Bulletin – July 2024 Australian Economy Assessing Potential Output and the Output Gap in Australia
- Download 838KB
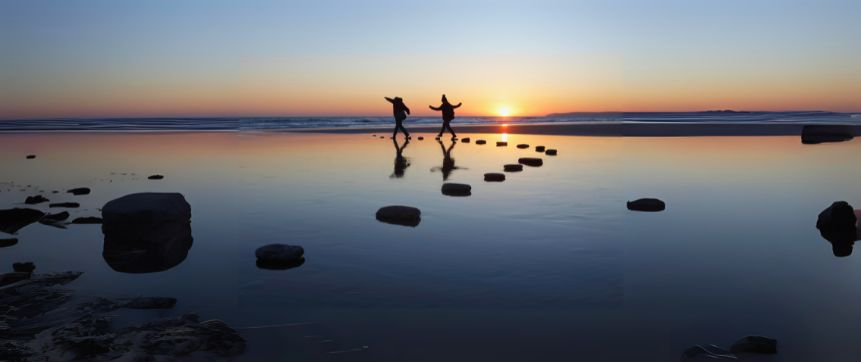
Abstract
The output gap – the difference between actual output and potential output – is an important consideration for monetary policy as it is a measure of the extent of spare capacity in the economy. This article explains how RBA staff form an assessment of potential output and the output gap. We draw on a range of model-based estimates, capacity utilisation indicators and activity measures. Model-based estimates give a quantitative assessment of the level of spare capacity in the economy, but there is considerable uncertainty modelling unobserved concepts like potential output and the output gap. Ultimately, assessing spare capacity in the economy requires careful judgement in weighing up all available information, which the RBA sets out in its quarterly Statement on Monetary Policy.
Introduction
Monetary policy in Australia primarily influences aggregate demand – that is, the overall spending on goods and services in the economy. How the level of aggregate demand affects consumer price inflation and the labour market depends on the productive capacity of the economy. Potential output is a measure of this productive capacity. It is not an objective of monetary policy and monetary policy can do little to directly influence it. However, the output gap – the difference between actual output and potential output – is a measure of spare capacity in the economy that is closely related to the RBAs objectives of price stability and full employment. Following the 2023 Review of the RBA, the Treasurer and the Reserve Bank Board agreed in the Statement on the Conduct of Monetary Policy that the RBA would regularly publish its assessment of potential output (Treasurer and Reserve Bank Board 2023). An explanation about the role of potential output and the output gap in monetary policy was provided in the May Statement on Monetary Policy (RBA 2024a). This article explains in more detail how RBA staff form an assessment of potential output and the output gap.
Potential output and the Australian economy
What is potential output?
Potential output is the total amount of goods and services that can be produced by an economy that is operating at a sustainable capacity. In this context, sustainable means being consistent with low and stable inflation over the business cycle. When output is equal to potential output, the economy is considered to be in balance. Over the medium-to-long term, an economy that is operating at its potential level of output is also achieving both price stability and sustained full employment.
Potential output captures the productive capacity of the economy, which grows over time, and so determines the longer term economic growth that Australia can sustain. It depends on all the inputs that are available for production – so-called factors of production – and how efficiently and intensively they are used to produce goods and services – so-called multifactor productivity (MFP). It is common to focus on two primary factors of production: labour, which comprises total hours worked; and capital, which comprises all the buildings, machinery and equipment used by firms, as well as intellectual property assets like computer software.[1]
The level of potential output varies over time because of structural changes in the availability of labour and capital, as well as changes in productivity. These changes are likely to have an enduring effect on the productive capacity of the economy. The availability of labour evolves with growth in the overall size of the population and the number of hours each person is willing and able to work when the economy is at full employment. The stock of capital increases or decreases as firms invest in new assets and maintain or retire assets that have depreciated over time.[2] Productivity growth captures how efficiently the economy can use its resources to produce goods and services, which will depend on drivers including technological progress, the skills of the workforce and firms managerial expertise. When productivity grows, the economy can produce more output even if no additional labour or capital is used. All else being equal, productivity growth can reduce inflationary pressure in the short term, as goods and services can be produced more cheaply by using inputs more efficiently.
