Research Discussion Paper – RDP 2025-01 Are Investment Tax Breaks Effective? Australian Evidence
February 2025
1. Introduction
Business investment is crucial for economic growth and prosperity. It contributes to productivity, the key driver of living standards, by raising the amount of capital each worker has to use (capital deepening) and by embodying technological advances. Business investment also plays an important role in economic cycles: while it only accounts for around 11 per cent of GDP in Australia, it has long been recognised as being one of the most volatile components of output across the cycle (Kydland and Prescott 1982). Given these long- and short-term considerations, it is unsurprising that policymakers across the globe have in the past designed policies to promote investment both during ‘normal’ times and in response to adverse economic shocks.[1]
Australia has been no different. Over the past two decades numerous investment tax breaks (ITB) have been introduced in Australia to stimulate investment. These have been used as a structural tool to raise investment in normal times and as a cyclical tool to stimulate investment during downturns, such as the global financial crisis (GFC) and the COVID-19 pandemic. While the exact details of the various policies differed, each increased the (net present) value of depreciation expenses that firms could use to lower their taxable income. Hence, such policies generated a fiscal cost in terms of forgone tax revenue. For example, the tax incentives introduced in 2019 in Australia to support investment for medium-sized businesses were estimated to cost the government $400 million over the four years after its implementation (Parliament of the Commonwealth of Australia 2019). It is therefore important to understand the effects of these policies so that we can assess whether they are cost effective. Moreover, understanding the effectiveness of these policies can also help with forecasting by providing a sense of their macroeconomic effects.
In this paper, we examine the effectiveness of seven distinct policies implemented between 2009 and 2021 in stimulating additional investment in Australia. Examining a suite of policies under varying macroeconomic conditions for one country can allow us to better understand the macroeconomic context in which ITB policies can increase or fail to increase investment and which design features may lead to policy success or failure. We will focus on identifying additional investment that would not have happened in the absence of the policy distinct from subsidies of existing investment plans or shifts in the timing of investment.
High-quality, unique data make this possible. We use data from the Australian Bureau of Statistics (ABS) New Capital Expenditure (CAPEX) survey and administrative tax return data from the Australian Tax office, both accessed using the ABS Business Longitudinal Analysis Data Environment (BLADE). We use two different approaches to identifying the effects of these policies, both of which leverage the cut-offs for eligibility using firm size as captured by turnover: difference in differences (DD), and regression discontinuity design (RDD).
We find strong evidence that the policies implemented during the GFC increased investment, consistent with Rodgers and Hambur (2018). However, there is little evidence of other policies implemented during the 2010s, or the COVID-19 pandemic, having a substantive effect on firm investment.
These findings suggest that ITB can be an effective countercyclical tool, but their effectiveness is likely to be highly dependent on the nature of the economic downturn. For example, they seem more likely to be effective in a downturn caused by a dislocation in credit markets, compared to a pandemic where the economy is partly shut down. Beyond countercyclical policy, smaller policies targeted at structurally raising the level of investment during normal economic times seem of limited benefit. This does not mean that such policies will never be effective. For example, it may be that the policies we studied were too small to have an appreciable effect, or were implemented in periods of policy uncertainty. But the results do suggest the something would need to be different before we would expect a substantial investment response.
This paper makes a number of contributions to the literature. First, it adds to our knowledge of business investment incentives. By considering seven distinct policies with different rules and different eligibility criteria implemented under different macroeconomic conditions, but in a single country, it allows us to learn about heterogeneity of effects with respect to firm size, program rules and cyclical economic conditions.
Second, we provide evidence on the role of dividend imputation in dampening the effects of ITB, building on Rodgers and Hambur (2018). These findings contribute to the debate on the ‘old’ and ‘new’ views of corporate finance. We find that unincorporated businesses, who are not subject to imputation, respond far more strongly to ITB. This is consistent with the old view where the marginal source of funds is external, rather than internal. However, for the GFC policy, incorporated companies also responded to ITB, though by less than unincorporated businesses. This suggests that either ITB affected investment through non-traditional channels, like easing financing constraints that may have been particularly binding during the GFC, or there may be heterogeneity amongst incorporated firms in their financing approach. Some may fund investment from retained earnings (the new view), whereas others may be more dependent upon external funding. If this funding is from non-domestic sources, dividend imputation may be less relevant and ITB policies may decrease the cost of capital and stimulate investment.
Third, we provide the first comprehensive study of business investment incentives in Australia, expanding the scope of policies beyond those previously considered. Finally, we provide some initial evidence that corporate tax rate cuts for small businesses appear to have stimulated additional investment. This is an area for further detailed analysis.
The rest of this paper is set out as follows. Section 2 gives an overview of the policies used in Australia. Section 3 gives an overview of the literature on the effect of investment incentive policies. Section 4 provides an overview of our data. Section 5 outlines our identification strategies and Section 6 presents our results. Sensitivity analysis is included in Section 7 and Section 8 concludes.
2. Overview of Investment Incentives in Australia
The ‘modern’ system of turnover-based investment incentives started in 2007, with the unification of small business tax concessions under a common turnover test of $2 million. Amongst other benefits, these changes enabled businesses that satisfied the turnover test to access instant deductions or ‘write-offs’ for depreciating assets costing less than $1,000 and allowed the cost of most other assets to be pooled and depreciated at an accelerated rate. Since then, there have been seven important changes to business investment incentives (see Table 1). Three of these incentives – the GFC tax credit and two COVID-19-related policies – were designed as countercyclical stimulus in response to adverse economic shocks. The other four policies expanded eligibility for ITB in terms of asset value and firm size in order to ‘structurally’ raise the level of investment to support productivity.[2] In all cases the ITB applied only to machinery & equipment, while buildings were excluded. We use this exclusion both as a placebo test, and to differentiate ITB from the effects of corporate tax rate cuts that coincided with some ITB policies.
Policy | Turnover limit | Policy type | Asset limit |
---|---|---|---|
GFC 2009 | $2 million | Extra deduction | Uncapped |
Small 2012 | $2 million | Instant asset write-off | $6,500 |
Small 2015 | $2 million | Instant asset write-off | $20,000 |
Medium 2016 | $10 million | Instant asset write-off | $20,000 |
Medium 2019 | $50 million | Instant asset write-off | $30,000 |
COVID 2020 | $500 million | Instant asset write-off | $150,000 |
COVID 2021 | $5 billion | Instant asset write-off | Uncapped |
Sources: See Appendix A. |
The first three policies relate to small businesses with a turnover of under $2 million.
The GFC investment tax credit (GFC 2009) offered a deduction for new business investment in addition to normal depreciation deductions. When the policy was first announced in December 2008 it afforded all businesses an extra deduction of 10 per cent (Swan 2008), which was then raised to 30 per cent in the March quarter of 2009 (Swan 2009a). In May 2009 the policy was further changed, which allowed for a differentiated benefit based on firm size (Swan 2009b). Small businesses were able to deduct a bonus 50 per cent for investment made from 13 December 2008 to 31 December 2009 costing at least $1,000 (with this applying retrospectively to investments made before the adjustment to the policy). Other businesses were allowed to deduct a bonus 30 per cent bonus on investments over $10,000 for investments committed to before 30 June 2009, and a 10 per cent bonus deduction for those committed to during the second half of 2009. We focus on the second half of 2009 for our analysis, when the subsidy rates differed substantially.
The small business incentive 2012 (Small 2012) gave businesses with a turnover up to $2 million an increase in the instant asset write-off threshold from $1,000 to $6,500 from 1 July 2012. Legislation enacting the increase from $1,000 to $5,000 was contingent on the passage of the Mineral Resource Rent Tax legislation and the further increase to $6,500 was contingent on the passage of legislation related to emissions reductions. This increase in the asset threshold was subsequently unwound from 1 January 2014 and reset to $1,000 until 2015 when the next set of incentives were introduced.
The small business incentive 2015 (Small 2015) increased the asset threshold from $1,000 to $20,000 from 12 May 2015 to 30 June 2017. However, small businesses also received a 1.5 percentage point tax cut from 1 July 2015, so firm behaviour during this period will be affected by both the instant asset write-off and the corporate tax rate cut (see Table 2). We focus mainly on the period up to 31 December 2015 due to the announcement of further ITB in 2016.
Small business rate | Small business threshold | |
---|---|---|
2014/15 | 30 per cent | $2 million |
2015/16 | 28.5 per cent | $2 million |
2016/17 | 27.5 per cent | $10 million |
2017/18 | 27.5 per cent | $25 million |
2018/19 | 27.5 per cent | $50 million |
2019/20 | 27.5 per cent | $50 million |
2020/21 | 26 per cent | $50 million |
2021/22 | 25 per cent | $50 million |
Sources: See Appendix A. |
The next two policies extended instant write-off benefits to medium-sized businesses.
The expanded business incentive 2016 (Medium 2016) was announced in early 2016. It extended the instant asset write-off to businesses with a turnover of up to $10 million, for expenditure up to $20,000. It came into force from mid-2016. The same package also gave these businesses a tax rate cut from 30 per cent to 27.5 per cent (Table 2).
The medium-sized business incentive 2019 (Medium 2019) extended the instant asset write-off to businesses with a turnover of up to $50 million, allowing them to fully expense capital investments made between 2 April 2019 and 30 June 2020 for assets that cost less than $30,000. However, this policy effectively ended on 11 March 2020 as the Australian Government introduced a new investment incentive that started on 12 March 2020 in response to the COVID-19 pandemic.
The large businesses incentive (COVID 2020) was available from 12 March 2020 to 6 October 2020. Under this policy, larger businesses were given access to a range of new incentive measures. Firstly, businesses with a turnover of up to $500 million were given access to an instant asset writeoff for capital assets costing less than $150,000. The assets covered included past purchases, so it covered assets purchased after 2 April 2019. Second, an accelerated depreciation rate of 50 per cent was available to businesses in the year the asset was purchased for assets costing more than $150,000 purchased in 2019/20 and 2020/21.
Full expensing measures for very large businesses (COVID 2021) were available from 6 October 2020. They allowed businesses with a turnover of up to $5 billion to immediately deduct the full cost of investment without any dollar limit.
It is difficult to measure the impact of these policies on firms' cost of capital. This is because we do not observe the proportion of assets used by each firm that falls below the asset cost cap, nor do we observe the tax life of these assets (with longer-lived assets benefiting more from instant write-off). For example, firms that primarily use inexpensive assets (costing less than the cost cap) with long tax lives would benefit more than firms that use expensive assets (costing more than the cost cap) or firms that use assets with short tax lives. However, we can make some general observations in relation to generosity. The GFC investment tax credit was the most generous of all the policies because asset values were uncapped, and it allowed businesses to ‘double dip’ – they were able to access an extra deduction, as well as normal depreciation allowances in future years. In contrast, all other tax incentives merely allowed a bring-forward of depreciation deductions from future years, with the main benefit being a timing benefit. The COVID 2020 and 2021 policies were the most generous of these bring-forward policies because the asset limits were much higher or uncapped and they covered businesses with much higher turnovers than previous policies. The 2015 and 2016 policies were not only less generous, but were rendered less valuable by corporate tax rate cuts that were implemented at the same time. Lower tax rates imply that each dollar of deduction is worth less in foregone tax.
3. Literature
Hall and Jorgenson (1967) first analysed the impact of tax incentives on business investment and the intervening (nearly) sixty years have seen many economies implement large-scale business tax reforms with an aim of stimulating investment, both in normal times and as a countercyclical tool. Questions around the efficacy and cost effectiveness of such measures remain a live part of the policy debate.
