urbanisation.
4.1 Measuring Urbanisation
Previous urban economics literature gives us little guidance about the appropriate measure of urbanisation. Since we are especially interested in the effects on asset shares due to geographical concentration and the general benefits that cities provide, we confine our search to measures based on population and/or distance.
Population density is perhaps the most straightforward measure for the degree of ‘isolation’ of a local district. We measure population density for each household's Statistical Local Area (SLA), which are government administrative areas. Population density has the advantage that it captures the urbanisation/geographical concentration of the place of residence and it gives a plausible representation of the concentration of cities in Australia (Figure 4).[7] Glaeser (1999) argues that the benefits of living in larger cities are generated from interaction between individuals, while Marshall (1961) argues that intellectual flows between individuals depreciate over space. Hence, we choose population density as our preferred measure. However, it is comforting that other urbanisation measures provide similar empirical results.
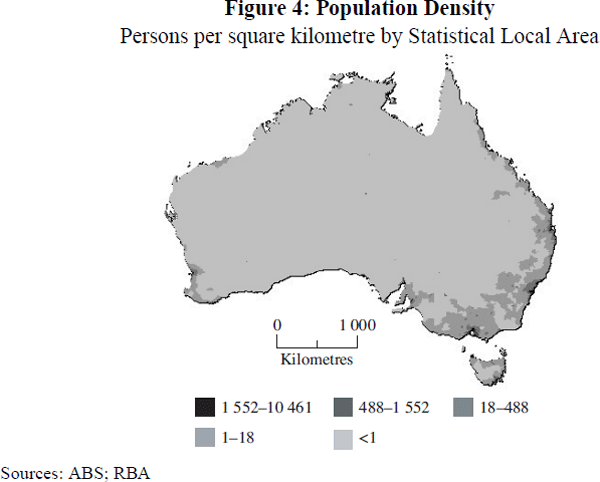
One other common measure, which accounts for both population and remoteness, is the Accessibility/Remoteness Index of Australia (ARIA) for each household's SLA, compiled by the Australian Bureau of Statistics (ABS).[8] ARIA is a ‘measurement of the physical road distance between where people live and the places those people travel to in order to obtain goods and services, and to enjoy the opportunities for social interaction’ (ABS Cat No 1244.0 2001, p 11). As well as accounting for population, ARIA incorporates the relative distance the household must travel to access a full range of services.[9] The ARIA measure identifies relatively urbanised regions further inland than may have been expected for Australia (Figure 5). It is questionable whether the benefits of geographical concentration are sufficiently high in these areas to be driving up dwelling prices to an appreciable extent. Therefore, accessibility or remoteness measured in this way does not appear to be the best proxy for our urbanisation exercise.
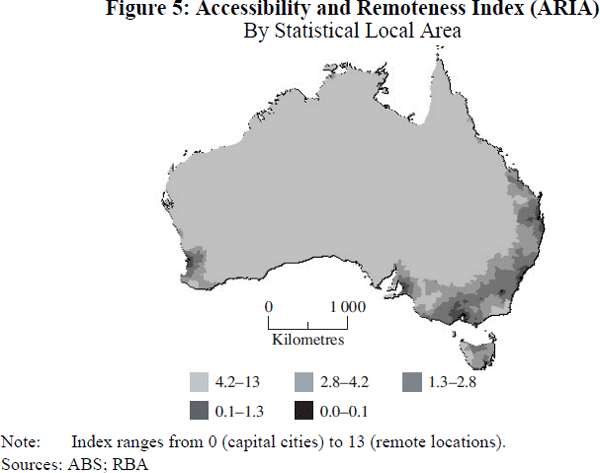
4.2 Model and Methodology
To test the relationship between urbanisation and property asset holdings in the portfolio, we regress the share of total assets held in the form of property on a measure of urbanisation, controlling for other variables that may affect the portfolio decision. We restrict ourselves to modelling only owner-occupiers for two reasons. First, renters have a zero share of housing assets in their portfolio, requiring a more complex modelling strategy such as the two-part models commonly used in the portfolio share literature. For such models, the first stage relates to tenure choice whereas the focus of our paper is on asset allocation. As pointed out in Duan et al (1983), households with a zero share of property in total assets are not missing shares, they simply tell us nothing about the difference in the property share of assets between urban and rural locations. Second, many renters may be unable to afford to buy their own home. In this case, not owning the home is not an asset allocation choice. This is supported by HILDA evidence that renter households tend to have less net wealth than owner-occupiers.[10] A final comment is that the omission of renter households is likely to make little difference as home ownership rates in Australia are similar between rural, other major city or capital city locations.