Temporary shocks to the production of goods and services will affect the economys supply capacity for only brief periods. As a result, they can affect short-run aggregate supply, but are not likely to affect potential output and are less likely to matter for inflation over the medium term. For example, severe weather, such as floods, can temporarily disrupt the domestic production of certain foods. While such events can lead to a shortage of these foods, and put significant upwards pressure on their prices, the effects on the overall productive capacity of the economy are typically not persistent enough to affect potential output over the horizon that is important for monetary policy. That said, if such shocks are more frequent or intense, this could affect the available resources of the economy in an ongoing manner and so weigh on potential output over time.
What is the output gap?
The level of potential output provides a benchmark to assess the balance of aggregate demand and aggregate supply. A simple aggregate demand/aggregate supply framework can illustrate this balance, as shown in Figure 1. In the figure, aggregate demand reflects the total demand for goods and services in the economy and potential output reflects long-run aggregate supply in the economy.[3] The aggregate demand (AD) curves are downward sloping as demand is higher when inflation is low; while the potential output curve is vertical as it reflects the long-run productive capacity of the economy, regardless of prices. There is also a short-run aggregate supply (SRAS) curve, which reflects actual supply or how suppliers meet aggregate demand in the short run. The SRAS curve is upward sloping as businesses are more willing to supply goods and services as prices rise.
When output exceeds potential output (point A), in this case due to higher demand, there is a positive output gap. The economy can accommodate this in the short run, as aggregate supply can exceed potential output in the short run by utilising existing factors of production intensively to meet demand (moving along the SRAS curve). That is, workers can increase their hours and firms can ramp up their use of existing capital. But this cannot be sustained without inflationary pressures rising. Wage pressures will increase due to tight conditions in the labour market and other input costs will rise when firms operate their stock of capital intensively and compete for scarce inputs. As a result, inflation will typically rise above target. This is costly as higher inflation erodes real incomes and can hamper long-run economic growth by introducing uncertainty into the economy. To bring inflation back to target, the RBA may need to raise the nominal cash rate. This reduces aggregate demand (demand shifts to AD0), bringing the economy to point C. At point C, the economy is considered to be in balance; aggregate demand is at the level such that output is equal to potential output and inflation is at target.
On the other hand, when aggregate demand is weak and output is below potential output (point B), there is a negative output gap. A negative output gap often coincides with employment below full employment and capital being underutilised. This is costly in terms of the lost production and consumption from not making full use of Australias resources, and in terms of the financial and social costs of employment being below full employment. The experience of many economies after deep recessions has led to suggestions that demand shortfalls can have very persistent (or even permanent) effects on potential output; for example, through so-called labour market hysteresis or scarring effects whereby people who are unemployed for an extended period experience a gradual erosion of their skills and become more likely to leave the labour force altogether. Along with the social costs of long-term unemployment, potential output is also reduced. A negative output gap will also see inflationary pressures (such as wage and other input cost pressures) decrease, which will lead to inflation falling below target. To bring inflation back to target, the RBA may need to lower the nominal cash rate. This will increase aggregate demand (demand shifts to AD0), bringing the economy to point C and back into balance.
How does potential output relate to full employment?
Full employment is the maximum level of employment consistent with low and stable inflation over the business cycle.[4] While this implies a balance between demand and supply in the labour market, it also requires demand for goods and services to be in balance with what the economy can sustainably produce, and thereby economic activity in line with potential output. Over the medium-to-long term, an economy with a closed output gap is also achieving sustained full employment.
