RDP 2011-03: Urban Structure and Housing Prices: Some Evidence from Australian Cities 3. Determinants of the Urban Structure
September 2011
- Download the Paper 613KB
We now turn to analyse the impact that choices about transport infrastructure, zoning and development policies, and population size have on the structure of cities. Following Newman, Kenworthy and Vintila (1992), one can consider the structure of a city as influenced by a number of factors including:
- the transport possibilities available for residents;
- policies with respect to development and infrastructure on the fringe;
- the priority given to the provision of space (or the avoidance of density) within the city; and
- the planning practices and institutions that affect the three foregoing factors.
We model these influences by changing aspects of the calibration described above. In particular, we discuss three types of public policies that affect housing market outcomes: (i) policies that impact on the efficiency of the transport infrastructure within the city, (ii) policies that limit housing density, and (iii) policies that increase the cost of building new housing. As is noted above, the model is a partial equilibrium one, which implies that the analysis cannot capture all the effects of such policies. While we expect that the results that we highlight are of first-order importance for variables such as housing prices, we also discuss some of the associated trade-offs likely to be present in more real-world settings. In each case, it is also important to remember the static nature of the model, so that we are not specifically modelling the short-run effects of changes in such policies on an existing city. Rather, the exercises that follow could be thought of as comparing the long-run equilibrium structure of two otherwise identical cities, if they were to differ only in the application of a particular policy.[5]
3.1 Transport Costs
We first consider the impact of policies with respect to transport infrastructure. In particular, we contrast the urban structure of two cities, one where there has been significant investment in transport infrastructure – defined broadly to include both public transport and car-based road infrastructure – and the other where the transport infrastructure is less well developed and transport costs are higher as a result. We abstract from any issues relating to the financing of the transport infrastructure as they are beyond the scope of this paper. Considering these costs would, of course, reduce the net gains to households that come from improved transport infrastructure.[6]
In the calibration, we consider the effect of doubling transport costs, with the results shown in Figure 3. Because commuting is more costly, households have a stronger incentive to live closer to the CBD, and the city size is significantly smaller as a result (lower right panel), with the calibration suggesting a fall in the radius of the city from 35 kilometres to around 21 kilometres. To accommodate the denser population, building heights are higher close to the CBD (middle left) and dwelling sizes are smaller (upper right). Housing and land prices are also higher close to the CBD (upper and lower left panels). At greater distances from the CBD, several of the curves cross: with higher transport costs, it is more costly to live further out, so housing prices, land prices and density near the new city fringe (around 20 kilometres) are lower than at the same distance under low transport costs. This is not to say, however, that any part of the population is better off. For example, if we were to rank households by distance from the CBD, then households at any distance percentile have a smaller home and face higher housing prices (per square metre) under higher transport costs than households at that same percentile under lower transport costs.
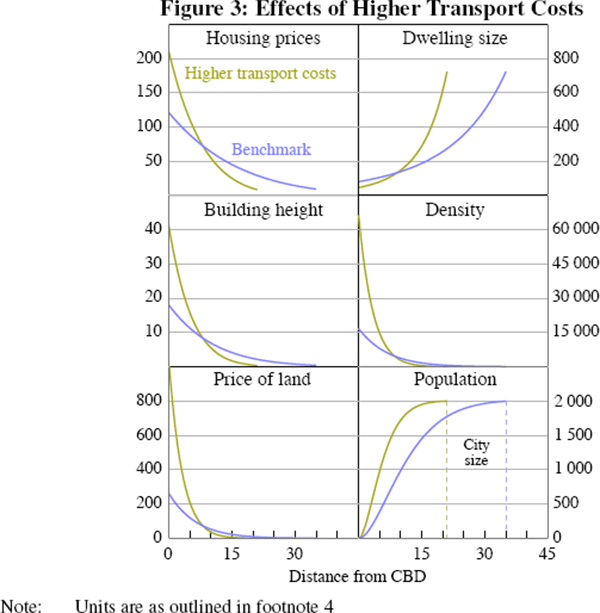
Overall, the effect of poor transport infrastructure and higher transport costs is that households spend more of their resources commuting, live in smaller homes and face higher average housing and land prices. By contrast, the model suggests that well-directed investment in transport infrastructure makes it more feasible to live further from the CBD and can thereby reduce the cost of housing.