Investment tax incentives are generally thought to affect investment through two channels. The ‘traditional’ user cost channel, and the ‘non-traditional’ financing frictions channel.
The user cost channel postulates that firms will invest as long as the marginal benefit of that investment is higher than the cost. Increasing tax deductions for investment expenditure reduces the costs, often referred to as the user cost of capital (UC), by increasing the present value of depreciation allowances that firms can use to lower their tax. This can be seen using a measure of the the UC derived by Hall and Jorgenson (1967):
where P is the real price of investment goods, M is the weighted average cost of funds (debt and equity), and is the business tax rate. Z is the ratio of the present value of future depreciation allowances, relative to the initial purchase price of the asset. These allowances permit businesses to deduct the cost of their capital investment from their taxable income over several years, with the period approximately reflecting the economic life of the asset. By increasing or bringing forward the timing of these allowances, investment incentives can increase Z and so lower the UC.
A permanent policy change that lowers the UC leads to a burst of investment as firms adjust to their new higher desired capital stock.[3] Investment may remain higher going forward as firms invest to maintain the higher capital stock. Temporary policies may have a larger and more immediate effect as firms bring forward investment to the current period when it is cheaper (Abel 1982). However, empirically this bring-forward effect may be hard to detect, particularly in the case of long-lived assets, as the future ‘hole’ in investment could be spread over many years (House and Shapiro 2008).
Imputation systems such as exist in Australia change these costs and benefits. Under Australia's imputation system, company tax operates as a withholding tax. When dividends are issued to individuals, those individuals receive a credit for the company tax paid. Individuals are taxed annually on all of their income and can claim the credits for tax already paid. In particular, as they provide the end owner of the company with a tax offset for the tax paid by the company, they can ‘wash out’ the benefits of the depreciation allowances. Dividend imputation has been removed in many countries, including the United Kingdom and New Zealand, as corporate tax rates have been lowered. They remain an important feature of the Australian system.
Under the old view of corporate finance where businesses fund new investment through raising equity, the UC can be augmented for imputation as in Officer (1994):
Imputation is captured by the term , which measures the value of a dollar of tax paid at the company level to shareholders. This value reflects the fact that, under dividend imputation, tax paid at the company level is a credit for personal income tax for resident shareholders. If all shareholders are domestic (and in the presence of full refundability of imputation credits), as may be the case for small firms, the value of is 1 – tax paid at the company level lowers shareholders' taxes dollar-for-dollar. In this case increases in Z do not affect the cost of capital nor do they affect investment. If some shareholders are non-residents, as may be the case for larger firms, may be below 1. In contrast, the new view posits that the marginal source of funds for firms is retained earnings. In this case the imputation system has little influence on the UC.
These two views of corporate finance lead to two very different conclusions regarding whether firms that are subject to imputation will respond differently to the ITB than those which are not. Under the old view, firms not subject to imputation (i.e. unincorporated businesses) should respond more strongly. Under the new view, both firm types should respond similarly.[4] As such, ITB can be used to assess which view of corporate finance is most consistent with the empirical evidence.
Numerous studies have examined similar ITB overseas. Empirical studies on the United States (House and Shapiro 2008; Zwick and Mahon 2017) and the United Kingdom (Maffini, Xing and Devereux 2019) have found strong investment responses to tax incentives (and associated decreases in the user cost of capital).
House and Shapiro (2008) test if businesses increased their expenditure on longer-lived assets, and whether there was a change in aggregate investment behaviour in response to a change in policy. To look at asset lives, they use data from the Bureau of Economic Analysis to construct a quarterly panel of investment quantities and prices by asset type. They match 36 asset types to the Internal Revenue Service (IRS) depreciation schedule to track eligible and ineligible investment as well as short/long asset lives between 1959 and 2005. A structural model is then estimated to provide a baseline for investment activity, GDP and employment without the bonus investment. House and Shapiro then compare forecast errors across asset types. A strong response to the tax break was found in terms of the investment composition, with an investment supply elasticity of between 10 and 20 per cent. However, only modest increases in aggregate output and employment were found, as the policy narrowly benefited a small subset of investment goods.
Zwick and Mahon (2017) study the same bonus depreciation policy as House and Shapiro (2008) but use detailed IRS corporate tax return data that include information on investment in equipment and structures. In 2008, the sample represented about 1.8 per cent of the total population of 6.4 million C and S corporation returns. In each non-bonus year, the authors compute the share of eligible investment a firm reports in each asset life and property category. They then compute the present discounted value of one dollar of deductions for eligible investment, and combine this with the eligible investment shares to construct an industry-average deduction value. As the policy raises the net present value of longer-lived investment by more, they use this variation in asset lives in a DD regression to identify the policy effect. They find evidence that the policy affected investment. They also find evidence that the policy had a larger effect on the investment of firms that appeared to be financially constrained, indicating that the policy worked at least partly by loosening constraints.
Maffini et al (2019) study UK corporate tax returns to analyse the impact of a 2004 change in policy. It allowed more firms to access small business depreciation concessions, which took the form of a 40 per cent depreciation rate compared to the 25 per cent available for larger companies. Similar to what we do, they exploit this exogenous change in the qualifying thresholds and compare the response for companies newly qualified for the greater depreciation deductions to those that remain ineligible. Consistent with the US studies, Maffini et al find a substantial positive effect of the more generous depreciation allowances on firms' investment. Relative to the control group, the depreciation allowances raised the investment rate in eligible assets of newly-qualified companies by between 2.1 and 2.5 percentage points within three years of the change.
Zhang, Chen and He (2018) and Liu and Mao (2019) analyse the effect of a permanent 17 per cent tax credit for fixed investment for six industries in northwest China between 2004 and 2009. Using geographical restrictions to identify the policy effect, Liu and Mao (2019) find that the tax credit raised fixed investment of eligible firms by 28 per cent on average during 2004–2007, relative to 2001–2003, corresponding to a user cost elasticity of 1.84. The strong response by firms found by Liu and Mao (2019) is consistent with Zhang et al (2018), who found that, on average, the reform raised investment of the treated firms relative to the control firms by 38.4 per cent.
Australian evidence is sparse, partly due to data availability issues in the past. Rodgers and Hambur (2018) analyse the effect of tax breaks for machinery and equipment during the GFC (this is the first of the seven reforms that we consider in this paper). They use RDD and DD methods around the turnover threshold to compare the investment of small and large businesses using business tax microdata and unpublished survey data from the ABS.
Consistent with the difference in corporate taxation regimes (i.e. classical taxation in the United States and dividend imputation in Australia) and the old view of corporate finance, Rodgers and Hambur (2018) find a lower responsiveness of investment to the ITB compared to Zwick and Mahon (2017), as measured by the elasticity of investment. However, they find a much larger elasticity, more in line with the US studies, when focusing on unincorporated businesses who face a classical taxation regime similar to US corporations. They find some evidence that incorporated companies also responded. Given that the policy was targeted at smaller companies, who have very few non-resident shareholders, they argue that under Australia's dividend imputation system, equals one for these companies. They conclude that their findings suggest that the policies worked partly by relaxing financing constraints.[5] Our results presented below are consistent with this previous research on Australia which only examined one of the seven policies that we consider.
4. Data
We merge survey data from the ABS with business taxation administrative data from the Australian Taxation Office (ATO) to construct our sample. This provides us with two different sources of investment data. The first is the CAPEX survey. This is a survey of around 10,000 firms each quarter. Consistently large investors as determined by the ABS (large mining companies, banks and a few other large firms) are surveyed every quarter, while the remainder are stratified by industry, location and employment size, drawing from the administrative business register. The advantage of the CAPEX data is that they provide investment by asset type: machinery & equipment (assets that are generally eligible for the investment incentives) and buildings & structures (assets that are not generally eligible for investment incentives). However, the sample is too small to use for our RDD. Once combined with other taxation data (see below) we have a panel with around 90,000 businesses and around 667,000 observations spanning the period 2000/01 to 2018/19. See Appendix B for summary statistics of the data that we use to analyse each policy.
Our second source of investment data is capital expenditure reported in firms' business activity statements (BAS). These are available quarterly covering the period 2001–2021. As these data are reported by almost all firms, the sample is very large.[6] However, eligible and ineligible investment are not separately reported in these data.
For the analysis, we consider both the intensive and extensive margins. The intensive margin is simply the (natural) log of investment. For the extensive margin, our dependent variable is the log odds ratio for the share of firms investing, calculated at the industry division–turnover category level, as in Zwick and Mahon (2017).[7] The extensive margin is particularly relevant for the analysis of small business policies, as only around 25 per cent of small firms (< 20 staff) invest each quarter based on tax data, compared to around 80 per cent of large firms (200+ staff). In our RDD design we focus only on the intensive margin.
These investment data are combined with other tax data available on an annual basis. These tax data contain a rich set of information on business type, turnover, assets, liabilities and ownership structure. The tax data also include details of which businesses are connected to others through a business group identifier. This aggregation is useful since the legislated turnover tests are on an ‘aggregated turnover’ basis, which includes the annual turnover of not just the primary business but also of any other controlled or affiliated businesses in related business groups. Lastly, the tax data allow us to differentiate between different legal structures: companies, partnerships, trusts and individual entities. These distinctions are important in the Australian context given companies are subject to a dividend imputation regime, which may limit the value of ITB to the firm.
For the analysis, we follow Rodgers and Hambur (2018) and exclude the mining sector to abstract from any effects from the mining boom. The public sector is removed (based on the 2008 ABS Standard Economic Sector Classifications of Australia). The finance sector is also excluded from the RDD, due to conceptual difficulties measuring investment in the tax data for the sector.
5. Identification Strategies
To identify any effect of the ITBs we use both a DD approach and an RDD approach. As explained below, each identify different impacts and rather than focus on one, we use both to see if there are any effects of the ITB policies. Our assessment of the impact of the policies is based on an examination of the combined evidence across these approaches.
5.1 Difference in differences
Our first approach is a DD model. We exploit the timing of the policies, as well as the exogenous qualifying turnover thresholds, to identify the impact of the policy, comparing qualifying and other firms during and outside of the policy period. The treatment and control groups are summarised in Table 3.
Policy | Turnover | Dates | |
---|---|---|---|
Treated | Control | ||
GFC 2009 | < $2 million | $2 million to $5 million | Sep 2009 to Dec 2009 |
Small 2012 | < $2 million | $2 million to $5 million | Sep 2012 to Dec 2013 |
Small 2015 | < $2 million | $2 million to $5 million | Sep 2015 to Dec 2015 |
Medium 2016 | $2 million to $10 million | $10 million to $20 million | Sep 2016 to Jun 2017 |
Medium 2019 | $10 million to $50 million | $50 million to $60 million | Jun 2019 to Mar 2020 |
COVID 2020 | $50 million to $500 million | $500 million to $600 million | Mar 2020 to Jun 2020 |
COVID 2021 | $500 million to $5 billion | $5 billion to $6 billion | Dec 2020 to Jun 2021 |
Our regression takes the following form:
where Invi,j,t is our measure of investment (intensive or extensive margin) for machinery & equipment for business i in industry j in period t, is a dummy that equals one in the periods of operation of the relevant policy, and dummy is equal to one for businesses that meet the relevant turnover test. We also include a number of controls in the model to help with identification and with precision. First, we include firm fixed effects to capture time-invariant firm-specific factors influencing investment. We also include additional time-varying, firm-level controls to capture other factors that could be influencing investment, captured in Xi,j,t. Specifically these are full-time equivalent employment and deciles of the distribution of firm turnover. We also include a vector of quarterly dummies, , intereacted with dummies for the treated and control groups, to control for seasonal patterns that might affect investment. And we include industry*time fixed effects (INDj × Dt) to capture heterogeneous industry-level trends and conditions, which might be particularly important during the GFC and COVID-19 episodes as different industries were differentially exposed to these shocks. As such, we are effectively comparing small and large businesses within the same industry.[8] Standard errors are clustered at the firm level for intensive margins and industry levels for extensive margins.[9]
To define a firm's eligibility we turn to the tax code. The code states that a firm is small if their revenue in a period is under a certain threshold. There are several different periods that can be used for this assessment under the tax code eligibility rules:
- revenue in year t;
- revenue in year t – 1; or
- revenue in year t – 2 if the firm had a reasonable expectation when it undertook the investment that revenue in year t would be below the threshold.