Because the portfolio shares are bounded between 0 and 100 (in percentage terms), an appropriate model is the logistic regression.[11] In this regression, the dependent variable is transformed and the model estimated using OLS[12], with the equations taking the form:
where: sharei is the percentage of assets held in property of household i; popdens is the population density of that household's Statistical Local Area (SLA); and X is a set of independent household characteristics.
4.3 Estimation Results for All Owner-occupiers
The results from the logistic regression for all owner-occupiers are presented in Table 3. From this table, the sign of the coefficient can tell us the direction of the relationship between a particular variable and the share of housing assets. However, the magnitudes of the coefficients in logistic regressions have no intuitive interpretation. Therefore, marginal effects are provided, evaluated at the sample means.[13]
Variable | Coefficient | Sample mean | Selected unit | Marginal effect |
---|---|---|---|---|
Population density | 0.02*** | 963 persons per square kilometre | 100 persons per square kilometre | 0.43*** |
Household income | −2.30×10−3*** | $67,892 | $1,000 | −0.04*** |
Household income squared | 3.15×10−6*** | |||
Net wealth | −9.62×10−4*** | $555,982 | $1,000 | −0.02*** |
Net wealth squared | 5.88×10−8*** | |||
Age | −0.05*** | 50.10 years | 1 year | −0.20*** |
Age squared | 4.20×10−4*** | |||
Divorced | 0.22*** | 0.05 | Compared with married | 5.22*** |
Separated | 0.18* | 0.04 | Compared with married | 4.16* |
Widowed | 0.25*** | 0.09 | Compared with married | 5.77*** |
Earns rental income | −0.55*** | 0.18 | Not earning rental income | −13.46*** |
Gender | −0.08** | 0.64 | Compared with female | −1.85** |
Tradesperson | 0.09* | 0.10 | Compared with professional | 2.14* |
Intermediate production worker | −0.11* | 0.06 | Compared with professional | −2.71* |
Not working | 0.29*** | 0.30 | Compared with professional | 6.75*** |
Part-time employed | 0.15*** | 0.12 | Compared with full-time employed | 3.64*** |
Couple with no children | −0.15*** | 0.32 | Compared with couple with children | −3.56*** |
Lives alone | −0.26*** | 0.27 | Compared with couple with children | −6.30*** |
Single parent | −0.19* | 0.03 | Compared with couple with children | −4.53* |
Constant | 2.34*** | |||
Number of observations = 4,422 | ||||
R2 = 0.36 | ||||
Note: ***, ** and * represent significance at the 1, 5 and 10 per cent levels, respectively. |
4.3.1 Population density
Our model shows a strong relationship between urbanisation and the share of the own home in total assets. Even after controlling for household income, net wealth, and other household characteristics, we find that the degree of urbanisation increases the share of assets held in the own home. Figure 6 graphs the estimated housing share of total assets at various population density (urbanisation) levels. To do this, we increase population density by equal increments while setting all other explanatory variables to their average values. Figure 6 shows, for example, that for households residing in Cairns – a population density of around 5,600 persons per square kilometre – the share of assets that they hold in their own home is estimated to be 78 per cent, controlling for other factors. This is quite a large share, given that Cairns is not even a capital city, and thus highlights the sizeable effect that living in more agglomerated cities can have on home values.