3.2 Density Restrictions
Next, we consider the effect of zoning policies that reduce the density that would result in an unconstrained case. In practice, there are a wide range of restrictions placed on the usage of land for housing, in many cases to limit the density of housing. These include zoning rules that allow only relatively low density uses for particular areas or blocks, or subdivision rules that set minimum limits on lot sizes or street frontages. For the current exercise, we consider a situation where zoning rules limit the density of housing by imposing an across-the-city restriction on the floor-to-area ratio (FAR) of buildings, that is a restriction on the total amount of floor space that can be produced on any unit of land. While in the model all buildings at a given distance from the CBD are identical in height, the FAR that we impose can be thought of as a restriction on average building height in any neighbourhood. Thus our analysis can be viewed as consistent with a range of types of density restrictions, including real-world examples where some land in a suburb can be used for higher-density housing and other land is zoned to only permit single-family houses. As in Bertaud and Brueckner (2005), we apply a FAR restriction that corresponds approximately to a limit (on average) of four stories in any area.
Figure 4 shows the impact on the equilibrium structure of a height restriction across the city. This type of zoning restriction constrains the supply of housing, with the restriction obviously most binding close to the CBD where building height would naturally be highest. Because a significant proportion of the city's population are unable to live in higher-density housing close to the the CBD, the population on average is ‘forced outwards’, overall city size is larger (bottom right panel), overall density is lower, and the population devotes more resources to commuting. The zoning restrictions force building height close to the CBD to be lower, but building height in the middle and outer suburbs is higher than it would otherwise be (middle left), to accommodate the people forced out from close to the CBD.
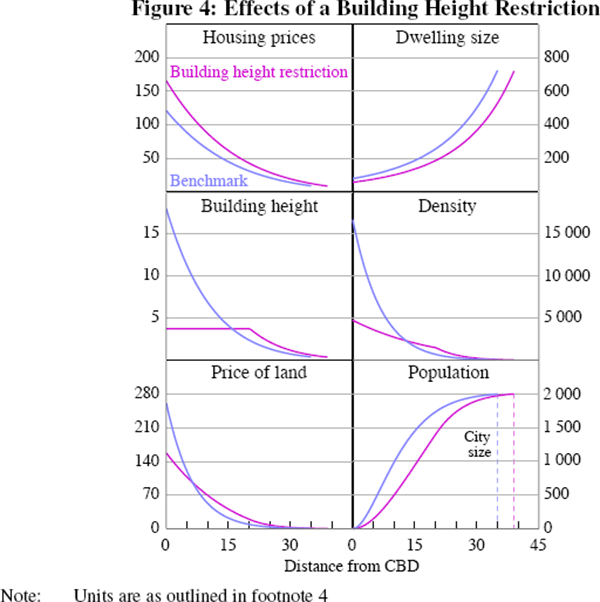
The price of housing is higher at all distances from the CBD (top left) because the supply of well-located housing has been reduced. As a result of the higher price of housing, dwelling size is lower at all distances from the CBD (top right). The effect on land prices, however, differs depending on distance from the CBD. Land prices are lower close to the CBD (bottom left), where density is much lower than in the unconstrained city (middle right), because developers cannot build as high and therefore accommodate as many people. But land prices and density are higher than they would otherwise be in the middle and outer rings, where there is increased demand for housing to accommodate those residents who are unable to live close to the CBD.