This final category, which combines revenue in year t – 2 with expectations about revenue in year t, was designed for firms that may have had a windfall gain in year t – 1 and for whom revenue in year t – 2 is more ‘representative’ of their normal revenue. Given firms may not know time t sales during the year when investment decisions are made, they are allowed to rely on a reasonable expectation. We don't use firms that rely on this rule for the DD regressions, but they are used for the RDD identification in combination with information about actual turnover in time t and t – 1.
For the purposes of the DD regressions, we use revenue in year t – 1 in assessing whether the firm qualifies for the policy, as in Rodgers and Hambur (2018). As discussed below, for our RDD regressions we take a different approach, exploiting the third category noted above to get very tight identification.
One concern with identifying the effects of these ITB is that some coincided with changes in the corporate tax rate for firms based on the same threshold. Table 2 shows the changes in the tax rate over time. Of particular concern, the changes in 2015/16 and 2016/17 coincide perfectly with the 2015 and 2016 ITB. The reduction in the corporate tax rate would have simultaneously lowered the value of the ITB for companies, and potentially stimulated additional investment. As such, this may affect our estimated effects for these policies. To assess the degree to which this might be affecting our results, we provide some additional tests for outcomes across assets that were and were not eligible for the ITB (see Section 7.1).
5.2 Regression discontinuity design
Another way to exploit the exogenous eligibility threshold is to use a RDD approach. Similar to DD, this involves looking at differences in outcomes for eligible and ineligible firms. But instead of comparing outcomes more broadly between these groups, it focuses on an area around the policy threshold cut-off, comparing outcomes for firms just above and just below the threshold to determine whether there is a discontinuity at the threshold.
The advantage of this approach is that the identification is sharper and it requires relatively weak assumptions – namely, that in absence of treatment there would be no discontinuity at the threshold.[10] However, the approach requires more data and the identified effect, while sharp, is very local and can be hard to extrapolate to an aggregate effect. Given the data requirements, we use the BAS data described above for the RDD estimates.
For identifying the relevant cut-off we adopt the approach of Rodgers and Hambur (2018). This approach focuses on firms that would qualify based on whether their turnover was above or below the threshold in year t – 2. This helps to minimise any possibility that firms could manipulate their way into treatment.
To isolate these firms, we remove firms that could qualify for the incentives based on their incomes in tax year t or t – 1 (i.e. businesses with turnover under the turnover limit in those years). Amongst the remaining firms, the only firms that could use their income in t – 2 to meet eligibility are those that had a reasonable expectation that their income in t would be below threshold. The tax rules note that the best way to assess this is revenue in t – 1 (Australian Taxation Office undated). That is, only firms with revenue a little bit above the threshold in t – 1, could say they reasonably expect to be below the threshold in t.
To meet this final requirement, we remove firms with income in t – 1 above a certain threshold. We use a ceiling of 1.25 times the threshold. This is equivalent to Rodgers and Hambur (2018) using a ceiling of $2.5 million for the $2 million threshold. While somewhat arbitrary, Rodgers and Hambur found estimates tended to be quite robust to the exact choice of threshold. The selection rules we use to choose our estimation sample are summarised in Table 4.
Turnover in t – 2 | Turnover in t – 1 | Turnover in t | |
---|---|---|---|
GFC 2009, Small 2012, Small 2015 | |||
Dropped from sample | < $2 million | < $2 million | |
Policy | $2 million – η to $2 million | $2 million to $2.5 million | |
Control | $2 million to $2 million + η | $2 million to $2.5 million | |
Medium 2016 | |||
Dropped from sample | < $10 million | < $10 million | |
Policy | $10 million – η to $10 million | $10 million to $12.5 million | |
Control | $10 million to $10 million + η | $10 million to $12.5 million |
In summary, we focus on the subset of firms who had turnover above the threshold in year t, and between the threshold and 1.25 times the threshold in year t – 1. This group would qualify for the ITB only if their turnover was below the threshold in t – 2, but otherwise would not. So we have a clean eligibility cut-off at the threshold to separate eligible and non-eligible firms based upon their revenue at time t – 2.
The RDD can be thought of as estimating a local polynomial model on either side of the threshold to estimate the conditional mean precisely on each side of the threshold.
As noted, for the regression we focus on a small region either side of the cut-off. To select this region we implement the automatic bandwidth selection procedure proposed by Calonico, Cattaneo and Titiunik (2014) with a triangular kernel. We also also use their bias adjusted standard errors.[11] The results are robust to selecting different bandwidths. For all RDD regressions we cluster standard errors at the firm level.
6. Results
6.1 Macro stabilisation policies
First, focusing on the graphical evidence for the DD approach (Figure 1), we see clear evidence of an effect for the GFC policies: investment for both groups followed a similar pattern, before diverging in the second half of 2009 when the differential tax incentive was in place (dark shading). This was particularly evident at the intensive margin.
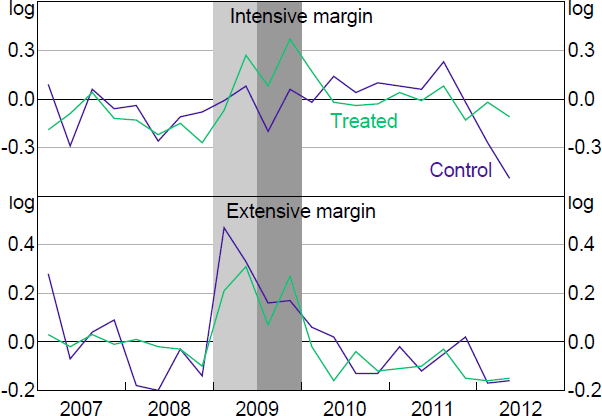
Notes: Controls for employment size, turnover decile, industry division time trends and seasonal size dummies. Light shading indicates period where treated and control firms received similar investment tax break. Dark shading indicates period with different tax breaks, which is the treatment period for regressions.
Sources: ABS; Authors' calculations.
In contrast, focusing on the COVID-19 policies (Figure 2), we can see that over this period all firm size groupings follow a broadly similar investment path. Given the overlapping nature of these policies we visualise them differently to the other DD results. We show investment for each group, where the shaded colour corresponds to the largest firm now eligible for a tax concession. If the policies were affecting investment, we would expect to see each line pick up, relative to the line for the next size group, when we enter the relevant shaded period. However, there is no evidence of an increase in investment as each group becomes eligible for the incentive, suggesting little evidence of an effect from the policy. Instead, all lines follow fairly similar paths.
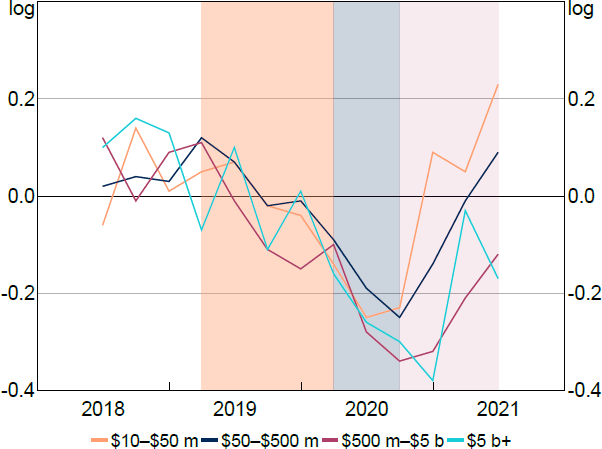
Notes: Size based on income in 2018/19. Controls for employment size, turnover decile, industry division time trends and seasonal size dummies. Shaded region indicates largest firms that were eligible for investment incentives.
Sources: ABS; Authors' calculations.
These findings are borne out in the regression analysis (Table 5). As with Rodgers and Hambur (2018), the GFC tax incentive seems to have successfully raised investment on the intensive margin, with treated firms spending substantially on eligible investment. The effects are also larger for unincorporated businesses, consistent with previous findings and the fact that the imputation system should wash out much (if not all) of the benefit for small domestically owned companies. This would also be supportive of the old view of corporate finance. Nevertheless, given there is still a response amongst companies it suggests that either the ITB is working through other channels, like easing cash flow constraints, or that there might be some heterogeneity in firm funding or ownership. For example, some firms may rely on internal funds or have overseas owners (who do not receive value from imputation credits) and so the ITB affects their cost of capital.
In contrast, we do not find statistically significant evidence that the COVID policies increased investment.[12] There is even some small evidence for a negative effect at the intensive margin for the 2020 policy but the confidence interval is wide.
Intensive margin | Extensive margin | ||||||
---|---|---|---|---|---|---|---|
All firms | Companies | Unincorporated | All firms | Companies | Unincorporated | ||
GFC 2009 | 0.456*** (0.136) |
0.414** (0.168) |
0.870*** (0.238) |
0.207* (0.113) |
0.176 (0.132) |
0.274** (0.139) |
|
Observations | 7,226 | 4,660 | 2,520 | 46,069 | 24,758 | 21,293 | |
COVID 2020 | −0.291* (0.150) |
0.138 (0.219) |
|||||
Observations | 17,701 | 25,643 | |||||
COVID 2021 | 0.404 (0.267) |
0.546 (0.449) |
|||||
Observations | 5,040 | 6,147 | |||||
Notes: Mining is excluded. Regressions include controls, except for COVID 2021 intensive margin results which are estimated without controls for employment and turnover decile due to small sample sizes. ***, ** and * denote statistical significance at the 1, 5 and 10 per cent levels, respectively. Cluster robust standard errors in parentheses. |
Turning to the RDD approach, graphically we see a clear shift down in investment after the threshold for the GFC policy (Figure 3). This is evident whether we focus on average investment within small sales buckets, or a line of best fit using a linear or local polynomial model. This is also evident in the regressions, with a large fall in investment above the threshold, suggesting the ITB raised investment for eligible firms (Table 6).[13]
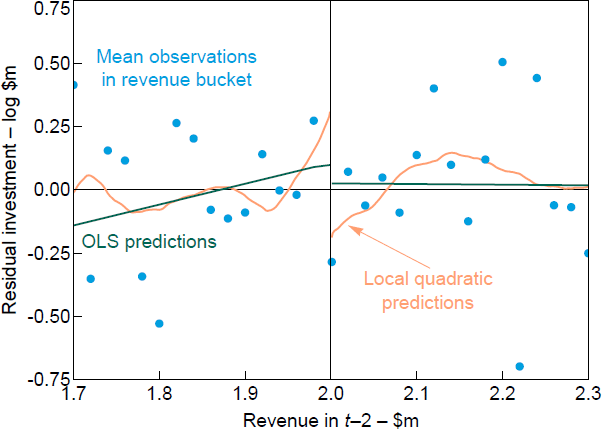
Note: Residual from a regression of log investment on industry fixed effects.