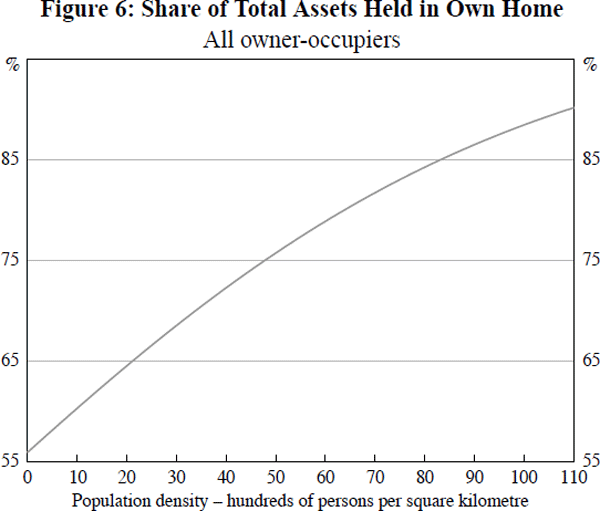
Also of interest is the shape of the housing-share curve. This provides us with the marginal effects of urbanisation on the share of assets devoted to housing at each population density level. From Table 3 we know that the average marginal effect of population density on the share of housing wealth is quite large, with an increase in the population density of 100 persons per square kilometre increasing the share of housing assets by 0.4 percentage points, on average. However, Figure 6 also illustrates that this marginal effect is not constant across population density levels. For example, an average household moving from Cairns to Brisbane city – an increase in urbanisation of around 2,000 persons per square kilometre – will have an estimated increase in their share of total assets held in their own home of 5.6 percentage points. Yet, a move between the less dense cities of Canberra and Perth – the same 2,000 persons per square kilometre increase in urbanisation – results in a larger increase in the housing share of total assets, of 8.0 percentage points.[14], [15] In fact, the maximum marginal effect of population density on housing asset shares appears to occur at a population density level well below our sample range; this is the point where the slope of the housing-share curve is at its steepest.[16]
The shape of the housing-share curve suggests that a given rise in urbanisation has a slightly larger effect on the holdings of housing assets for households living in more rural areas than for those living in more urban areas. Because we know from Table 2 that the quality of housing is not appreciably different between urban and rural areas and our regression results control for income and wealth, the additional benefits of urbanisation are likely to be due to such things as access to a fuller range of amenities and the depth and frequency of human interactions that a more populated city can bring. Hence, it is perhaps not unexpected that the marginal effect of this is greatest for rural areas. However, an alternative interpretation of the shape of the housing-share curve is that the marginal non-pecuniary benefits of urbanisation might be constant (or even rising) with population density, but the marginal costs associated with a more concentrated asset portfolio could be increasing. That is, households may become increasingly reluctant to increase their share of assets held in one house, thereby leading to the flattening shape of the housing-share curve. By extending our analysis to multiple property owners we will be able to determine whether it is non-linear portfolio concentration costs or non-linear urban-premium benefits that are dictating the shape of the owner-occupier housing-share curve (for their own home).
4.3.2 Household characteristics
Our estimated model in Table 3 controls for a range of other factors that could affect the housing share in total assets. Household income has a negative effect on the share of assets held in housing; at sample means, as the income of the households rises by $1,000, the share of housing assets is estimated to fall by 0.04 percentage points. One possible reason for this, supported by results found in Rosen and Wu (2004) for the US, is that households with higher incomes hold a higher share of their assets in superannuation because of their higher employment income. It is interesting, however, to compare this negative coefficient with results from probit models, which find that the probability of owning a house actually increases with income (Kohler and Rossiter 2005). In combination, these results support the idea that households want a certain level of housing services, but beyond this a declining share of additional income is devoted to housing services.[17]
We also find that net wealth has a negative effect on the housing share of wealth, with an increase in net wealth of $1,000 decreasing the share of assets that households hold in their own home by 0.02 percentage points, on average.[18] This suggests that higher net wealth results in diversification into other assets, including, perhaps, other property assets.[19] This evidence is similar to that by Rosen and Wu (2004) who find for the US that superannuation, bonds and risky assets increase with net wealth, while safe financial assets decline with net wealth.