In the short term, deviations of output from potential output tend to coincide with movements in the labour market away from full employment. Tight labour market conditions – such as when the unemployment rate falls below levels consistent with full employment, creating a negative unemployment gap – tend to coincide with intensive use of capital inputs and a deviation of output from its potential level. This is a well-documented empirical relationship known as Okuns law. While estimates of Okuns law can be sensitive to modelling assumptions, the precise measures of spare capacity used, and structural change, a model-based estimate for Australia suggests that a negative unemployment gap of 1 percentage point tends to be associated with a positive output gap of 1¼ per cent, on average (Graph 1). In addition to the utilisation rates of capital and labour responding similarly to shifts in aggregate demand, the association in the graph also reflects the fact that labour inputs account for a large share of costs of production in the aggregate economy – that is, the labour component comprises a substantial portion of the output gap. But there is still some variation in the relationship, as the respective gaps can sometimes give different signals about the balance between demand and supply in the economy.
How does the output gap relate to inflation?
The output gap captures imbalances between aggregate demand and aggregate supply in the markets for goods and services and therefore the ability of, or need for, firms to adjust prices. When actual output and potential output diverge, inflation will typically move away from target. But over the medium-to-long term, a closed output gap sees inflation running at target.
Conceptually, the output gap is a better indicator of near-term inflationary pressures than measures of spare capacity in the labour market alone, but this might not be the case in practice. The relationship between a measure of spare capacity (either labour or activity) and inflation is known as the Phillips curve (Graph 2). Since most of the cyclical variation in output gap measures is driven by the labour market, the additional information – and uncertainty – in the output gap often does not provide more statistical explanatory power than labour market gaps in models of inflation.[5] Nonetheless, the relationships between the output gap, labour market and inflation provide frameworks that helps us measure potential output and the output gap.
Assessing potential output and the output gap
Potential output and the output gap cannot be observed directly, they can only be inferred from other information and so the estimates are uncertain. This is common to other so-called star variables, including the neutral rate of interest and the non-accelerating inflation rate of unemployment (NAIRU). The main focus of our assessment is short-to-medium term fluctuations of output from the level of potential output; that is, the extent to which the output gap moves over the business cycle.
The RBA uses a broad-based approach to assessing potential output and the output gap, which is consistent with peer central banks. Model-based estimates provide a core foundation for our assessment, as there are fewer survey-based indicators of spare capacity for product markets compared with factor markets (including the labour market). But the RBA does draw on a broad range of information beyond models – including capacity utilisation indicators, activity measures and inflation outcomes – to form an assessment of potential output and the output gap. Due to the close connection with the labour market, this includes indicators and model-based estimates that feed into our assessment of full employment. Our assessment is also guided by economic research and views from academics, market economists, government agencies, international organisations and other central banks.
How models inform our assessment
By exploiting historical relationships between economic indicators, models help us synthesise multiple pieces of information into a quantitative assessment of potential output and the output gap. There are four broad modelling approaches for estimating potential output: the production function approach, univariate filters, multivariate filters and structural models. Different modelling approaches have different strengths and weaknesses. The RBA uses a suite of models to capture a range of perspectives about potential output and the output gap. This includes a production function approach and two multivariate filters, which is broadly consistent with peer central banks and international institutions such as the Organisation for Economic Co-operation and Development (OECD) and International Monetary Fund (IMF).
Models require us to make a number of design choices and assumptions, and estimates can be highly sensitive to these features. In line with academic research and the approach of peer central banks, we use economic theory, statistical properties of the data and judgement to inform the structure of the models. However, given the uncertainty around modelling decisions, we also have alternative specifications for each model in the suite, which helps capture a broader range of possible outcomes.
Overall, the RBAs three different models emphasise different aspects of potential output and the output gap:
- The production function model draws out the various drivers of long-term economic growth and provides smooth estimates of potential output.
- The small multivariate output gap (SMOG) model relates the output gap to the cyclical components of inflation, unemployment and GDP, and results in estimates of potential output that fluctuate more than the production function estimates.
- The Joint-stars model is a large multivariate filter that combines features of the production function and SMOG models to jointly estimate long-term drivers of growth and cyclical output gap movements.
The SMOG and Joint-stars models bring together observed historical relationships and an economic structure that relates to the objectives of monetary policy (Figure 2). In contrast, the production function approach has less explicit connection to monetary policy objectives, but in focusing on the fundamental determinants of economic activity (like productivity and population) it provides a useful long-run benchmark for economic growth.