Mar 2009 | Jun 2009 | Sep 2009 | Dec 2009 | Mar 2010 | |
---|---|---|---|---|---|
All firms | |||||
Beta | −0.114 | 0.006 | −0.404** | −0.036 | −0.022 |
Robust p-value | (0.49) | (0.79) | (0.02) | (0.82) | (0.88) |
Bandwidth | 328,163 | 326,907 | 246,555 | 467,689 | 508,608 |
Effective observations | 2,106 | 2,421 | 1,692 | 2,875 | 2,713 |
Companies | |||||
Beta | −0.127 | 0.177 | −0.154 | −0.036 | −0.155 |
Robust p-value | (0.51) | (0.30) | (0.35) | (0.78) | (0.41) |
Bandwidth | 374,530 | 353,778 | 313,475 | 368,003 | 560,025 |
Effective observations | 1,332 | 1,490 | 1,173 | 1,457 | 1,685 |
Unincorporated | |||||
Beta | 0.005 | −0.292 | −0.570** | −0.032 | 0.187 |
Robust p-value | (0.96) | (0.31) | (0.03) | (0.96) | (0.45) |
Bandwidth | 315,877 | 366,361 | 275,067 | 385,634 | 466,038 |
Effective observations | 867 | 1,084 | 813 | 1,089 | 1,081 |
Notes: Bolded quarters are policy periods. ***, ** and * denote statistical significance at the 1, 5 and 10 per cent levels, respectively. |
Interestingly, based on the RDD, it appears that most of the effect occurred in the first quarter of the policy (i.e. September quarter 2009), with very little evidence of an effect in the second quarter. In some sense this is surprising, as it means there was a long period between the outlay and receiving the tax benefit. The latter only happened when the company lodged its return at the end of the tax year. The finding could reflect an announcement effect. However, it could also reflect uncertainty around further changes to the policy, given the nature of what the policy would be had changed several times over the preceding year in various announcements.
The lack of a offsetting effect in the quarters following the end of the policy suggests either that the policy led to an increase in investment, rather than just a bring forward of future investment, or that the bring forward effect was quite drawn out and not readily observable. These results are consistent with Rodgers and Hambur (2018). The size of our estimates differ from theirs because we use an indicator variable to identify the 2009 GFC tax credit in contrast to their measure of the intensity of treatment. Our modelling choice is for consistency within our paper across the different policies we consider.
Unfortunately we cannot asses the COVID polices using RDD as the sample of firms close to the thresholds for the COVID-19 policies is too small to implement RDD.
The difference in the results across the GFC and COVID policies may reflect the differences in the types of economic shocks during these periods. The fact that the GFC was a financial shock that did not severely slow real activity in Australia (unemployment did not rise above 6 per cent), but may have impacted credit supply, could have meant that generous measures which increased access to funds through the tax system were more likely to be successful. In contrast, the COVID-19 shock resulted in lockdowns across Australia as well as disruptions in machinery and equipment production globally. These two factors together may have hampered the ability and incentives for business to source and install new capital purchases in order to take advantage of the investment tax breaks.
6.2 Structural small business policies
We can also compare the 2009 policy results with other small business policies to interrogate the differences in effects across the policies. For both the 2012 and 2015 policies there is very little graphical evidence of a policy effect in the DD set-up: investment for the treated and control groups follow similar paths pre treatment, with no change post treatment (see Figures 4 and 5).
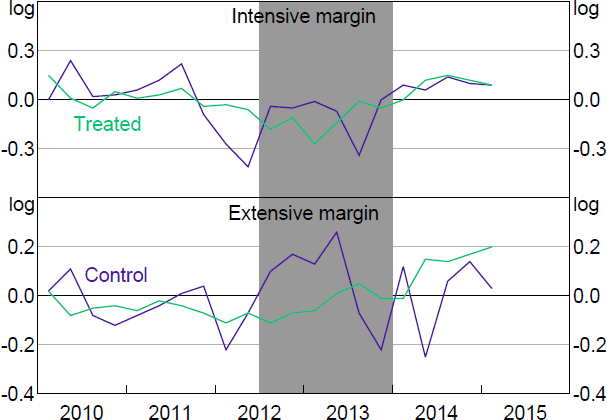
Notes: Controls for employment size, turnover decile, industry division time trends and seasonal size dummies. Shading indicates the treatment period for regressions.
Sources: ABS; Authors' calculations.
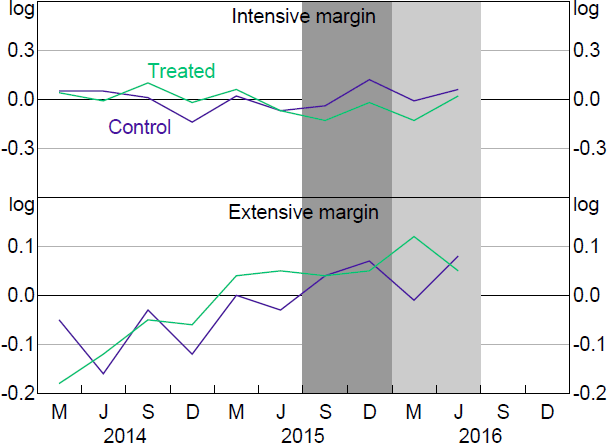
Notes: Controls for employment size, turnover decile, industry division time trends and seasonal size dummies. Dark shading indicates treatment period for DD regressions, prior to any speculation of further support. Light shading indicates period where differential investment tax break remains in place, but excluded from DD regressions.
Sources: ABS; Authors' calculations.
Consistent with this, the regression analysis suggests that the 2012 policy did not significantly raise investment (Table 7). As such we find no evidence the 2012 polices affected investment.
Intensive margin | Extensive margin | ||||||
---|---|---|---|---|---|---|---|
All firms | Companies | Unincorporated | All firms | Companies | Unincorporated | ||
GFC 2009 | 0.456*** (0.136) |
0.414** (0.168) |
0.870*** (0.238) |
0.207* (0.113) |
0.176 (0.132) |
0.274** (0.139) |
|
Observations | 7,226 | 4,660 | 2,520 | 46,069 | 24,758 | 21,293 | |
Small 2012 | 0.091 (0.125) |
0.031 (0.165) |
0.321 (0.200) |
0.043 (0.087) |
0.076 (0.095) |
−0.031 (0.110) |
|
Observations | 7,253 | 4,704 | 2,521 | 49,474 | 25,920 | 23,534 | |
Small 2015 | 0.140 (0.129) |
0.070 (0.159) |
0.440* (0.230) |
0.138 (0.100) |
0.137 (0.120) |
0.154 (0.105) |
|
Observations | 3,911 | 2,631 | 1,270 | 24,172 | 13,298 | 10,857 | |
Notes: Regressions include controls. ***, ** and * denote statistical significance at the 1, 5 and 10 per cent levels, respectively. Cluster robust standard errors in parentheses. |
There is some evidence that the 2015 policy had an effect on investment, particularly on the intensive margin for unincorporated businesses. However, it is somewhat weaker compared to the GFC policy. Furthermore, the 2015 policy may be confounded by the cuts to the corporate tax rate for small businesses that were also being implemented at the same time. As discussed below in Section 7.1, we do find some evidence these polices affected investment for companies. It is possible this could have led to some spillovers to unincorporated businesses, so the evidence of effects should be taken with some additional caution.[14],[15]
Again, the RDD results are fairly similar to the DD results. There is no evidence that the 2012 policy increased investment, even when focusing only on unincorporated businesses (Figure 6 and Table 8). The 2015 policy on the other hand did lead to some increase in investment, though only for unincorporated businesses (Figure 7 and Table 9). Again, the potential influence of the corporate tax rate cuts should be kept in mind. Moreover, the effect is evident in the March 2016 quarter, once the next set of incentives that applied to firms with up to $10 million in revenue had already been announced, which may confound the results (though the Medium 2016 policy does not seem to have had an effect).
The apparent negative impact of the policy in the June 2015 quarter, before the policy began, may be a sign that firms held off investment in anticipation of the policy. If this were the case, however, we would expect to see a strong impact of the policy in the first two quarters where it was in place (September and December 2015). However, there is no measurable impact of the policy during that period.
These lack of responses, particularly for the 2012 policy, may seem somewhat surprising in the context of the GFC results, given the targeted groups were identical. However, the 2012 changes happened in a context of heightened political uncertainty. The legislation enacting the increase from $1,000 to $5,000 was contingent on the passage of the mineral resource rent tax legislation. The further increase to $6,500 contingent on the passage of legislation related to emissions reductions. Uncertainty around these other policies, particularly the fact that the opposition party committed to unwinding these policies if it won government before the expiration of the tax break, may have led businesses to hold off on investment.
Some evidence suggests the 2015 policy had a small effect for unincorporated businesses. However, this evidence is weaker and macroeconomically less significant, given we find no aggregate economic impact. This could reflect a number of factors: heightened political uncertainty with the Australian Senate blocking government legislation and culminating in the first double dissolution election since 1987; the smaller nature of the policy; the introduction of the policy at the same time as corporate tax cuts that lowered the value of the investment tax break; and looser financial conditions, which may have meant less scope for the policy to influence companies.
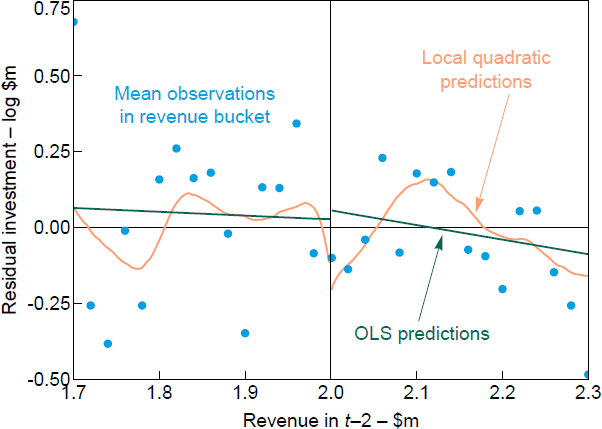
Note: Residual from a regression of log investment on industry fixed effects.
