RDP 2025-01: Are Investment Tax Breaks Effective? Australian Evidence 7. Robustness
February 2025
- Download the Paper 1.89MB
In this section we discuss a number of threats to the identification of our models and conduct several robustness checks to assess the sensitivity of our findings and to aid in interpretation. These include the potential for firms to adjust their behaviour to utilise the policy, and other policies that may have affected behaviour.
7.1 The impact of corporate tax cuts
Unfortunately, the 2015 and 2016 results may be confounded by corporate tax cuts that were implemented for the policy groups contemporaneously. These were introduced at the same time as the ITB, and had the exact same size cut-off for eligibility (see Tables 1 and 2).
In order to consider whether these policies had an effect, we exploit the fact that the ITB only applied to machinery & equipment, while the tax cuts lowered the cost of capital for any investment. We re-estimate the DD models, but for buildings & structures investment, as reported in the CAPEX data. While there is no response of this ineligible investment to the GFC policy, we do see a significant increase for the 2015 policy at the intensive margin (Table C1). As discussed above, this could potentially bias us towards finding a response to ITB, though in practice we find no evidence of a response from companies in either case. It does suggest that the corporate tax cuts themselves may have had an impact on increased investment. There is no evidence of an effect from the 2016 tax rate change.
7.2 Other policies
We know about the corporate tax rates changes potentially affecting the 2015 and 2016 ITB policies but it may be that there are other policies that create differential incentives to invest at the threshold.
One possibility is that such policies pre-existed the ITB. This is unlikely to affect the DD results, as it will be captured by the pre-treatment difference in outcomes. But it could affect the RDD results. To consider this possibility we run the RDD for a few quarters prior to the introduction of the policy. These are included in the RDD results tables. There is no estimated systematic difference in investment at the threshold before the policies were in place, suggesting that it is not other pre-existing policies driving the results.
Another way to account for potentially confounding policies or factors that affect firms at the threshold is to look at other firm-level metrics that could be affected by those policies, but which should be less affected by the investment tax incentives. Table 12 examines firms' wages and revenue, focusing on quarters where there was evidence of a significant investment response. We find no evidence of a discontinuity in these other observables, providing further evidence that the effects we are finding reflect the investment policies.
Sep 2009 | Dec 2015 | Mar 2016 | Jun 2016 | |
---|---|---|---|---|
Wages | ||||
Beta | 0.039 | 0.030 | 0.070 | 0.066 |
Robust p-value | (0.38) | (0.52) | (0.12) | (0.11) |
Bandwidth | 337,338 | 384,609 | 373,948 | 372,523 |
Effective observations | 5,131 | 7,917 | 7,781 | 7,771 |
Revenue | ||||
Beta | −0.017 | −0.030 | 0.010 | −0.026 |
Robust p-value | (0.67) | (0.12) | (0.84) | (0.32) |
Bandwidth | 350,983 | 288,230 | 404,467 | 307,524 |
Effective observations | 5,599 | 7,899 | 9,517 | 8,224 |
Note: ***, ** and * denote statistical significance at the 1, 5 and 10 per cent levels, respectively. |
7.3 Validity tests – selection into treatment
One of the requirements for valid RDD is that businesses cannot manipulate the forcing variable, revenue, to change their treatment status. If businesses can select into treatment, the assumption that businesses just above and below the cut-off are identical would be violated. Businesses with a higher ex ante propensity to invest would have a greater incentive to select into treatment.
In our case, manipulation of the forcing variable – revenue measured over a year before the announcement of the differential tax break – is improbable. Consistent with this, there is no graphical evidence of bunching of businesses just below the threshold (Figure 10). These graphical results are confirmed by the formal test proposed by Cattaneo, Jansson and Ma (2020).
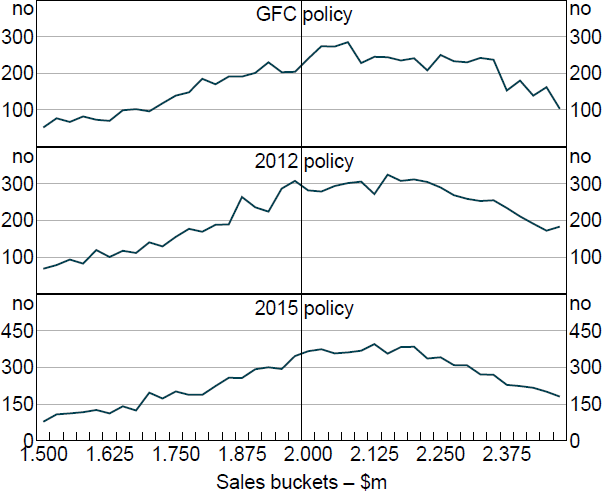
Notes: Sales buckets are $25,000 wide. Sales in year t – 2.