A production function model
A production function is an economic equation that expresses how much output is produced for given quantities of the factors of production. The RBAs production function model infers potential output by filtering slow-moving structural trends in the factors of production from observable data. These structural trends reflect the amount of labour, capital and MFP available in the economy when it is operating sustainably – so-called potential labour, potential capital and potential MFP. We combine these potential components using a production function to estimate aggregate potential output, which is then used to calculate an output gap (Graph 3).[6] Since it focuses on the structural trend of the factors of production, the production function approach is useful in highlighting the structural forces on the supply side of the economy that drive economic growth in the long term, such as structural trends in labour force participation.
Potential labour depends on the working age population and structural trends in the participation rate, employment rate and average hours worked. As the observed participation rate and average hours worked respond to the business cycle, we need to adjust these series using statistical techniques to obtain estimates of their underlying structural trends. The trend employment rate is calculated as one minus the NAIRU (the structural trend of the unemployment rate). The NAIRU is estimated separately as discussed in Ballantyne, Sharma and Taylor (2024). Since 2020, there have been large swings in population growth and, by extension, potential labour and potential output due to the pandemic (Graph 4). Despite current population growth remaining elevated, overall these movements have broadly washed out to return the level of the working age population to around its pre-pandemic trend.
We assume potential capital is equal to the capital stock.[7] This implies that when the economy is operating at its potential, the entirety of the net capital stock is being utilised at normal rates. Growth in potential capital has been relatively stable over the past few years (Graph 4). Recent growth reflects broad-based investment in the non-mining sector. The large decline in potential capital growth from 2013 to 2015 reflects structural shifts in the mining sector as it transitioned from investment – that is, building potential capital – to production and realising its potential capital.
Potential MFP reflects long-run technological progress in the economy, as well as additions to the skill level of the workforce or organisational expertise. MFP is difficult to measure in practice, so we estimate it as the residual in the production function (which is a standard approach). We estimate potential MFP by extracting the slow-moving trend of MFP using a statistical filter. Growth in potential MFP has declined over the past decade, consistent with a broader slowdown in productivity growth seen in other advanced economies (Bruno, Dunphy and Georgiakakis 2023).
While the production function approach abstracts from cyclical factors by statistical filtering, a limitation of the model is that it does not take direct signal from inflation. In addition, there is considerable uncertainty in filtering structural trends, particularly for MFP. Given this uncertainty, we have an alternate specification of the model that allows for more volatility in potential MFP.
A small multivariate output gap (SMOG) model
The SMOG model is a multivariate filtering model. This class of models infers unobservable variables from data on several measurable variables. The SMOG model estimates the trend and cyclical components of non-farm GDP by using non-farm GDP, inflation, nominal unit labour costs (a productivity-adjusted measure of wages) and the unemployment rate. The trend estimates provide the level of potential output, while the cyclical component provides the output gap.
The SMOG model infers the output gap from the cyclical movements in unemployment through an Okuns law relationship and inflation through a Phillips curve relationship. Nominal unit labour costs also help inform the cyclical movements in unemployment through a Phillips curve relationship based on wages growth (instead of inflation). For example, high inflation or wages growth may indicate building price pressure in an economy that is operating beyond its ability to sustainably produce goods and services. The model would then point to a positive output gap (GDP above potential output). Alternatively, high unemployment is suggestive of supply capacity exceeding demand in the economy; the model would read this as indicating a negative output gap.
The SMOG model also takes signal from GDP to jointly inform the output gap and the level of potential output. This is one key difference with the production function approach, which focuses on estimating potential output and then allows the output gap to be calculated separately. One consequence of this modelling decision is that the SMOG model tends to produce more volatile estimates of potential output.