Mar 2012 | Jun 2012 | Sep 2012 | Dec 2012 | Mar 2013 | Jun 2013 | Sep 2013 | Dec 2013 | |
---|---|---|---|---|---|---|---|---|
All firms | ||||||||
Beta | −0.149 | −0.039 | −0.047 | 0.162 | 0.186 | 0.171 | −0.042 | −0.112 |
Robust p-value | (0.53) | (0.78) | (0.85) | (0.25) | (0.26) | (0.14) | (0.93) | (0.61) |
Bandwidth | 372,005 | 421,748 | 379,932 | 345,167 | 380,623 | 406,370 | 316,471 | 411,141 |
Effective obs | 2,765 | 3,177 | 2,713 | 2,556 | 2,639 | 2,952 | 2,402 | 2,990 |
Companies | ||||||||
Beta | −0.288 | 0.091 | 0.040 | 0.187 | 0.151 | 0.527** | 0.148 | −0.173 |
Robust p-value | (0.21) | (0.77) | (0.91) | (0.32) | (0.70) | (0.02) | (0.41) | (0.43) |
Bandwidth | 436,224 | 390,962 | 401,357 | 456,602 | 362,765 | 323,714 | 410,413 | 539,201 |
Effective obs | 1,664 | 1,614 | 1,470 | 1,631 | 1,312 | 1,317 | 1,531 | 1,813 |
Unincorporated | ||||||||
Beta | 0.298 | −0.163 | −0.149 | 0.040 | 0.203 | −0.118 | −0.121 | 0.011 |
Robust p-value | (0.69) | (0.60) | (0.70) | (0.66) | (0.24) | (0.72) | (0.92) | (0.81) |
Bandwidth | 381,694 | 482,329 | 360,767 | 327,458 | 434,046 | 400,103 | 290,010 | 367,851 |
Effective obs | 1,298 | 1,557 | 1,255 | 1,170 | 1,383 | 1,371 | 1,059 | 1,344 |
Notes: Bolded quarters are policy periods. ***, ** and * denote statistical significance at the 1, 5 and 10 per cent levels, respectively. |
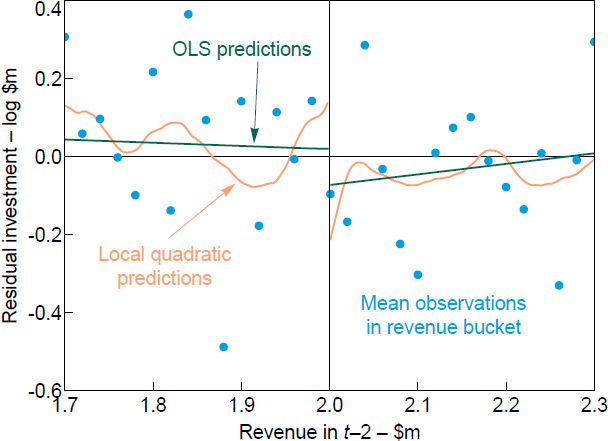
Note: Residual from a regression of log investment on industry fixed effects.
Mar 2015 | Jun 2015 | Sep 2015 | Dec 2015 | Mar 2016 | Jun 2016 | |
---|---|---|---|---|---|---|
All firms | ||||||
Beta | −0.126 | 0.226* | −0.012 | 0.055 | −0.306* | −0.279* |
Robust p-value | (0.47) | (0.08) | (0.90) | (0.61) | (0.07) | (0.07) |
Bandwidth | 402,386 | 344,157 | 376,464 | 407,926 | 326,053 | 368,267 |
Effective observations | 3,234 | 3,161 | 3,129 | 3,412 | 2,915 | 3,333 |
Companies | ||||||
Beta | −0.258 | −0.021 | 0.180 | 0.446** | −0.136 | −0.051 |
Robust p-value | (0.20) | (0.92) | (0.52) | (0.03) | (0.47) | (0.76) |
Bandwidth | 334,682 | 400,725 | 385,850 | 350,061 | 451,190 | 484,585 |
Effective observations | 1,492 | 1,875 | 1,728 | 1,688 | 1,955 | 2,154 |
Unincorporated | ||||||
Beta | 0.011 | 0.484** | −0.235 | −0.356 | −0.518** | −0.567** |
Robust p-value | (0.76) | (0.01) | (0.40) | (0.10) | (0.03) | (0.01) |
Bandwidth | 381,886 | 386,019 | 375,716 | 352,855 | 315,226 | 329,403 |
Effective observations | 1,482 | 1,591 | 1,408 | 1,436 | 1,277 | 1,399 |
Notes: Bolded quarters are policy periods. ***, ** and * denote statistical significance at the 1, 5 and 10 per cent levels, respectively. |
6.3 Structural medium business policies
Focusing on the 2016 and 2019 medium business policies, which cover firms at the $10 million and $50 million thresholds, respectively, there is again little graphical evidence of an effect in the DD approach (See Figures 8 and 9). The pre-trends for the extensive margins are quite volatile suggesting that these results should be interpreted with some caution.[16]
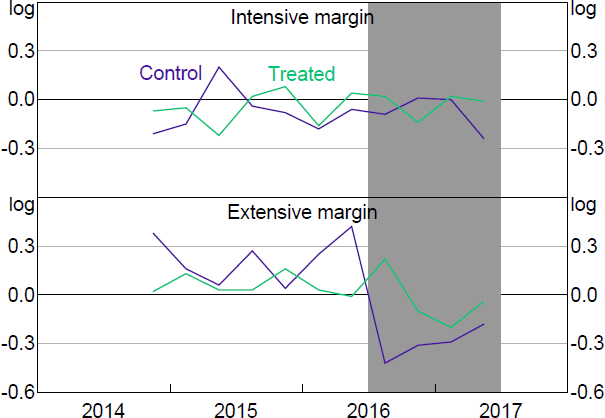
Notes: Controls for employment size, turnover decile, industry division time trends and seasonal size dummies. Shading indicates the treatment period for regressions.
Sources: ABS; Authors' calculations.
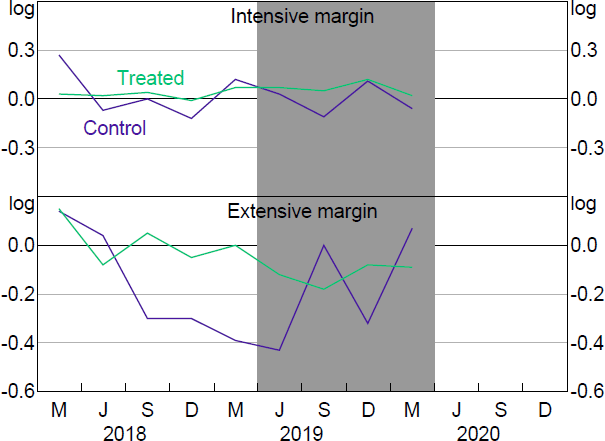
Notes: Controls for employment size, turnover decile, industry division time trends and seasonal size dummies. Shading indicates the treatment period for regressions.
Sources: ABS; Authors' calculations.
Turning to the regression results, there is some evidence of an effect at the extensive margin in the DD approach for all firms, though as noted, we should be cautious in interpreting this result (Table 10). Moreover, as discussed below, in part these results might reflect the effect of coincident corporate tax cuts (Section 7.1). Meanwhile, the 2019 policy does not appear to have increased investment.
Intensive margin | Extensive margin | ||||||
---|---|---|---|---|---|---|---|
All firms | Companies | Unincorporated | All firms | Companies | Unincorporated | ||
Medium 2016 | −0.074 (0.120) |
0.017 (0.139) |
−0.618** (0.260) |
0.295** (0.122) |
0.293** (0.142) |
0.295 (0.178) |
|
Observations | 8,663 | 6,846 | 1,772 | 18,962 | 14,062 | 4,873 | |
Medium 2019 | 0.034 (0.116) |
0.084 (0.125) |
−0.135 (0.253) |
−0.289 (0.203) |
−0.211 (0.200) |
−0.784** (0.336) |
|
Observations | 7,978 | 6,732 | 1,212 | 13,646 | 11,026 | 2,593 | |
Notes: Regressions include controls. ***, ** and * denote statistical significance at the 1, 5 and 10 per cent levels, respectively. Cluster robust standard errors in parentheses. |
We also show the RDD results for the 2016 policy in Table 11. There is no evidence that the policy led to increased investment. However, firms are sparser around the $10 million cut-off, as evidenced by the larger optimal bandwidth and smaller observation count. There may be too few firms to precisely estimate an RDD model for this policy.
Mar 2016 | Jun 2016 | Sep 2016 | Dec 2016 | Mar 2017 | Jun 2017 | |
---|---|---|---|---|---|---|
All businesses | ||||||
Beta | 0.099 | 0.293 | −0.247 | 0.179 | 0.140 | −0.140 |
Robust p-value | (0.78) | (0.14) | (0.36) | (0.49) | (0.47) | (0.49) |
Bandwidth | 2,156,505 | 2,296,991 | 1,702,082 | 2,275,956 | 1,970,100 | 1,451,920 |
Effective observations | 1,154 | 1,224 | 1,039 | 1,218 | 1,140 | 957 |
Companies | ||||||
Beta | 0.144 | 0.017 | −0.272 | 0.330 | 0.401 | 0.058 |
Robust p-value | (0.69) | (0.98) | (0.33) | (0.27) | (0.16) | (0.99) |
Bandwidth | 2,663,093 | 2,315,771 | 1,499,775 | 2,357,502 | 1,638,978 | 1,619,548 |
Effective observations | 874 | 852 | 670 | 874 | 712 | 695 |
Unincorporated | ||||||
Beta | −0.026 | 0.921** | −0.342 | −0.186 | −0.302 | −0.561 |
Robust p-value | (0.79) | (0.03) | (0.60) | (0.75) | (0.62) | (0.19) |
Bandwidth | 1,497,854 | 1,765,229 | 1,969,430 | 1,850,328 | 2,080,014 | 1,673,069 |
Effective observations | 293 | 331 | 326 | 333 | 338 | 334 |
Notes: Bolded quarters are policy periods. ***, ** and * denote statistical significance at the 1, 5 and 10 per cent levels, respectively. |
7. Robustness
In this section we discuss a number of threats to the identification of our models and conduct several robustness checks to assess the sensitivity of our findings and to aid in interpretation. These include the potential for firms to adjust their behaviour to utilise the policy, and other policies that may have affected behaviour.
7.1 The impact of corporate tax cuts
Unfortunately, the 2015 and 2016 results may be confounded by corporate tax cuts that were implemented for the policy groups contemporaneously. These were introduced at the same time as the ITB, and had the exact same size cut-off for eligibility (see Tables 1 and 2).
In order to consider whether these policies had an effect, we exploit the fact that the ITB only applied to machinery & equipment, while the tax cuts lowered the cost of capital for any investment. We re-estimate the DD models, but for buildings & structures investment, as reported in the CAPEX data. While there is no response of this ineligible investment to the GFC policy, we do see a significant increase for the 2015 policy at the intensive margin (Table C1). As discussed above, this could potentially bias us towards finding a response to ITB, though in practice we find no evidence of a response from companies in either case. It does suggest that the corporate tax cuts themselves may have had an impact on increased investment. There is no evidence of an effect from the 2016 tax rate change.
7.2 Other policies
We know about the corporate tax rates changes potentially affecting the 2015 and 2016 ITB policies but it may be that there are other policies that create differential incentives to invest at the threshold.
One possibility is that such policies pre-existed the ITB. This is unlikely to affect the DD results, as it will be captured by the pre-treatment difference in outcomes. But it could affect the RDD results. To consider this possibility we run the RDD for a few quarters prior to the introduction of the policy. These are included in the RDD results tables. There is no estimated systematic difference in investment at the threshold before the policies were in place, suggesting that it is not other pre-existing policies driving the results.
Another way to account for potentially confounding policies or factors that affect firms at the threshold is to look at other firm-level metrics that could be affected by those policies, but which should be less affected by the investment tax incentives. Table 12 examines firms' wages and revenue, focusing on quarters where there was evidence of a significant investment response. We find no evidence of a discontinuity in these other observables, providing further evidence that the effects we are finding reflect the investment policies.