As discussed in Rodgers and Hambur (2018), one additional concern that is fairly unique to our RDD set-up due to the policy rules is that businesses might be manipulating their way out of the estimation sample. In particular, businesses with revenue above the cut-off in t – 2 and who forecast revenue just above the threshold in t – 1 or t, might have the incentive to manipulate their revenue in those years to qualify for the tax breaks. The firms would be manipulating their way our of the ‘control’ group (but not into the treated group). This could create a situation where our control group is comprised of firms with a lower propensity to invest than a typical firm, as those firms with a higher propensity to invest have manipulated out of the sample.
We test this possibility in two ways. One is to replace current investment in the regression with two-year lagged investment. This test of a pre-determined covariate should provide some sense of whether there are inherent differences between the treated and control groups.
Table 13 shows these results, focusing on the periods where the investment response was statistically significant. We find no evidence that there was a difference in the earlier investment propensity of firms either side of the threshold.
Sep 2009 | Dec 2015 | Mar 2016 | Jun 2016 | |
---|---|---|---|---|
Beta | 0.003 | −0.065 | −0.007 | 0.095 |
Robust p-value | (0.93) | (0.69) | (0.96) | (0.45) |
Bandwidth | 464,597 | 377,614 | 403,782 | 405,104 |
Effective observations | 2,763 | 3,134 | 3,181 | 3,506 |
Note: ***, ** and * denote statistical significance at the 1, 5 and 10 per cent levels, respectively. |
Still, we may be concerned that even if the earlier propensity to invest was the same, it may not be the case during the period of interest. As a further test, we rely on the assumption that businesses are only likely to be able to manipulate their revenue to a certain extent. Businesses with revenue just above the threshold may be able to manipulate their revenue down, but businesses with revenue well above the turnover limit could not.
As shown in Rodgers and Hambur (2018, Figure 6), there is some evidence that firms manipulated their sales in year t – 1 to qualify for the GFC policy. Specifically, there is a small spike in the number of firms with turnover just below $2 million in 2008/09, followed by a dip just after. It appears that most of this came from firms with ‘potential’ turnover between $2 million and $2.1 million.
To account for this we follow Rodgers and Hambur (2018) and re-estimate the model, only keeping firms with revenue in t – 1 or t that was more than 5 per cent above the threshold (equivalent to $100,000 for the $2 million threshold). The logic here is that for this subset of firms, it would have been harder for them to manipulate turnover to be below the threshold. As such, amongst the remaining firms we should be less worried that we are only left with those that didn't have the incentive to manipulate into the policy (and out of our sample) using turnover in t or t – 1, as all remaining firms would have had moderate difficulty in doing so.
Table 14 shows the results, again focusing on the statistically significant periods. The results are quite similar, suggesting that manipulation is not driving the findings.
Sep 2008 | Dec 2015 | Mar 2016 | Jun 2016 | |
---|---|---|---|---|
All firms | ||||
Beta | −0.364* | −0.073 | −0.500** | −0.197 |
Robust p-value | (0.05) | (0.79) | (0.02) | (0.35) |
Bandwidth | 306,648 | 426,061 | 298,216 | 522,424 |
Effective observations | 1,584 | 2,763 | 1,969 | 3,014 |
Unincorporated | ||||
Beta | −0.528* | −0.542** | −0.728** | −0.607** |
Robust p-value | (0.06) | (0.04) | (0.02) | (0.03) |
Bandwidth | 315,977 | 335,454 | 311,429 | 386,911 |
Effective observations | 705 | 1,198 | 1,010 | 1,208 |
Note: ***, ** and * denote statistical significance at the 1, 5 and 10 per cent levels, respectively. |
7.4 Repeated treatment
As Australia has implemented a number of different ITB policies over recent years, one concern could be interaction between the various policies and overlap of the time periods covered by the policies. For example, firms that took up the GFC policy may have had less incentive to invest using the 2012 policy if they had already made all the investment they desired.
We do see some evidence of overlap. For example, around half of the treated group in our GFC regressions were in our treated group for the 2012 policy. However, both our results and Rodgers and Hambur (2018) provide very limited evidence of bring forward over the one to two years following the GFC policy. We think the likelihood that this is driving our results for the 2012 policy is very low.