The model allows us to calculate how much each observable variable contributes to movements in the estimates of the output gap. Unemployment is the largest contributor, driving about 35 per cent of movements in the estimate (Graph 5). This reinforces the importance of the labour market in assessing spare capacity in the Australian economy; however, other factors also contribute substantially, which is consistent with the balance between supply and demand in the economy depending on more than just the labour market. Inflation drives a little under 30 per cent of movements in the output gap estimates, and nominal unit labour costs contribute a further 10 per cent. The large inflation contribution indicates that the SMOG model is taking meaningful signal about the output gap from price pressures in the economy, which the production function approach does not incorporate directly. As such, potential output in this model corresponds more closely to the level of output the economy can sustain while maintaining low and stable inflation. Finally, GDP also drives a little under 30 per cent of movements in the estimated output gap.
Although the SMOG model has benefits in terms of its simplicity and its ability to take signal from inflation, the model is not without its limitations. Most importantly, the simpler structure we have imposed on potential output precludes the model from taking signal from the underlying drivers of the productive capacity of the economy, such as population growth and productivity growth. The model estimates are also sensitive to assumptions, so we maintain a few alternative specifications. Further details on this model are provided in Appendix A.
A jointly estimated star variable (Joint-stars) model
The Joint-stars model is a large multivariate filter model that jointly estimates many so-called star variables that together capture the unobserved determinants of the supply side of the Australia economy.[8] The model jointly estimates potential output, the output gap, the NAIRU and the neutral interest rate. It integrates features from both the SMOG model and the production function model; it takes signal from cyclical variables such as inflation, the unemployment rate and the cash rate, but also uses a production function that incorporates productivity, labour and capital to construct estimates of potential output and the output gap.
Potential output, the output gap, the NAIRU and the neutral interest rate are conceptually correlated and jointly estimating them can help to provide a consistent view of the economy. For example, short-term deviations of labour market conditions from full employment tend to coincide with deviations of output from its potential level, and the model takes this into account when estimating the NAIRU and potential output. Additionally, deviations of the real policy rate (the cash rate less expected inflation) from the neutral interest rate should reflect the stance of monetary policy and so affect the evolution of the output gap.
The Joint-stars model draws information from a larger set of economic indicators; cyclical variables such as inflation, the real cash rate and the unemployment rate, as well as factors of production like the capital stock and components of labour supply. The output gap is identified as the co-movement across a range of variables within the system, while potential output is aggregated from the structural trends in productivity, labour and capital using a production function. The model is structured so that each observable consists of a trend, a cyclical component and a measurement error (Figure 3).[9] The trend components are related through a production function, and trend labour is further decomposed into the trend working age population, the trend participation rate, the NAIRU and trend average hours worked. This is similar to the production function model. And like the SMOG model, the output gap is estimated by extracting the cyclical movements of capital, labour, inflation and the real cash rate.
An advantage of the Joint-stars model is its holistic estimation of trend and cyclical components, which are based on economic relationships rather than independently applying statistical filters (as in the production function model). By including many of the major macroeconomic indicators in a single system and estimating the trends and cyclical components jointly, we can assess the trade-offs among competing signals from these indicators in an integrated way. For example, when incoming data send different signals for the output gap – such as inflation and unemployment rising simultaneously – the model can determine how much weight to place on the different series to generate a coherent view. A limitation is that the model is complex, which can make the drivers of outcomes less transparent and so, at times, more challenging to reconcile the models estimates with expert judgements. The Joint-stars model also has alternative specifications that vary in their approach to accounting for the impact of the COVID-19 pandemic and their use of differing measures of real interest rates.
External estimates
The RBA also takes into consideration estimates of potential output and the output gap for Australia by third parties such as the IMF and the OECD. The IMFs main model is a simple multivariate filtering model similar to the RBAs SMOG model (Blagrave et al 2015), while the OECD uses a production function approach similar to the RBAs production function model (Chalaux and Guillemette 2019). Both external models produce annual estimates of potential output and the output gap. To obtain quarterly estimates we interpolate the annual estimates using a simple statistical technique. Additionally, the RBA collects market economists forecasts for potential output growth and current estimates of the output gap through the RBA survey of market economists, which is published in the statistical tables on a quarterly basis.