Sep 2009 | Dec 2015 | Mar 2016 | Jun 2016 | |
---|---|---|---|---|
Wages | ||||
Beta | 0.039 | 0.030 | 0.070 | 0.066 |
Robust p-value | (0.38) | (0.52) | (0.12) | (0.11) |
Bandwidth | 337,338 | 384,609 | 373,948 | 372,523 |
Effective observations | 5,131 | 7,917 | 7,781 | 7,771 |
Revenue | ||||
Beta | −0.017 | −0.030 | 0.010 | −0.026 |
Robust p-value | (0.67) | (0.12) | (0.84) | (0.32) |
Bandwidth | 350,983 | 288,230 | 404,467 | 307,524 |
Effective observations | 5,599 | 7,899 | 9,517 | 8,224 |
Note: ***, ** and * denote statistical significance at the 1, 5 and 10 per cent levels, respectively. |
7.3 Validity tests – selection into treatment
One of the requirements for valid RDD is that businesses cannot manipulate the forcing variable, revenue, to change their treatment status. If businesses can select into treatment, the assumption that businesses just above and below the cut-off are identical would be violated. Businesses with a higher ex ante propensity to invest would have a greater incentive to select into treatment.
In our case, manipulation of the forcing variable – revenue measured over a year before the announcement of the differential tax break – is improbable. Consistent with this, there is no graphical evidence of bunching of businesses just below the threshold (Figure 10). These graphical results are confirmed by the formal test proposed by Cattaneo, Jansson and Ma (2020).
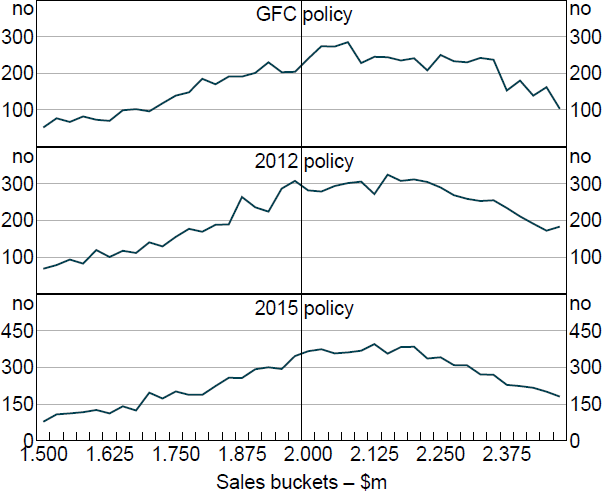
Notes: Sales buckets are $25,000 wide. Sales in year t – 2.
As discussed in Rodgers and Hambur (2018), one additional concern that is fairly unique to our RDD set-up due to the policy rules is that businesses might be manipulating their way out of the estimation sample. In particular, businesses with revenue above the cut-off in t – 2 and who forecast revenue just above the threshold in t – 1 or t, might have the incentive to manipulate their revenue in those years to qualify for the tax breaks. The firms would be manipulating their way our of the ‘control’ group (but not into the treated group). This could create a situation where our control group is comprised of firms with a lower propensity to invest than a typical firm, as those firms with a higher propensity to invest have manipulated out of the sample.
We test this possibility in two ways. One is to replace current investment in the regression with two-year lagged investment. This test of a pre-determined covariate should provide some sense of whether there are inherent differences between the treated and control groups.
Table 13 shows these results, focusing on the periods where the investment response was statistically significant. We find no evidence that there was a difference in the earlier investment propensity of firms either side of the threshold.
Sep 2009 | Dec 2015 | Mar 2016 | Jun 2016 | |
---|---|---|---|---|
Beta | 0.003 | −0.065 | −0.007 | 0.095 |
Robust p-value | (0.93) | (0.69) | (0.96) | (0.45) |
Bandwidth | 464,597 | 377,614 | 403,782 | 405,104 |
Effective observations | 2,763 | 3,134 | 3,181 | 3,506 |
Note: ***, ** and * denote statistical significance at the 1, 5 and 10 per cent levels, respectively. |
Still, we may be concerned that even if the earlier propensity to invest was the same, it may not be the case during the period of interest. As a further test, we rely on the assumption that businesses are only likely to be able to manipulate their revenue to a certain extent. Businesses with revenue just above the threshold may be able to manipulate their revenue down, but businesses with revenue well above the turnover limit could not.
As shown in Rodgers and Hambur (2018, Figure 6), there is some evidence that firms manipulated their sales in year t – 1 to qualify for the GFC policy. Specifically, there is a small spike in the number of firms with turnover just below $2 million in 2008/09, followed by a dip just after. It appears that most of this came from firms with ‘potential’ turnover between $2 million and $2.1 million.
To account for this we follow Rodgers and Hambur (2018) and re-estimate the model, only keeping firms with revenue in t – 1 or t that was more than 5 per cent above the threshold (equivalent to $100,000 for the $2 million threshold). The logic here is that for this subset of firms, it would have been harder for them to manipulate turnover to be below the threshold. As such, amongst the remaining firms we should be less worried that we are only left with those that didn't have the incentive to manipulate into the policy (and out of our sample) using turnover in t or t – 1, as all remaining firms would have had moderate difficulty in doing so.
Table 14 shows the results, again focusing on the statistically significant periods. The results are quite similar, suggesting that manipulation is not driving the findings.
Sep 2008 | Dec 2015 | Mar 2016 | Jun 2016 | |
---|---|---|---|---|
All firms | ||||
Beta | −0.364* | −0.073 | −0.500** | −0.197 |
Robust p-value | (0.05) | (0.79) | (0.02) | (0.35) |
Bandwidth | 306,648 | 426,061 | 298,216 | 522,424 |
Effective observations | 1,584 | 2,763 | 1,969 | 3,014 |
Unincorporated | ||||
Beta | −0.528* | −0.542** | −0.728** | −0.607** |
Robust p-value | (0.06) | (0.04) | (0.02) | (0.03) |
Bandwidth | 315,977 | 335,454 | 311,429 | 386,911 |
Effective observations | 705 | 1,198 | 1,010 | 1,208 |
Note: ***, ** and * denote statistical significance at the 1, 5 and 10 per cent levels, respectively. |
7.4 Repeated treatment
As Australia has implemented a number of different ITB policies over recent years, one concern could be interaction between the various policies and overlap of the time periods covered by the policies. For example, firms that took up the GFC policy may have had less incentive to invest using the 2012 policy if they had already made all the investment they desired.
We do see some evidence of overlap. For example, around half of the treated group in our GFC regressions were in our treated group for the 2012 policy. However, both our results and Rodgers and Hambur (2018) provide very limited evidence of bring forward over the one to two years following the GFC policy. We think the likelihood that this is driving our results for the 2012 policy is very low.
8. Conclusions
Various tax incentives have been provided to businesses in Australia over the past 15 years, both as a stimulus tool and as a structural tool to raise aggregate investment. Such incentives are inherently costly in fiscal terms, and so it is important to understand whether they are actually effective in stimulating additional investment.
We evaluate seven policies over a twelve-year period using difference in differences and regression discontinuity designs to identify the causal impact of investment tax breaks on firm investment. Our time period covers ‘normal’ economic times, the global financial crisis and the COVID-19 pandemic. This approach of examining a range of policies, at different time periods and in one country allows us to draw conclusions about heterogeneous effects of investment tax breaks free from the problems of poor comparability often found in cross-country studies.
ITBs seem to be somewhat less effective in Australia, compared to many other countries. This appears related to the existence of dividend imputation, which lessens the value of the ITB to company shareholders and means that the policies mainly affect small unincorporated businesses. This is an important insight about the relationship between ITBs and other elements of the design of the overall tax system. Our finding is consistent with the old view of corporate finance, where firms fund themselves externally.
Overall, our results suggest the effectiveness of the policies studied is mixed at best, and probably highly dependent on the nature of the policy and macroeconomic conditions. In Australia, ITB policies as economic stimulus may be most effective during downturns which are driven by a shock to credit supply, rather than a sharp decrease in demand. ITBs appear to loosen cash flow constraints for businesses in periods of credit dislocation. In normal times they seem to have little effect. Policies targeted at smaller firms appear somewhat more effective: unincorporated businesses in particular are far more responsive. Policy uncertainty, reflected in frequent policy changes and uncertainty about the continuation of a policy, may undermine effectiveness.
Finally, while not the main focus of our paper, we do find some evidence that corporate tax rate cuts led to an increase in investment, consistent with theory and previous empirical evidence from cross-country and other studies.
Appendix A: Policy Episodes
A.1 The GFC investment tax credit
The Australian Government provided a temporary bonus income tax deduction for new investment in tangible depreciating assets undertaken between 13 December 2008 and 31 December 2009.
For small business entities (where turnover was less that $2 million), the tax break was 50 per cent of their investment in an eligible asset undertaken on or before 31 December 2009. For all other taxpayers, there was a 30 per cent deduction for all investments committed to before 30 June 2009 and a 10 per cent deduction for those committed to during the second half of 2009. The tax break could be claimed in the income year that the asset was first used or installed ready for use. The GFC tax break was in addition to any depreciation allowances for the asset in the year of investment and future depreciation deductions. New investment in relation to an asset needed to exceed a certain threshold before qualifying for the tax break. The new investment threshold was $1,000 for small business entities and $10,000 for all other taxpayers.
The investment tax break was announced in the Treasurer's Media Releases No. 141 of 12 December 2008 and No. 013 of 3 February 2009 and as part of the 2009/10 Budget of 12 May 2009. Amendments were passed in Tax Laws Amendment (Small Business and General Business Tax Break) Act 2009, which received Royal Assent on 22 May 2009.
For a pre-policy period over which we assess common trends we take the period from 1 July 2007. This is when the definition of small business was changed to $2 million in turnover. The end of the pre-policy period was 30 June 2009.
A.2 The small business incentive 2012
Small business taxpayers received an increase in the instant asset write-off cost threshold from $1,000 to $6,500 for 2012/13 and later income years. Simplification and consolidation of the small business depreciation pools were also implemented at the same time.
The change in asset threshold from $1,000 to $5,000 was announced on 2 May 2010. On 10 July 2011, the Australian Government announced the small business instant write-off threshold would be further increased to $6,500. The amendments were passed in the Tax Laws Amendment (Stronger, Fairer, Simpler and Other Measures) Act 2012, which received Royal Assent on 29 March 2012.
With a change in government, and repeal of the Mineral Resource Rent Tax (MRRT), the threshold was moved back to $1,000. The repeal of the MRRT was announced on 24 October 2013. The repeal of the higher threshold and related spending measures was announced later on 18 July 2014. The change in threshold was effective from 30 September 2014, with the passage of the Minerals Resource Rent Tax Repeal and Other Measures Bill 2014, which received Royal Assent on 5 September 2014.
We take the date of announcement of the repeal of the MRRT as the end date of the policy since the original threshold increase was funded by the MRRT. Businesses would have anticipated the repeal of other spending measures when the repeal of the MRRT was announced.
We define the policy period from 1 July 2012 to 31 December 2013. To look at pre-trends we take the period 1 January 2010 to 30 June 2012, which is the period after the end of the GFC investment incentive.
A.3 The small business incentive 2015
Small business entities (i.e. businesses with a turnover up to $2 million) were given an increased immediate deduction for a depreciating asset costing less than $20,000 from 12 May 2015. The temporary increase applied from 12 May 2015 until 30 June 2017. However, we only consider the policy through to 31 December 2015 to avoid any potential influence from the introduction of the lower corporate tax rate for firms with turnover up to $10 million (which include our control group) in May 2016.
The small business corporate tax rate was cut to 28.5 per cent in 2015 by the Tax Laws Amendment (Small Business Measures No. 1) Bill 2015, which received Royal Assent on 22 June 2015.
A.4 The expanded business incentive 2016
The small business threshold was increased from $2 million to $10 million and the corporate tax rate was cut to 27.5 per cent for businesses with a turnover less than $10 million from 2016/17 onwards. This meant that business with a turnover up to $10 million could access instant write-off provisions for assets costing up to $20,000. With the extension of that provision by 12 months, to 30 June 2018 (Income Tax (Transitional Provisions) Act 1997).