Consistent with life-cycle considerations, we find that the share of wealth in housing declines with age by 0.2 percentage points per year, on average. This is in line with results found by Fisher and Weber (2004), which suggest that the probability of holding a greater share of total assets in financial assets increases with age. Similarly for Australia, La Cava and Simon (2003) find that the probability of being financially constrained declines with age. Interestingly, there is a significant positive effect from the quadratic age term. This indicates that as households go into retirement (at around age 60), they no longer contribute to their holdings of other assets (such as superannuation) and in fact commence drawing them down, increasing the share of housing.
Male household heads were found to hold 1.9 percentage points less of their assets in the home than households headed by a female. As per the results for net wealth, this is likely to be related to the fact that male-headed households tend to be more diversified in their asset holdings; Rosen and Wu (2004) find that female-headed households hold a higher share of their portfolio in safe assets rather than superannuation, bonds or risky assets. Including a variable which interacts gender and single person households (results not shown) lessened the negative effect of gender. Single male households tend to hold less of their assets in the home because these households tend to hold a higher share of their assets in vehicles and risky financial assets than other household types, on average.
It was not unexpected to find that households that earn rental income hold a smaller share of their assets in their own home, consistent with the fact that the majority of these households own another property. In fact, there may be some evidence of substitution between investment in the own home and in rental properties given that for households earning rental income, the share of assets devoted to their own home is 13.5 percentage points lower than it is for owner-occupier households not earning rental income.
4.4 Estimation Results for Multiple Property Owners
We have also estimated our model for a subset of households that own both their own home and additional residential property (which may be a holiday home or a rental property). The results for this group may allow us to shed more light on the effect of the different motives for holding housing assets, the consumption of housing services (including associated amenities available in the vicinity of that house) and investment in property assets. Unfortunately, we have no information about the location of the investment property. We therefore consider total property rather than investment property on its own. We compare these households' own home and total property wealth to get some idea as to their relative demand for housing services and benefits of living in an urban area, and the demand for investment in property assets. A comparative set of results to those given in Table 3 are presented in Tables 4 and 5.
Variable | Coefficient | Sample mean | Selected unit | Marginal effect |
---|---|---|---|---|
Population density | 0.02*** | 1,102 persons per square kilometre | 100 persons per square kilometre | 0.40*** |
Household income | −1.65×10−3** | $92,054 | $1,000 | −0.03** |
Household income squared | 3.01×10−6** | |||
Net wealth | −4.78×10−4*** | $978,045 | $1,000 | −0.01*** |
Net wealth squared | 2.24×10−8*** | |||
Age | −0.03** | 49.64 years | 1 year | −0.16*** |
Age squared | 2.39×10−4** | |||
Never married | −0.27*** | 0.10 | Compared with married | −6.07*** |
Earns rental income | −0.19*** | 0.64 | Not earning rental income | −4.35*** |
Associate professional | −0.16** | 0.13 | Compared with professional | −3.58** |
Intermediate production worker | −0.19** | 0.05 | Compared with professional | −4.34** |