Pulling model-based estimates together
The RBA uses a suite of models to construct a range of estimates of potential output and the output gap (Graph 6). The suite includes the three models developed by the RBA, their alternative specifications, as well as some of the external estimates discussed above. The range of our model estimates covers the central estimates from each individual model (including alternative specifications) but does not capture uncertainty around each estimate. The RBA publishes the range of estimates of the output gap in the quarterly Statement on Monetary Policy.
How reliable are model estimates?
There are many different sources of uncertainty around model estimates, including uncertainty:
- from the data (measurement error, statistical noise and revisions)
- inherent in trying to infer unobservable variables through the movement of observed ones (filtering uncertainty)
- around the sizes of estimated relationships between variables (parameter uncertainty)
- over whether the structure and assumptions of a model correspond to the true properties of the economy (model uncertainty).
These different types of uncertainty can each be difficult to quantify and may interact with one another and compound, leading to each model estimate in our suite having substantial uncertainty around it.
A body of international academic research has investigated how large uncertainty is around estimates of the output gap and whether this affects how useful they are for informing policy. The results are mixed, depending on the technique used to estimate the output gap, and may also be country-specific. Most recent research indicates approaches that use a multivariate structure, such as the SMOG and Joint-stars models, produce more reliable estimates than univariate filtering.[10] However, the degree to which these estimates improve forecasting other variables such as inflation is still unclear.[11]
The use of a suite of models is one way to try to account for the substantial uncertainty inherent in modelling potential output and the output gap. Having multiple models with different underlying structures helps us determine a plausible range for the estimates to lie within. Moreover, if different model estimates and indicators agree with each other, that increases our confidence in the estimates of the output gap and potential output.
How economic indicators inform our assessment
In addition to model-based estimates, the RBA also draws on a range of labour market and non-market market indicators to inform our assessment of spare capacity. These indicators tend to focus only on specific segments of the economy, but nonetheless they help paint a fuller picture of spare capacity in the economy. For example, there are a wide range of labour market indicators used by the RBA to assess full employment (Ballantyne, Sharma and Taylor 2024). These same indicators are used to inform the RBAs assessment of potential output and the output gap. In addition, survey measures of capacity utilisation, and liaison with businesses provide insight into utilisation of existing labour and capital within businesses (Graph 7). This includes capital-intensive goods-related industries such as manufacturing, which gives a partial read on capital utilisation in the economy. Data on vacancies in residential property (reflecting spare capacity in housing) and other commercial structures in the economy also give a partial view of capital utilisation in the economy.
Measures of aggregate activity relative to their long-run trend can also provide some information on spare capacity in the economy. In particular, the deviation of GDP from its long-run trend provides a quick and simple assessment of spare capacity in the economy. Inflation is another potentially useful measure of spare capacity; however, imbalances of demand and supply tend to flow through to inflation with a lag and movements in inflation can also reflect temporary shocks that are not likely to affect potential output. These caveats need to be taken into account when the extent of spare capacity is being assessed on the basis of inflation.
Conclusion
Potential output and the output gap are closely related to the RBAs objectives of price stability and full employment. They provide useful economic frameworks to assess the extent of spare capacity in the economy, which is an important input into monetary policy. Staff at the RBA consider a wide range of inputs to form an overall assessment of potential output and the output gap, both of which are not directly observable. This includes using model-based estimates, labour market and non-labour market indicators, and other measures such as inflation outcomes. There is considerable uncertainty around model-based estimates of the output gap, and the RBA will continue to develop and refine its suite of models.
Appendix A: Details of the small multivariate output gap (SMOG) model
The SMOG model is the simplest of the three models described in this article and is detailed here as a concrete example. The description below reflects the model as currently designed, but development of the model is ongoing. The model consists of two types of equations: signal equations, which describe the movement of observable variables over time, and state equations, which govern the evolution of the unobservable variables the model must estimate.
Signal equations in the SMOG model link the level of non-farm GDP, inflation, wages and unemployment to the business cycle and structural trends in the economy as follows.