While the model is not dynamic, Figure 4 points to the type of adjustment that could be expected over time if there were to be a significant city-wide easing in zoning restrictions. While the cost of housing could be expected to fall across the city, the price of land close to the CBD would be expected to rise, given that more housing can now be built on that land.
In addition, the calibrated model also allows us to compute, for any distance from the CBD, what the value of a single block of land would be if the zoning restriction did not apply solely for that block. Figure 5 adds one curve to the lower left panel of Figure 4. This curve shows the value of a block of land at each distance from the CBD, assuming that the height restriction did not apply for that block. The difference, as one would expect, is larger closer to the CBD. Indeed, whereas the absence of the zoning restriction for all land implies land prices are about 66 per cent higher near the CBD, in the absence of the restriction for just a single block near the CBD the land value is around 260 per cent higher. The differential between these scenarios gradually declines as one moves outwards, and there is no difference where the zoning restriction no longer binds.
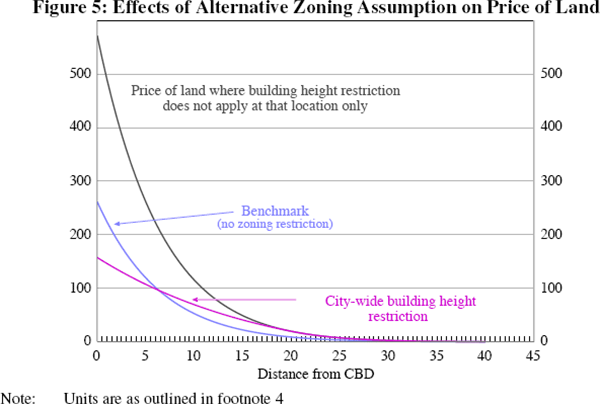
The large difference in the value of the land close to the CBD highlights the potentially significant incentives for landowners to seek ‘spot’ rezonings in circumstances where zoning laws are strongly binding. For example, Evans (1999), in discussing the case of the United Kingdom, notes that ‘because planning permission is worth acquiring, it has been worth spending money and resources to acquire it. So ... developers have often incurred substantial sums of “rent seeking expenditure” to try to persuade local planning authorities and the central government, if necessary, that planning permission should be given’. Indeed, Glaeser, Gyourko and Saks (2005a) set up a theoretical model where spending by developers and existing town residents are factors influencing decisions on land development by the relevant zoning body.
While the preceding results focus on the costs of binding zoning restrictions, in terms of higher housing prices resulting from reduced supply of housing, there are, of course, also benefits associated with well-designed zoning. For example, there are benefits from separating housing and industrial activity, and preserving green space within cities. More generally, there are a range of trade-offs associated with zoning policies. Our analysis highlights the effect on housing prices of reducing the supply of housing. Of course, policies to limit density may reflect other preferences of the existing population – which the model abstracts from – such as a preference to live ‘less densely’. However, the analysis highlights an important point: there is an unavoidable trade-off between density and housing prices.[7]
3.3 Frictions on Housing Production
We now consider the effect of other policies that increase the cost of housing supply. More specifically, we model the effect of frictions that create a wedge between the purchase price (the price consumers pay for housing) and the price that producers receive.
This exercise is an attempt to capture the effect of various cost-increasing factors cited by a range of Australian observers (e.g. Applied Economics (2010), Chapter 4 of Caplin et al (2003), Gurran, Ruming and Randolph (2009) and Productivity Commission (2011)). These factors include long and uncertain planning processes involving multiple state and local authorities, costs associated with structuring new developments to comply with various requirements, infrastructure charges associated with new developments (either within the city or at the fringe) that are in excess of any benefits developers or homebuyers may receive, and the interest costs associated with lengthy delays in the development process.[8]
Of course, any development process will involve steps which have associated costs and benefits. For example, while meeting environmental standards represents a cost to developers and homebuyers, these standards also result in private and social benefits. The model does not capture any such benefits. Instead, the frictions that we model can be viewed as capturing the extent to which costs to developers exceed any associated benefits they or homebuyers may receive.