Labourer | 0.22* | 0.03 | Compared with professional | 5.23* |
Not working | 0.16* | 0.19 | Compared with professional | 3.78* |
Part-time employed | 0.14** | 0.14 | Compared with full-time employed | 3.36** |
Couple with no children | −0.20*** | 0.36 | Compared with couple with children | −4.65*** |
Constant | 0.88*** | |||
Number of observations = 921 | ||||
R2 = 0.32 | ||||
Note: ***, ** and * represent significance at the 1, 5 and 10 per cent levels, respectively. |
Variable | Coefficient | Sample mean | Selected unit | Marginal effect |
---|---|---|---|---|
Population density | 0.02*** | 1,102 persons per square kilometre | 100 persons per square kilometre | 0.38*** |
Household income | −2.98×10−3*** | $92,054 | $1,000 | −0.04*** |
Household income squared | 5.86×10−6*** | |||
Net wealth | −5.60×10−4*** | $978,045 | $1,000 | −0.01*** |
Net wealth squared | 3.01×10−8*** | |||
Age | −0.06*** | 49.64 years | 1 year | −0.17** |
Age squared | 5.05 ×10−4*** | |||
Earns rental income | 0.21*** | 0.64 | Not earning rental income | 4.66*** |
Gender | −0.21*** | 0.67 | Compared with female | −4.45*** |
Not working | 0.22* | 0.19 | Compared with professional | 4.58** |
Part-time employed | 0.17* | 0.14 | Compared with full-time employed | 3.67* |
Dependent | −0.80*** | 2.17×10−3*** | Compared with couple with children | −19.04*** |
Couple with no children | −0.27*** | 0.36 | Compared with couple with children | −5.87*** |
Constant | 2.81*** | |||
Number of observations = 921 | ||||
R2 = 0.27 | ||||
Note: ***, ** and * represent significance at the 1, 5 and 10 per cent levels, respectively. |
4.4.1 Population density
Figure 7 shows the asset share of the own home and of all property holdings for owner-occupiers with multiple residential properties. This gives us some indication of the relative effects that urbanisation might have on each type of property share. First comparing the home owner results of Figure 6 with Figure 7, we can see that when more than one property is owned by the household, the share of assets held in the own home is smaller; for example, for a population density of around 5,600 (Cairns) the share is now only 56 per cent of assets, compared with 78 per cent across all owner-occupiers. Also, for multiple property owners the consumption and investment preferences regarding property can be identified: their consumption preference through the choice of their own home and their investment decision through the purchase of other property – that is, the additional amount they wish to hold in total property assets (Henderson and Ioannides 1983). Because these households are not likely to be constrained in their consumption decision, the slope of their own-home curve specifically gives us a measure of the benefits of living in a more populated city. Figure 7 shows that the own-home curve is now rising at a relatively constant rate over our sample of multiple property owners, by about 0.4 percentage points for every 100 persons per square kilometre.[20] This implies that the benefits of urbanisation increase linearly across the entire sample range of urbanisation levels. What this suggests for all owner-occupiers (Figure 6) is that the marginal benefit of living in more urban areas is not declining at higher population densities. Instead it seems that there is a rising marginal cost associated with holding an asset portfolio that is increasingly concentrated in housing.
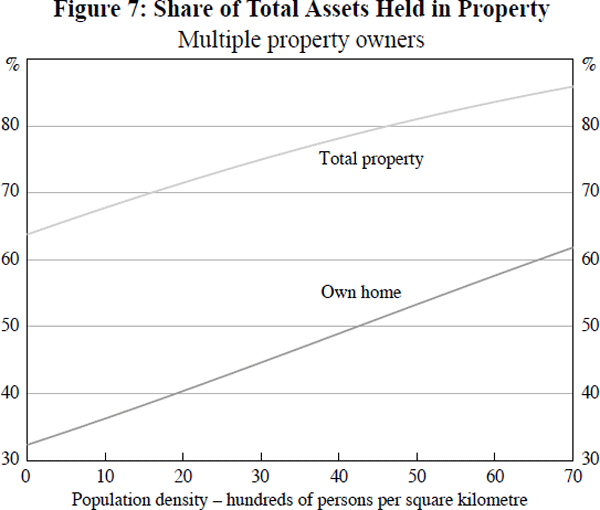
This latter point is supported by the shape of the total property curve for multiple property owners in Figure 7. This shows that an increase in the population density of 100 persons per square kilometre results in a 0.4 percentage point increase in the total property share, on average. More importantly, and unlike for the own home, the slope of the curve for the share of assets held in total property is declining at higher levels of urbanisation.