Non-farm GDP:
Unemployment rate:
Trimmed mean inflation:
Unit labour cost growth:
GDP evolves according to a trend (, potential output), cycle (, the output gap) and error term (to account for measurement error). Unemployment is similar, but we incorporate an extra term for the lagged unemployment gap () to allow the unemployment gap and output gap to diverge in a persistent (but not permanent) manner. This allows us to separate labour market tightness and broader spare capacity in the model.
The equations for the evolution of inflation and unit labour cost growth are more involved. They are generally similar to the equations described in Cusbert (2017), but we allow the relationship between inflation and its explanatory variables to differ before and after the inflation targeting period ( after inflation targeting). The SMOG model also differs slightly as we link trimmed mean inflation to the output gap, the measure of spare capacity most relevant for firms price-setting, and link unit labour costs to the unemployment gap, the measure of spare capacity most relevant for wage outcomes.
State variables in the SMOG model govern the evolution of the output gap, potential output, and the trend unemployment rate as follows.
Output gap:
Potential output:
Trend unemployment rate:
where is trend potential output growth.
This structure is similar to models used in academic literature and in other central banks (e.g. Blagrave et al 2015; Furlanetto et al 2023). All error terms are assumed to be uncorrelated and follow a normal distribution. The inflation equation includes a break in the variance from March quarter 1993 and the unit labour cost equation includes a break in the variance from March quarter 1984.
To separate movements in the output gap from potential output, additional restrictions can be imposed that determine how smooth potential output is relative to the output gap and whether GDP itself is measured with noise. The data provide limited information on the validity of these assumptions; that is, many different modelling assumptions are equally plausible. To account for this uncertainty, we produce three alternative specifications of the SMOG model that impose different assumptions about the dynamics of potential output and the amount of noise in the data. Each alternative represents a different judgement about the right balance of theoretical coherence and being consistent with the data. The alternatives can result in meaningfully different estimates of the output gap, spanning from around zero to around 1½ per cent for the December quarter 2023.
The model is estimated by maximum likelihood using the Kalman filter, from the June quarter 1978 to the current period. Evans, Moore and Rees (2018) provide an intuitive explanation of the estimation procedure. The model estimate of the output gap is shown in Graph A1, along with uncertainty bands that account for filtering and parameter uncertainty.
Endnotes
The authors are from Economic Analysis Department. They would like to thank Angelina Bruno, Jenny Lui, Martin McCarthy, James Morley, Matt Read and Tim Robinson, along with Dan Rees for his extensive work developing the Joint-stars model. [*]
Land is a common third factor of production. Human capital is sometimes considered a fourth factor of production, but is captured by productivity in the approach used here. Intermediate goods, such as component parts used in manufacturing of a final consumption product, are not considered a primary factor of production. [1]
The entire capital stock is not fully utilised all the time, even when output is at potential – for example, machines often require downtime for maintenance, equipment may be sitting idle while waiting to be moved to a different location, and retail and office buildings are typically not in use 24 hours a day. [2]
The RBA often uses the terms aggregate demand and aggregate supply (or simply, demand and supply) to refer to actual output and potential output, respectively, which is a simplification compared with the terminology used in this article. In that context, aggregate demand and aggregate supply should not be confused with the quantity of output demanded, or the quantity of output supplied, which should be equal, aside from any changes in inventories. [3]
For details on the role of full employment in monetary policy and how it is assessed, see RBA (2024b). [4]
Spare capacity, measured by a labour market gap or output gap, is a core component of most inflation models. For details of the RBAs approaches to modelling inflation, see Cassidy et al (2019). These models are distinct from the models that use inflation to infer the output gap, such as the SMOG model described in Appendix A. [5]
The RBAs production function assumes constant returns to scale, which means that if each of labour and capital is increased by 1 per cent then output is increased by 1 per cent. It also allows for the share of output that is earned by the providers of labour and capital (loosely speaking, households and businesses) to vary over time. Constant returns to scale is a simplifying assumption we make and is commonly used in production function models by peer central banks. [6]
Measured as capital stock net of depreciation. [7]
The Joint-stars model approach is similar to the US Federal Reserve Boards multivariate unobserved components model (Fleischman and Roberts 2011; Reifschneider, Williams and Wilcox 2015). [8]
The data used to estimate the Joint-stars model are non-farm GDP, trimmed-mean inflation, inflation expectation, average hours worked, the unemployment rate, the labour force participation rate, the working-age population, the capital stock, and the real cash rate. [9]
For background on the issues of reliability when estimating unobserved variables, see Orphanides and van Norden (2002). More recent literature indicates that multivariate filtering models can improve reliability (including in Australia): see Gruen, Robinson and Stone (2002); Jarociński and Lenza (2016); Alichi et al (2017); Rünstler and Vlekke (2018). [10]
For a discussion of how unreliable estimates can lead to worse inflation forecasts, see Orphanides and van Norden (2005). [11]
References
Alichi A, O Bizimana, D Laxton, K Tanyeri, H Wang, J Yao and F Zhang (2017), Multivariate Filter Estimation of Potential Output for the United States, IMF Working Paper WP/17/106.