Figure 6 illustrates the impact of frictions in the production of housing, such that there is a wedge that lifts the selling price by 25 per cent above the cost of supply. In the city with frictions in production, consumers pay a higher price than otherwise at all locations (upper left panel). As a result people live in smaller dwellings (upper right). As these frictions make it less profitable to produce and sell housing, housing supply and building height fall at all locations (middle left). With housing development less profitable and less demand for housing, the price of land falls at all locations (bottom left). There is a small reduction in the size of the city, but overall population density is broadly unchanged with effects of dwelling sizes and building heights broadly offsetting (middle and lower right). With households facing higher prices and living in smaller dwellings, they are worse off as a result of the frictions in housing production.[9]
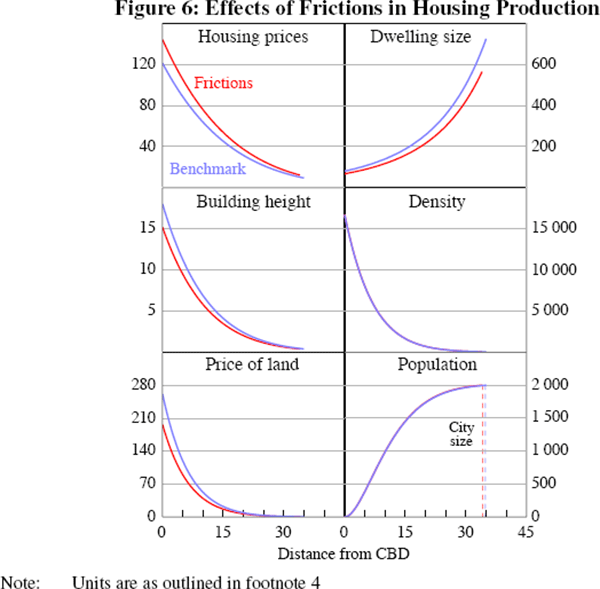
3.4 Population Growth
How would an unconstrained city adjust to a larger population? The model is static and therefore cannot capture the dynamics associated with urban change. It is nevertheless useful for a comparative analysis of different longer-run urban equilibria. In particular, the model allows us to compare the equilibrium structure of two cities with otherwise similar characteristics (incomes, preferences, housing production technology, transport costs, etc) but differing in population. In Figure 7 we compare the equilibria of two cities with populations of two and four million people.
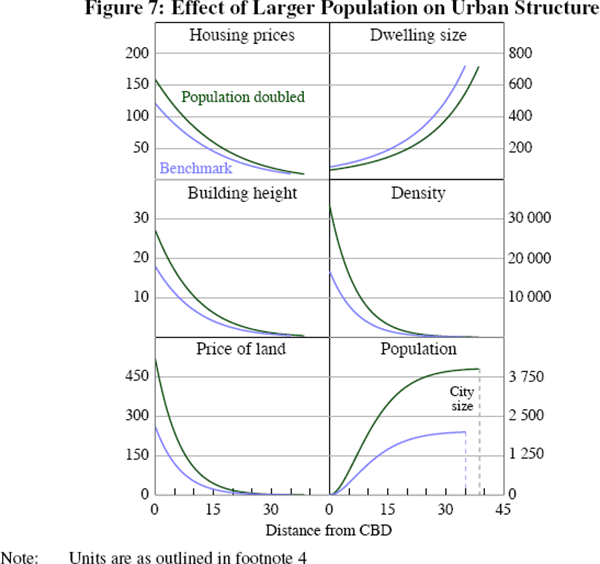
Not surprisingly, with greater demand for housing, housing and land prices are higher at all locations in the city with a larger population (upper and lower left panels). Because of higher housing prices, households demand smaller dwellings (upper right) and population density is higher (middle right). Building height rises at all distances from the CBD (middle left). The larger population results in the city occupying a larger area (lower right).