The tax cuts and change in definition of small businesses were announced on 3 May 2016 as part of the 2016/17 Budget and implemented in Treasury Laws Amendment (Enterprise Tax Plan) Act 2017, which received Royal Assent on 19 May 2017.
For identification purposes we take 1 July 2017 as the end date of this policy. After this date, tax cuts (lowering the corporate rate to 27.5 per cent) were implemented for businesses with a turnover of less than $25 million as per the Treasury Laws Amendment (Enterprise Tax Plan) Act 2017.
A.5 The medium-sized business incentive 2019
The instant asset write-off cap increased the maximum amount of allowable expenditure for each asset of $30,000 between 2 April 2019 to 30 June 2020 (although this was overtaken by new provisions that commenced on 11 March 2020 and included businesses with a turnover of up to $50 million).
The corporate tax rate was cut to 27.5 per cent for businesses with a turnover of less than $50 million from 1 July 2018 onwards, in accordance with legislation passed in 2017 (Treasury Laws Amendment (Enterprise Tax Plan) Act 2017).
We define the policy period as 2 April 2019 to 11 March 2020. We define the treatment group as businesses with a turnover of between $10 million and $50 million for 2018/19. We use businesses with a turnover of more than $50 million as the control group.
A.6 The large businesses incentive
The asset cost cap was increased from $30,000 to $150,000 and the turnover threshold was increased from $50 million to $500 million from 12 March 2020 to 30 June 2020. The proposal was announced in March and enacted in Coronavirus Economic Response Package Omnibus Act 2020. This policy was extended by six months to end on 31 December 2020 (announced in June 2020 and passed in Treasury Laws Amendment (2020 Measures No. 3) Act 2020). However, this was overtaken by the expanded COVID-19 temporary full expensing package, which started on 6 October 2020.
We define the policy period as 1 April 2020 to 30 September 2020. We define the treatment group as businesses with a turnover of between $10 million and $50 million for 2018/19. We use businesses with a turnover of more than $50 million as the control group.
A.7 Full expensing measures for very large businesses
Lastly, the instant asset write-off was expanded into temporary full expensing (TFE). Under TFE, businesses with a turnover of less than $5 billion could deduct the full cost of eligible depreciating assets. There was no cap on the value of the asset price. It applied to assets first used or installed between 6 October 2020 and 30 June 2022. The policy was announced on 6 October 2020 and passed in Treasury Laws Amendment (A Tax Plan for the COVID-19 Economic Recovery) Act 2020.
We define a policy period of 1 October 2020 to 30 June 2021, the latest observation in our data (the policy expired on 30 June 2023). We define a treatment group of businesses with a turnover between $500 million and $5 billion and use businesses with a turnover between $5 and $6 billion as the control group.
Appendix B: Summary Statistics
Treated | Control | ||||||
---|---|---|---|---|---|---|---|
Mean | Std deviation | Obs | Mean | Std deviation | Obs | ||
Investment(a) ($'000) | 156.68 | 1,783.51 | 16,242 | 214.42 | 3,305.30 | 5,052 | |
Extensive margin | –1.40 | 0.87 | 75,542 | 0.27 | 1.75 | 10,123 | |
Group turnover ($m) | 0.42 | 0.84 | 86,202 | 3.28 | 0.86 | 13,780 | |
Employment | 14.18 | 137.90 | 54,595 | 27.14 | 94.24 | 11,821 | |
Notes: Group turnover is the sum of the turnovers of all entities in the enterprise group to
which a firm belongs. Where a firm does not belong to an enterprise group, group turnover is
equivalent to firm turnover. Firms with group turnover under $2 million are included in the
treated group. Firms with group turnover $2 million to $5 million are assigned to the
control. |
Treated | Control | ||||||
---|---|---|---|---|---|---|---|
Mean | Std deviation | Obs | Mean | Std deviation | Obs | ||
Investment(a) ($'000) | 224.42 | 2,817.32 | 6,223 | 268.32 | 1,323.15 | 3,678 | |
Extensive margin | 0.06 | 1.48 | 13,017 | 1.12 | 1.87 | 5,702 | |
Group turnover ($m) | 5.10 | 2.31 | 14,959 | 14.28 | 2.82 | 6,529 | |
Employment | 54.28 | 275.22 | 12,802 | 82.32 | 139.00 | 5,745 | |
Notes: Group turnover is the sum of the turnovers of all entities in the enterprise group to
which a firm belongs. Where a firm does not belong to an enterprise group, group turnover is
equivalent to firm turnover. Firms with group turnover $2 million to $10 million are included in
the treated group. Firms with group turnover $10 million to $20 million are assigned to the
control. |
Treated | Control | ||||||
---|---|---|---|---|---|---|---|
Mean | Std deviation | Obs | Mean | Std deviation | Obs | ||
Investment(a) ($'000) | 393.21 | 1,956.87 | 6,953 | 531.55 | 1,606.68 | 890 | |
Extensive margin | 0.84 | 1.60 | 11,151 | 2.29 | 1.94 | 1,118 | |
Group turnover ($m) | 25.49 | 11.59 | 11,716 | 54.63 | 2.88 | 1,357 | |
Employment | 119.44 | 225.32 | 10,531 | 208.31 | 230.34 | 1,224 | |
Notes: Group turnover is the sum of the turnovers of all entities in the enterprise group to
which a firm belongs. Where a firm does not belong to an enterprise group, group turnover is
equivalent to firm turnover. Firms with group turnover $10 million to $50 million are included
in the treated group. Firms with group turnover $50 million to $100 million are assigned to the
control. |
Treated | Control | ||||||
---|---|---|---|---|---|---|---|
Mean | Std deviation | Obs | Mean | Std deviation | Obs | ||
Investment(a) ($'000) | 1,355.62 | 5,467.85 | 16,619 | 3,413.06 | 11,531.34 | 1,082 | |
Extensive margin | 1.64 | 1.54 | 21,938 | 3.09 | 1.60 | 1,208 | |
Group turnover ($m) | 196.90 | 120.30 | 22,231 | 549.30 | 28.83 | 1,337 | |
Employment | 412.37 | 470.99 | 20,751 | 771.23 | 920.41 | 1,305 | |
Notes: Group turnover is the sum of the turnovers of all entities in the enterprise group to
which a firm belongs. Where a firm does not belong to an enterprise group, group turnover is
equivalent to firm turnover. Firms with group turnover $50 million to $500 million are included
in the treated group. Firms with group turnover $500 million to $600 million are assigned to the
control. |
Treated | Control | ||||||
---|---|---|---|---|---|---|---|
Mean | Std deviation | Obs | Mean | Std deviation | Obs | ||
Investment(a) ($'000) | 156.68 | 1,783.51 | 2,079 | 214.42 | 3,305.30 | 114 | |
Extensive margin | –1.40 | 0.87 | 2,537 | 0.27 | 1.75 | 136 | |
Group turnover ($m) | 0.42 | 0.84 | 2,582 | 3.28 | 0.86 | 154 | |
Average asset life | 15.48 | 2.37 | 2,582 | 15.94 | 2.26 | 154 | |
Employment | 14.18 | 137.90 | 2,553 | 27.14 | 94.24 | 151 | |
Notes: Group turnover is the sum of the turnovers of all entities in the enterprise group to
which a firm belongs. Where a firm does not belong to an enterprise group, group turnover is
equivalent to firm turnover. Firms with group turnover $500 million to $5 billion are included
in the treated group. Firms with group turnover $5 billion to $6 billion are assigned to the
control. |
Appendix C: Testing for the Effect of Corporate Tax Changes
To explore the possible effect of the corporate tax cuts, we re-estimate the DD models using buildings & structures investment from the CAPEX data described above. This asset class is ineligible for the investment incentives that we study in this paper.
Looking at the results for the GFC policy period, we can see there is no significant increase in investment in buildings & structures either on the intensive or extensive margins. There appears to be no complementarity between investment in these asset types (i.e. increases in eligible machinery & equipment have no effect on investment in buildings & structures). This suggests that looking at buildings & structures can be a useful placebo test for other ITB policies.
In contrast, for the 2015, and to a lesser extent 2016, policies there is evidence of an increase in buildings & structures investment, suggesting that firms reacted to the corporate tax cuts.[17] As firms appear to be responding to the corporate tax cuts, we should be cautious about interpreting our findings regarding the effects of the 2015 and 2016 ITB.
Intensive margin | Extensive margin | ||||||
---|---|---|---|---|---|---|---|
All firms | Companies | Unincorporated | All firms | Companies | Unincorporated | ||
GFC 2009 | −0.017 (0.440) |
−0.074 (0.526) |
−0.242 (0.173) |
−0.182 (0.172) |
−0.449 (0.285) |
||
Observations | 1,250 | 734 | 25,880 | 13,330 | 12,518 | ||
Small 2015 | 0.665** (0.312) |
0.829* (0.448) |
0.010 (0.226) |
−0.157 (0.269) |
0.070 (0.333) |
||
Observations | 1,317 | 761 | 16,808 | 9,040 | 4,008 | ||
Medium 2016 | 0.171 (0.323) |
0.294 (0.351) |
0.219* (0.125) |
0.156 (0.134) |
0.277 (0.181) |
||
Observations | 1,897 | 1,493 | 10,612 | 7,651 | 2,908 | ||
Notes: Results exclude mining. Regressions do not include controls due to small sample size. ***, ** and * denote statistical significance at the 1, 5 and 10 per cent levels, respectively. Cluster robust standard errors in parentheses. |
Appendix D: Additional RDD Figures
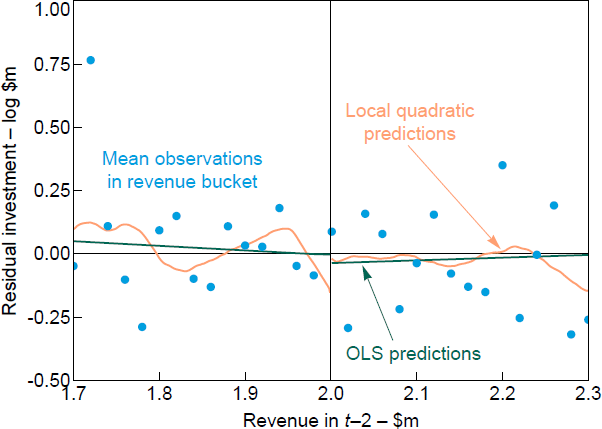
Note: Residual from a regression of log investment on industry fixed effects.
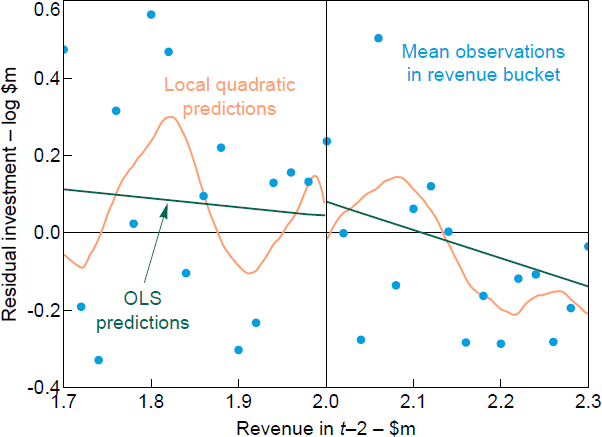
Note: Residual from a regression of log investment on industry fixed effects.