4.4.2 Household characteristics
For multiple property owning households, income and net wealth have a negative, statistically significant and non-linear effect on the share of assets held in the home. The age variable is consistent with the life-cycle hypothesis; as before, a one year increase in age decreases the share of assets held in the own home by 0.2 percentage points, on average. Also as before, if the household earns rental income, there is a smaller share of assets held in the own home, though for multiple property owners the share is only 4.4 percentage points lower than it is for those not earning rental income, compared with the 13.5 percentage point difference when all owner-occupiers are examined.
Turning to the total property regressions, we find that household income, net wealth and age have broadly the same effects on the total property share as for the own home regressions. In contrast to the own home regressions, we find that those households that earn rental income (which is likely to come from an investment property) hold a higher share of assets in total property than multiple property holders without rental income.
Footnotes
The maps (Figures 4 and 5) are divided into quintiles of SLAs. There are a greater number of SLAs in more urban areas. [7]
Other measures we considered were population and simple dummy variables for location. Again, the results were robust to the use of these measures. [8]
The index is only available for 1996 SLAs, thereby requiring some judgment in allocating the index into the appropriate 2001 SLAs. [9]
Five households in our sample hold 100 per cent of their assets in the home and are also excluded. We drop these from our sample because they are small in number and, unlike more liquid financial assets, it is unusual for households to hold 100 per cent of their assets in the home; hence, these are likely to be data errors. In fact, Poterba and Samwick (2003) in their study of US financial assets choose a two-limit tobit estimator with truncation at zero and one. Instead of following this approach, Rosen and Wu (2004) employ the one-limit tobit model with truncation at zero for reasons of simplicity. [10]
In order to restrict the portfolio shares, the transformed dependent variable takes on values between −∞ and ∞. Potentially, this could induce the problem of outliers in the transformed dependent variable for shares close to the boundaries of either 0 or 100 per cent. We choose to estimate shares between 5 and 95 per cent though this has little effect on the results. [11]
Because the dependent variable is a share, the variance of the error term is heteroskedastic. We therefore report the White heteroskedasticity-consistent standard errors. [12]
The marginal effects and their significance were calculated using the predictnl command in Stata/SE 9.0. [13]
Population densities of Cairns and the cities of Brisbane, Canberra and Perth are 5,641, 7,631, 968 and 2,904 persons per square kilometre, respectively. [14]
This experiment of changing population density while holding all other household characteristics constant ignores the potential link between income/wealth and urbanisation due to the wage-premium effect. One way to account for this is to include variables for the average income and wealth of each SLA in the regression analysis (while transforming individual income and wealth variables to be in terms of deviations from SLA averages). Doing so does not substantially alter the results, particularly with regards to population density. The coefficient estimate for average wealth is significant (at around −4.76×10−4) but not the coefficient for average income. Now consider a household moving from SLAs with population densities similar to Canberra to SLAs with densities similar to Perth. Instead of holding wealth constant, assume that average wealth moves up according to the average of these SLAs. The housing share of total assets still rises, but by 2 percentage points less than the case when wealth is assumed to be constant across SLAs. [15]
Very remote parts of Australia are excluded from the HILDA Survey sample. [16]
The significant quadratic effect suggests that at very high income levels a greater share of assets are held in the home. However, this effect only begins to take effect for household total income levels greater than $365,000, an income level applicable to less than 0.4 per cent of our sample, and may therefore be a result of our assumed functional form rather than an exact empirical estimate. [17]
While average net wealth reported in Table 3 may appear quite high, this is a result of the skewness of the distribution of net wealth. Median net wealth is $362,000. [18]
There is also a significant quadratic effect suggesting that at very high wealth levels ($8 million, representing less than 0.1 per cent of our sample) households choose to hold a higher share of assets in their own home. But the very small sample size available to estimate this turning point makes this result imprecise. [19]
As restricted by the model, the share is bounded between 0 and 100 and hence will flatten out at very high levels of population density. [20]