However, the increase in city size implied by our calibration is arguably quite small, with the radius of the city rising by only 9 per cent (from around 35 to 38 kilometres) and the area rising by 18 per cent, despite a doubling of the population. The reason is the increase in building heights and the reduction in dwelling sizes. Indeed, although there are two million more individuals in the larger city, only around 1 per cent of these live further from the CBD than the edge of the smaller city. In this light, the metropolitan strategies for the large Australian cities that target 50–70 per cent of population growth occurring in infill housing rather in greenfield housing might not seem unreasonable.[10]
We can also use the model to consider how a city constrained by zoning policies would adjust to a growing population. As before, the zoning restriction we consider is a FAR that limits building heights to around four stories. Figure 8 compares the equilibrium housing price (per square metre of housing) at 10 km from the CBD for different populations from 250,000 to four million, for two cases: that of an unconstrained city and that of a city with a zoning restriction like a FAR. For small populations there is almost no difference in housing prices because zoning restrictions are not really binding at 10 kilometres from the city. For larger populations, there is a gradually larger impact because zoning restrictions prevent the natural tendency towards higher buildings.
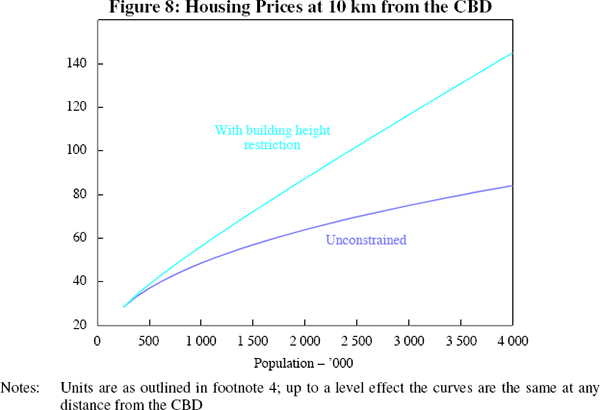
To get a further sense of the magnitudes involved, we consider the impact on housing prices of relaxing the zoning restriction. In particular, we increase the maximum FAR by one story. We find, for a population size of two million, that an extra story, by allowing increased housing supply closer to the CBD, leads to housing prices that are around 10 per cent lower. However, for a population of four million, allowing buildings to be higher by an extra story results in housing prices that are around 13 per cent lower. That is, with zoning restrictions, population growth results in a larger increase in equilibrium housing prices than would be the case in an unconstrained city.
Footnotes
Brueckner (2007) also discusses the effects of various forms of government land-use interventions. [5]
However, see, for example, Centre for International Economics (2011) for a study that points to net economic benefits from seven proposed transport projects in the Sydney metropolitan area. [6]
See Evans (2004) for a broader discussion of economic issues surrounding zoning and other land-use controls and Gurran (2007) for a discussion of the procedural aspects of the planning process in Australia. [7]
To illustrate the potential for the planning process to have a significant impact on the economics of development, we note that one state agency showed us a Gantt chart that showed more than 500 required steps that developers faced in the various phases of the planning process. [8]
As noted above, the analysis uses the ‘closed-city’ version of the model where the population is fixed. Alternatively, our analysis can be thought of as capturing the situation where the policies that we model apply to all cities within an economy (and external migration is small). In an ‘open-city’ case, where households have the choice of living in other cities not subject to such policies, we would expect smaller adverse effects on prices and dwelling size. Aura and Davidoff (2008) model this effect, showing that loosening supply-side constraints in one jurisdiction may have little effect on prices, but a co-ordinated easing across markets may have large effects. [9]
Of course, the results from our calibration should be viewed as applying to cities that could costlessly adjust to accommodate a higher population and increase in density. A model that allowed for path dependence, especially the sunk costs associated with existing housing and infrastructure, would no doubt indicate more population growth would occur at the fringe. [10]