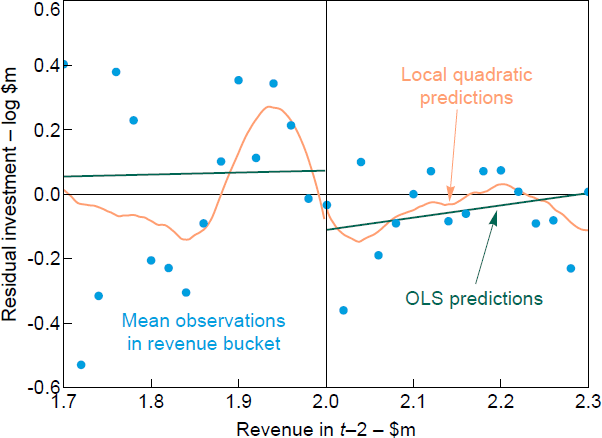
Note: Residual from a regression of log investment on industry fixed effects.
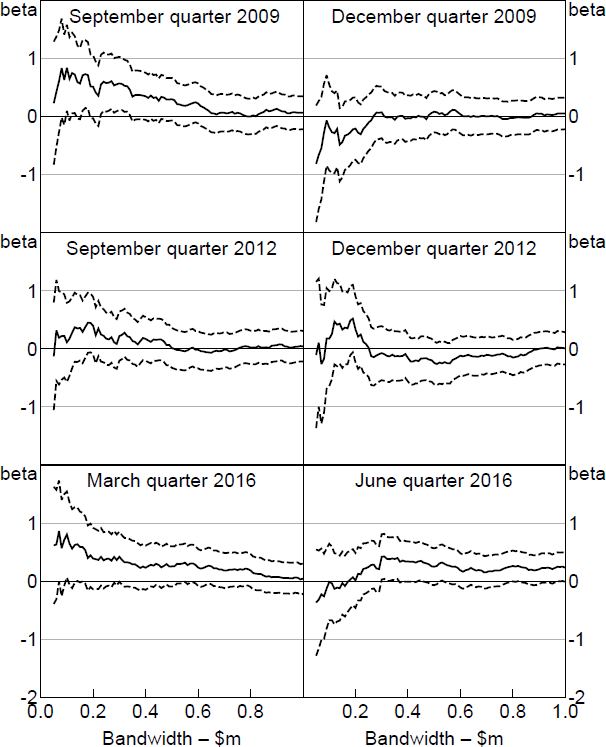
Notes: No covariates, estimated with quadratic. Dashed lines are 95 per cent confidence intervals.
References
Abel AB (1982), ‘Dynamic Effects of Permanent and Temporary Tax Policies in a q Model of Investment’, Journal of Monetary Economics, 9(3), pp 353–373.
Australian Taxation Office (undated), ‘Work Out if You're a Small Business for the Income Year’, Australian Taxation Office [website], accessed on 22 December 2017. Available at <https://webarchive.nla.gov.au/awa/20171113182714/https:/www.ato.gov.au/Business/Small-business-entity-concessions/Eligibility/Work-out-if-you-re-a-small-business-for-the-income-year/>.
Calonico S, MD Cattaneo and R Titiunik (2014), ‘Robust Nonparametric Confidence Intervals for Regression-discontinuity Designs’, Econometrica, 82(6), pp 2295–2326.
Cattaneo MD, M Jansson and X Ma (2020), ‘Simple Local Polynomial Density Estimators’, Journal of the American Statistical Association, 115(531), pp 1449–1455.
Cummins JG, KA Hassett and RG Hubbard (1994), ‘A Reconsideration of Investment Behavior Using Tax Reforms as Natural Experiments’, Brookings Papers on Economic Activity, 2, pp 1–59, 70–74.
Hall RE and DW Jorgenson (1967), ‘Tax Policy and Investment Behavior’, The American Economic Review, 57(3), pp 391–414.
House CL and MD Shapiro (2008), ‘Temporary Investment Tax Incentives: Theory with Evidence from Bonus Depreciation’, The American Economic Review, 98(3), pp 737–768.
Imbens GW and T Lemieux (2008), ‘Regression Discontinuity Designs: A Guide to Practice’, Journal of Econometrics, 142(2), pp 615–635.
Kydland FE and EC Prescott (1982), ‘Time to Build and Aggregate Fluctuations’, Econometrica, 50(6), pp 1345–1370.
Lee DS and T Lemieux (2010), ‘Regression Discontinuity Designs in Economics’, Journal of Economic Literature, 48(2), pp 281–355.
Liu Y and J Mao (2019), ‘How Do Tax Incentives Affect Investment and Productivity? Firm-level Evidence from China’, American Economic Journal: Economic Policy, 11(3), pp 261–291.
Maffini G, J Xing and MP Devereux (2019), ‘The Impact of Investment Incentives: Evidence from UK Corporation Tax Returns’, American Economic Journal: Economic Policy, 11(3), pp 361–389.
Officer RR (1994), ‘The Cost of Capital of a Company under an Imputation Tax System’, Accounting & Finance, 34(1), pp 1–17.
Parliament of the Commonwealth of Australia, The (2019), ‘Supplementary Explanatory Memorandum’, Treasury Laws Amendment (Increasing the Instant Write-off for Small Business Entities) Bill 2019.
Rodgers D and J Hambur (2018), ‘The GFC Investment Tax Break’, RBA Research Discussion Paper No 2018-07.
Sobeck K, R Breunig and A Evans (2022), Corporate Income Taxation in Australia: Theory, Current Practice and Future Policy Directions, Tax and Transfer Policy Institute Report No 01-2022, Canberra.
Swan PL (2019), ‘Investment, the Corporate Tax Rate, and the Pricing of Franking Credits’, Economic Record, 95(311), pp 480–496.
Swan W (Treasurer) (2008), ‘Investment Allowance to Boost Business Investment’, Media Release No 141, 12 December.
Swan W (Treasurer) (2009a), ‘Small Business and General Business Tax Break’, Media Release No 013, Joint Media Release with the Prime Minister and Minster for Small Business, 3 February.
Swan W (Treasurer) (2009b), ‘Small Business Tax Break Boost’, Press Release No 061, Joint Press Release with the Hon Dr Craig Emerson MP Minister for Small Business, Independent Contractors and the Service Economy, 12 May.
Yagan D (2015), ‘Capital Tax Reform and the Real Economy: The Effects of the 2003 Dividend Tax Cut’, The American Economic Review, 105(12), pp 3531–3563.
Zhang L, Y Chen and Z He (2018), ‘The Effect of Investment Tax Incentives: Evidence from China's Value-added Tax Reform’, International Tax and Public Finance, 25(4), pp 913–945.
Zwick E and J Mahon (2017), ‘Tax Policy and Heterogeneous Investment Behavior’, The American Economic Review, 107(1), pp 217–248.
Copyright and Disclaimer Notice
BLADE Disclaimer
The following Disclaimer Notice refers to data and graphs sourced from the Australian Bureau of Statistics' BLADE (Business Longitudinal Analysis Data Environment) database.
The results of these studies are based, in part, on data supplied to the ABS under the Taxation Administration Act 1953, A New Tax System (Australian Business Number) Act 1999, Australian Border Force Act 2015, Social Security (Administration) Act 1999, A New Tax System (Family Assistance) (Administration) Act 1999, Paid Parental Leave Act 2010 and/or the Student Assistance Act 1973. Such data may only be used for the purpose of administering the Census and Statistics Act 1905 or performance of functions of the ABS as set out in section 6 of the Australian Bureau of Statistics Act 1975. No individual information collected under the Census and Statistics Act 1905 is provided back to custodians for administrative or regulatory purposes. Any discussion of data limitations or weaknesses is in the context of using the data for statistical purposes and is not related to the ability of the data to support the Australian Taxation Office, Australian Business Register, Department of Social Services and/or Department of Home Affairs' core operational requirements.
Legislative requirements to ensure privacy and secrecy of these data have been followed. For access to MADIP and/or BLADE data under Section 16A of the ABS Act 1975 or enabled by section 15 of the Census and Statistics (Information Release and Access) Determination 2018, source data are de-identified and so data about specific individuals has not been viewed in conducting this analysis. In accordance with the Census and Statistics Act 1905, results have been treated where necessary to ensure that they are not likely to enable identification of a particular person or organisation.
Acknowledgements
Nu Nu Win would like to gratefully acknowledge the support of the Sir Roland Wilson Foundation PhD Scholarship Program. Thanks to seminar participants at the RBA, Australian Treasury, Department of Prime Minister and Cabinet, and the 2023 ANU Public Finance Conference. The views expressed in this paper are those of the authors and should not be attributed to the Reserve Bank of Australia, Australian Treasury or the Australian Government. Any errors are the sole responsibility of the authors.
Footnotes
Australian National University and Australian Treasury [*]
Economic Research Department, Reserve Bank of Australia [**]
Crawford School of Public Policy, Australian National University [***]
For example, the United States, the Netherlands, and South Korea all used investment tax incentives during the global financial crisis (GFC). More recently, the 2017 US tax reform incorporates a significant investment tax incentive in the form of full expensing. [1]
See Appendix A for more details of these policies. [2]
Given tax incentives work through this user cost channel, these policies have often been used to assess the effect of changes in the UC on investment more generally. They are seen as providing more plausibly exogenous variation than changes in financing costs (Cummins, Hassett and Hubbard 1994) and are less prone to measurement error. [3]
For a more detailed discussion see Sobeck, Breunig and Evans (2022). [4]
The broader literature on the value of is mixed. Many large Australian companies raise equity from international investors, who derive no benefit from dividend imputation. As such, is unlikely to be one for these businesses. Applied evidence based on samples of listed companies finds a range of values for , see Swan (2019) and Sobeck et al (2022). At the same time, studies overseas have found evidence in support of the new view (i.e. is 0), with Yagan (2015) finding evidence that cuts to dividend taxes in the United States in 2003 had little effect on investment. [5]
From 2017/18, small firms below $10 million in turnover no longer needed to report on their capital expenditure in the BAS. However, all policies from this point on were aimed at firms above this threshold, so this does not affect our analysis. [6]
More formally, it is defined as ln where n indicates industry, s size and t time [7]
Industry is measured at the division level and takes 19 possible values. The inclusion of these industry dummies will also control for differing asset lives across industries, which determine how beneficial the various policies will be for different industries. [8]
We focus on a static DD model for ease of exposition. Using dynamic DD models that allow for differing effects over the treatment periods leads to similar conclusions. [9]
For discussions on the theory and practice of RDD, see Imbens and Lemieux (2008) and Lee and Lemieux (2010). [10]
We implement this using the rdrobust package in Stata. [11]
Due to small sample sizes at higher turnover ranges, we do not include results by firm type for the COVID policies. [12]
For additional RDD figures see Appendix D. [13]
To the extent that some unincorporated businesses, such as trusts, are pass-through companies for corporate entities, there may also be an effect on investment for unincorporated businesses. We attempt to account for this using ABS information on business links. However, we cannot rule out the possibility that the corporate tax cuts could also affect investment of unincorporated businesses. [14]
Note that we end our DD regression in December 2015. This is because the tax cuts announced in May 2016 for firms with turnover up to $10 million may have affected the behaviour of our control group. That said, the results are broadly similar if we include the March and June quarters of 2016 in the regression. [15]
As noted above, the share of firms investing is much higher once we move to medium and larger firms. As such the extensive margin becomes a less relevant metric for these firms. [16]
Note that intensive margin unincorporated results are not shown due to the very small sample of unincorporated small firms doing building investment. [17]