Ballantyne A, A Sharma, T Taylor (2024), Assessing Full Employment in Australia, RBA Bulletin, April.
Blagrave P, R Garcia-Saltos, D Laxton and F Zhang (2015), A Simple Multivariate Filter for Estimating Potential Output, IMF Working Paper WP/15/79.
Bruno A, J Dunphy and F Georgiakakis (2023), Recent Trends in Australian Productivity, RBA Bulletin, September.
Cassidy N, E Rankin, M Read and C Seibold (2019), Explaining Low Inflation Using Models, RBA Bulletin, June.
Chalaux T and Y Guillemette (2019), The OECD Potential Output Estimation Methodology, OECD Economics Department Working Paper No 1563.
Cusbert T (2017), Estimating the NAIRU and the Unemployment Gap, RBA Bulletin, June.
Evans R, A Moore and D Rees (2018), The Cyclical Behaviour of Labour Force Participation, RBA Bulletin, September.
Fleischman CA and JM Roberts (2012), From Many Series, One Cycle: Improved Estimates of the Business Cycle from a Multivariate Unobserved Components Model, Board of Governors of the Federal Reserve System, Finance and Economics Discussion Series Paper No 2011-46.
Furlanetto F, K Hagelund, F Hansen and Ø Robstad (2023), Norges Bank Output Gap Estimates: Forecasting Properties, Reliability, Cyclical Sensitivity and Hysteresis, Oxford Bulletin of Economics and Statistics, 85(1), pp 238–267.
Gruen D, T Robinson and A Stone (2002), Output Gaps in Real Time: Are They Reliable Enough to Use for Monetary Policy?, RBA Research Discussion Paper No 2002-06.
Jarociński M and M Lenza (2016), An Inflation-predicting Measure of the Output Gap in the Euro Area, European Central Bank Working Paper Series No 1966.
Orphanides A and S van Norden (2002), The Unreliability of Output-Gap Estimates in Real Time, The Review of Economics and Statistics, 84(4), pp 569–583.
Orphanides A and S van Norden (2004), The Reliability of Inflation Forecasts based on Output-Gap Estimates in Real Time, Board of Governors of the Federal Reserve System, Finance and Economics Discussion Series Paper No 2004-68.
RBA (Reserve Bank of Australia) (2024a), Chapter 4: In Depth – Potential Output, Statement on Monetary Policy, May.
RBA (2024b), Chapter 4: In Depth – Full Employment, Statement on Monetary Policy, February.
Reifschneider D, W Wascher and D Wilcox (2015), Aggregate Supply in the United States: Recent Developments and Implications for the Conduct of Monetary Policy, IMF Economic Review, 63(1), pp 71–109.
Rünstler G and M Vlekke (2018), Business, Housing, and Credit Cycles, Journal of Applied Econometrics, 33(2), pp 212–226.
Treasurer and Reserve Bank Board (2023), Statement on the Conduct of Monetary Policy, 8 December.