RDP 1999-01: The Phillips Curve in Australia Appendix D: Technical Issues Involved in Estimating the Kalman Filter
January 1999
- Download the Paper 492KB
In this appendix we discuss some of the technical issues involved in estimating the NAIRU as a time-varying parameter using the Kalman filter.
Recall that we are treating the NAIRU as a unit root process of the form:
where vt is assumed to be , and we begin with a
price Phillips curve equation which corresponds to Debelle and Vickery's (1997) preferred
functional form:
where εt is assumed to be N(0,r).
Expanding Equation (D2) gives the following estimating equation:
which can be written more generally as:
where and
.
When α is known to be constant we can define a state variable and then
Equations (D1) and (D2) constitute a state space form (SSF):
where and
var(εt) = r.
Because of the ability to represent the equations as a SSF, most researchers using this approach to account for a time-varying NAIRU have estimated the state zt, conditional upon the past history of yt (and contemporaneous xt), with the prediction segment of the Kalman filter. This produces Et−1(zt). To do that it is necessary to initiate the recursion with an initial value for the state z1|0 and its variance z1|0. In most instances z1|0 is treated as a parameter and z1|0 is set to zero (Pagan 1980). Subsequently, conditional upon the values of r, σν and z1|0 one can derive the innovations ηt = yt − Et−1(yt) and their conditional variances ht, whereupon the log likelihood will be:
Maximising Equation (D7) then provides a way of estimating any unknown parameters.[30]
One of the most important parameters to be estimated is q. Apart from fixing it, as
Debelle and Vickery do, there have been other suggestions in the literature. Laxton et al (1998, p. 29) report setting q = r when using annual
data, because the resulting estimates of the NAIRU were not excessively volatile. This suggests
that one needs to study the impact of varying q more carefully in order to understand
exactly how the estimates of the NAIRU are made. To do so, it is best to concentrate
α and z1|0 out of the likelihood, leaving only r and
q as parameters. The key to doing this is to examine the Kalman prediction equations.
One can show that the prediction of the state using past
information is:
where Kt = Pt|t−1Ht(HtPt|t−1Ht + r)−1 is the gain of the Kalman filter, Pt|t−1 is the variance of zt conditional on past information (a quantity that is computed by the Kalman filter algorithm and depends only on r and q) and bt = 1 − HtKt. Recursively solving Equation (D8) gives:
where
are also generated recursively for j = 1…n using initial conditions ϕ1 = 1, sy1 = 0 and sx1 = 0.
With this information, the innovations ηt = yt − Et−1(yt) can be written as:
Since the maximum likelihood estimates of α and z1|0 maximise Equation (D7) it is clear that, for a given r and q, they can be estimated by performing a weighted least squares regression of yt − Htsyt against xt − Htsxt and Htϕt, where the weights are the inverse of the standard deviation of the innovations (their estimated variance ht depends only on r and q). Thus we can easily concentrate α and z1|0 out of the log likelihood, leaving only r and q.
The result just described is useful for producing graphical representations of the sensitivity
of the log likelihood to variations in q as well as helping us to understand how the
NAIRU is estimated. Equation (D8) shows that the estimate of the state zt is
a weighted average of all past values of with weights that decline
like bt but which also vary with Kt. If a linear version
of the Phillips curve had been used, Ht would not vary with time, and one
could have used the asymptotic version of the Kalman filter; this results in a constant gain
K and, hence, constant weights b. In that case one would simply be doing a
geometrically weighted average of the residuals
when forming the
estimated zt. To derive an estimate of the NAIRU from zt
one also needs to divide by the estimate of γ. This analysis points to the fact
that estimates of the NAIRU made using this methodology will depend upon the ability of
xt to predict the change in inflation yt, and not just
on r and q. Moreover, the NAIRU is very sensitive to the estimate made of
γ. In this respect, the problems of getting a precise estimate of the
time-varying NAIRU are the same as with the constant-NAIRU version in an equation such as
Equation (9), where the intercept in the regression is divided by γ. All that
happens now is that the numerator is replaced by a weighted average of some residuals rather
than an estimated intercept. The explicit formula in Equation (D8) could be useful for those
papers looking at monetary policy in the face of a changing NAIRU, for example, Wieland (1998), where the authorities need to solve a signal
extraction problem when devising an optimal policy.
An important message from this analysis is that close attention needs to be paid to devising a suitable specification for the equation linking yt and xt. For this reason Equation (9), from Section 2, seems a suitable source of extra regressors in xt over and above those used by Debelle and Vickery (1997). Such an extension produces the specification for the price Phillips Curve Equation (13), which we estimate in Section 3. Parameter estimates (and the associated t-ratios) for that equation are presented in the text. Here we examine the sensitivity of the log likelihood to variations in r and q using the technique just described. Figure D1 shows a three dimensional plot of the concentrated log-likelihood function against values of r and q. This figure shows that we could accept a wide range of hypotheses about values of q, which means that, for given r, our model is unable to provide a precise estimate of the variation in the NAIRU.
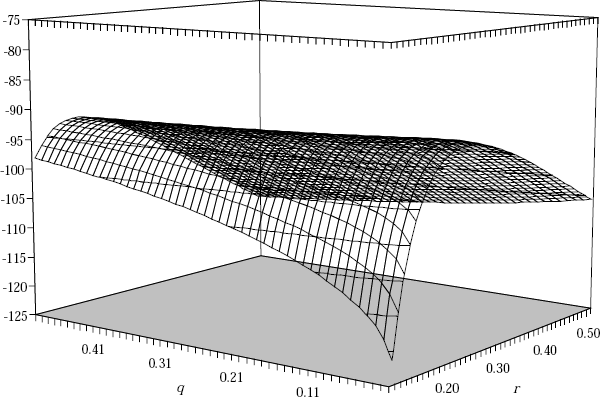
Similarly, for our preferred unit labour cost Phillips curve Equation (14), Figure D2 shows the concentrated log-likelihood function. Here again we see that we could accept a wide range of hypotheses about values of q.
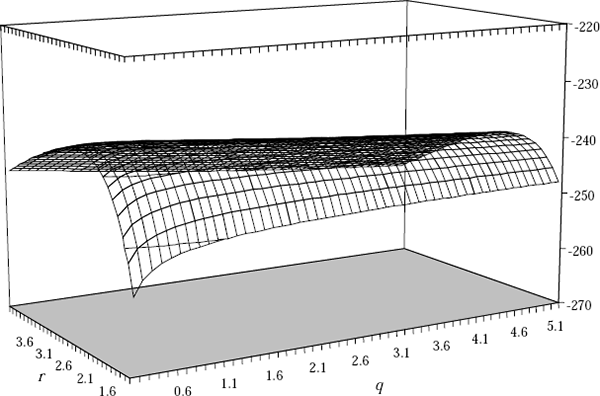
Footnote
Debelle and Vickery (1997) do not proceed in this way. Instead, they set r = 1, pre-specify z1|0 and P1|0, and then seem to determine q by how well the resulting estimated path of the NAIRU accords with their priors. Thus, the role of data in determining their NAIRU estimates is more limited than it need be. [30]